Exploring Collective Theory of Mind on Pedestrian Behavioral Intentions.
ACM Conference on Human Factors in Computing Systems(2024)
摘要
While crowdsourcing is commonly used for objective labeling, eliciting subjective annotations, like estimating mental states or perception of other’s intention, remains challenging. This study investigates crowdsourcing’s potential to predict pedestrian behavioral intentions. We recruited 120 participants to predict pedestrian intentions at different prediction horizons in 24 diverse videos. Our findings revealed that the status-quo bias significantly impacts intention estimation. Specifically, when asked what status the pedestrian will be, predictions inclined towards current state’s continuation over transition, with an overall accuracy of 53% at one-second prediction length on a balanced dataset. Rephrasing the annotation question mitigates this bias and improved the estimation accuracy to 79% for one-second ahead predictions, though accuracy drops with longer horizons and is affected by pedestrian actions and contextual information. Overall, this study provides insights into the factors affecting collective estimation of pedestrian intentions and aims to improve crowdsourcing cognitive labels for training better AV-pedestrian interaction algorithms.
更多查看译文
AI 理解论文
溯源树
样例
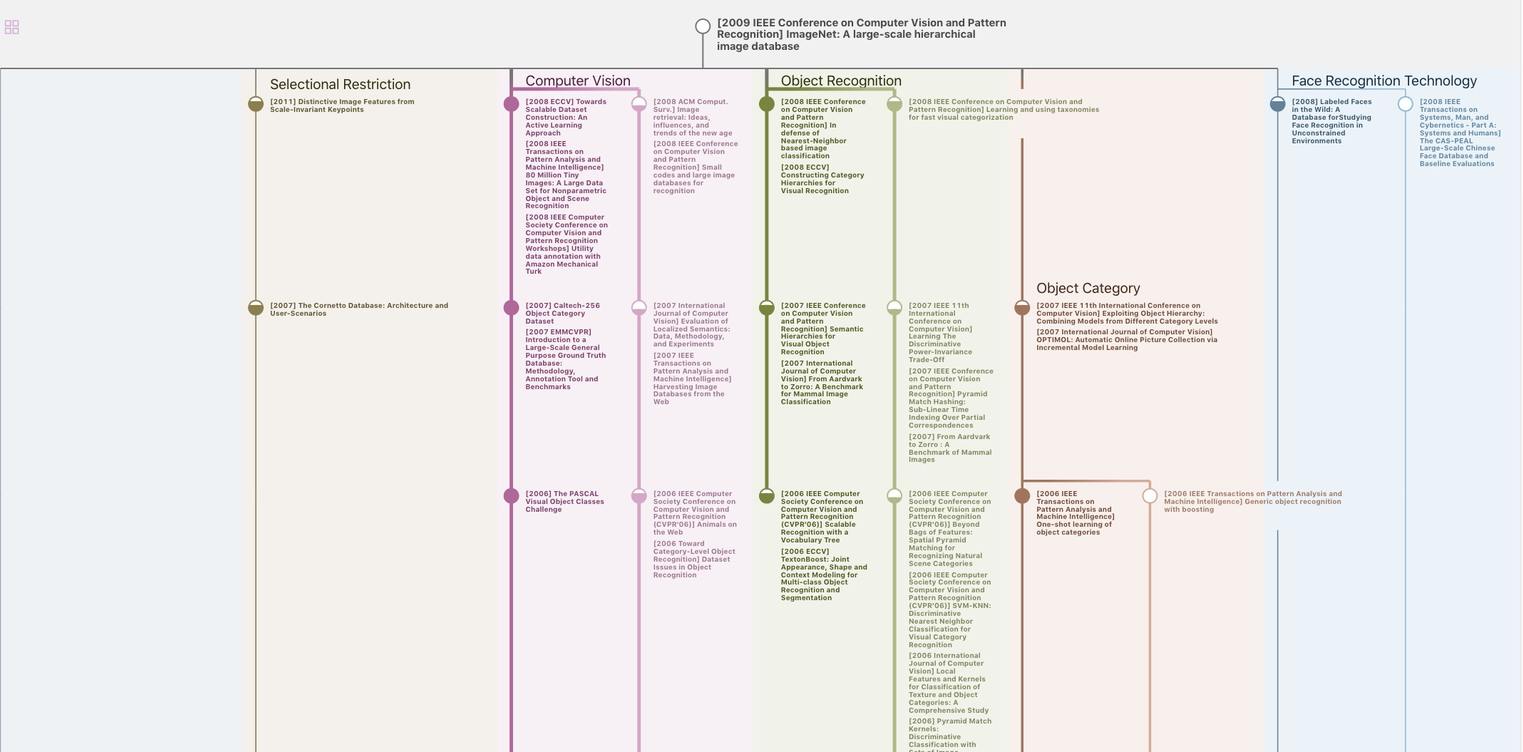
生成溯源树,研究论文发展脉络
Chat Paper
正在生成论文摘要