PERTAD: Towards Pseudo Verification for Anomaly Detection in Partially Labeled Graphs.
International Joint Conference on Web and Big Data(2023)
摘要
The graph-based anomaly detection task aims to identify nodes with patterns that deviate from those of the majority nodes in a large graph, where only a limited subset of nodes is annotated. However, inadequate supervised knowledge and uncertainty of anomalous structure restrict the performance of detection. In this paper, we propose PERTAD ( P seudo V ER ifica T ion for A nomaly D etection), a novel semi-supervised learning method for detecting anomalies in partially labeled graphs. Specifically, we first propose a self-verification framework that comprises a target network (T-model) and a verification network (V-model). The framework employs pseudo-labeled graphs to transfer the knowledge learned by T-model to V-model, and then corrects T-model with the performance error between the two networks on the labeled set. Furthermore, we introduce a layer-level aggregation mechanism for node representation in deep GNNs to address the uncertainty of anomalous structures. The proposed mechanism re-aggregates neighborhood information across layers, aiming to preserve low-order neighborhood characteristics and alleviate the over-smoothing effect. Extensive experiments on real-world graph-based anomaly detection tasks demonstrate that PERTAD significantly outperforms state-of-the-art baselines.
更多查看译文
AI 理解论文
溯源树
样例
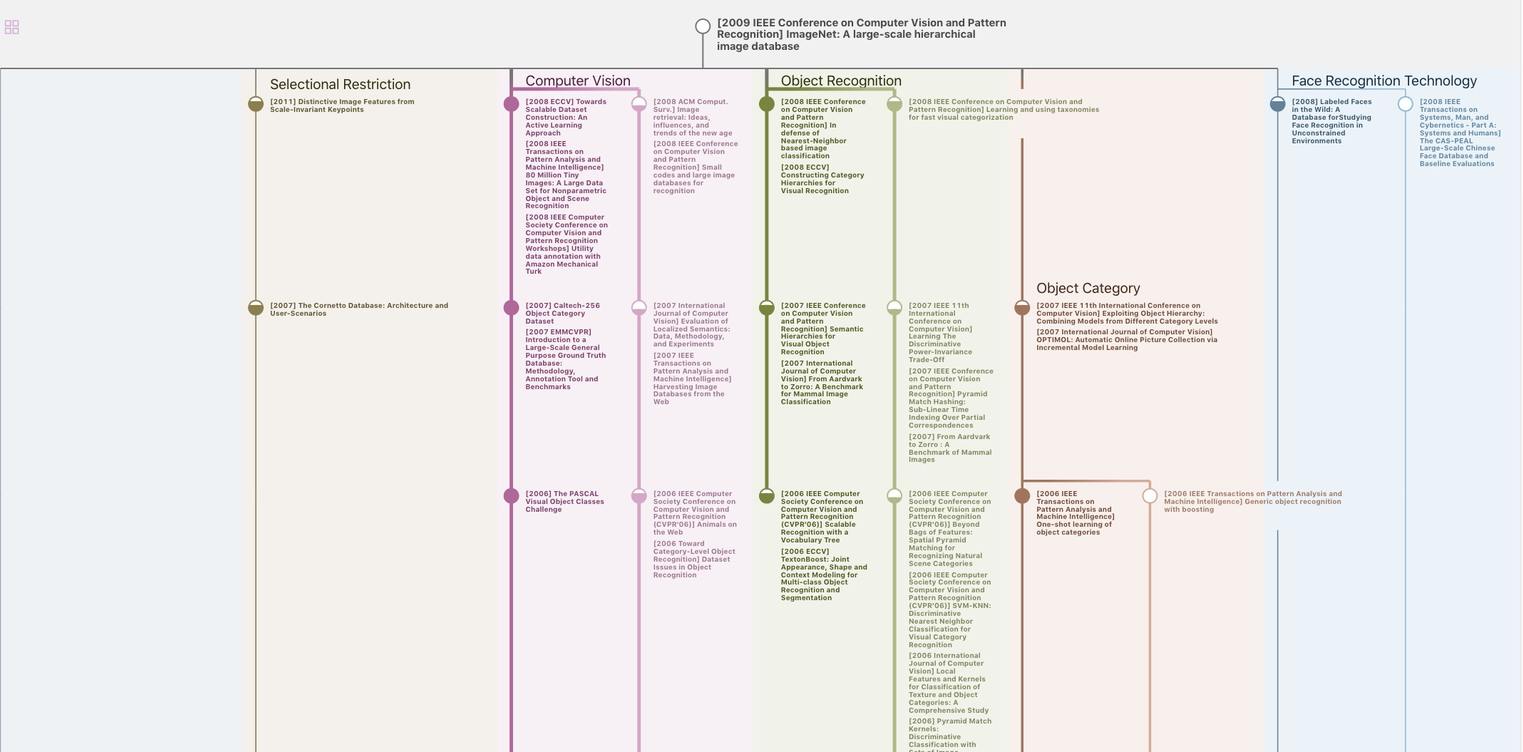
生成溯源树,研究论文发展脉络
Chat Paper
正在生成论文摘要