Next Generation Seismic Source Detection by Computer Vision: Untangling the Complexity of the 2016 Kaikōura Earthquake Sequence
Journal of Geophysical Research: Solid Earth(2024)
摘要
AbstractSeismic source locations are fundamental to many fields of Earth and planetary sciences, such as seismology, volcanology and tectonics. However, seismic source detection and location are challenging when events cluster closely in space and time with signals tangling together at observing stations, such as they often do in major aftershock sequences. Though emerging algorithms and artificial intelligence (AI) models have made processing high volumes of seismic data easier, their performance is still limited, especially for complex aftershock sequences. In this study, we propose a novel approach that utilizes three‐dimensional image segmentation—a computer vision technique—to detect and locate seismic sources, and develop this into a complete workflow, Source Untangler Guided by Artificial intelligence image Recognition (SUGAR). In our synthetic and real data tests, SUGAR can handle complex, energetic earthquake sequences in near real time better than skillful analysts and other AI and non‐AI based algorithms. We apply SUGAR to the 2016 Kaikōura, New Zealand sequence and obtain five times more events than the analyst‐based GeoNet catalog. The improved aftershock distribution illuminates a continuous fault system with extensive fracture zones beneath the segmented, discontinuous surface ruptures. Our method has broader applicability to non‐earthquake sources and other time series image data sets.
更多查看译文
AI 理解论文
溯源树
样例
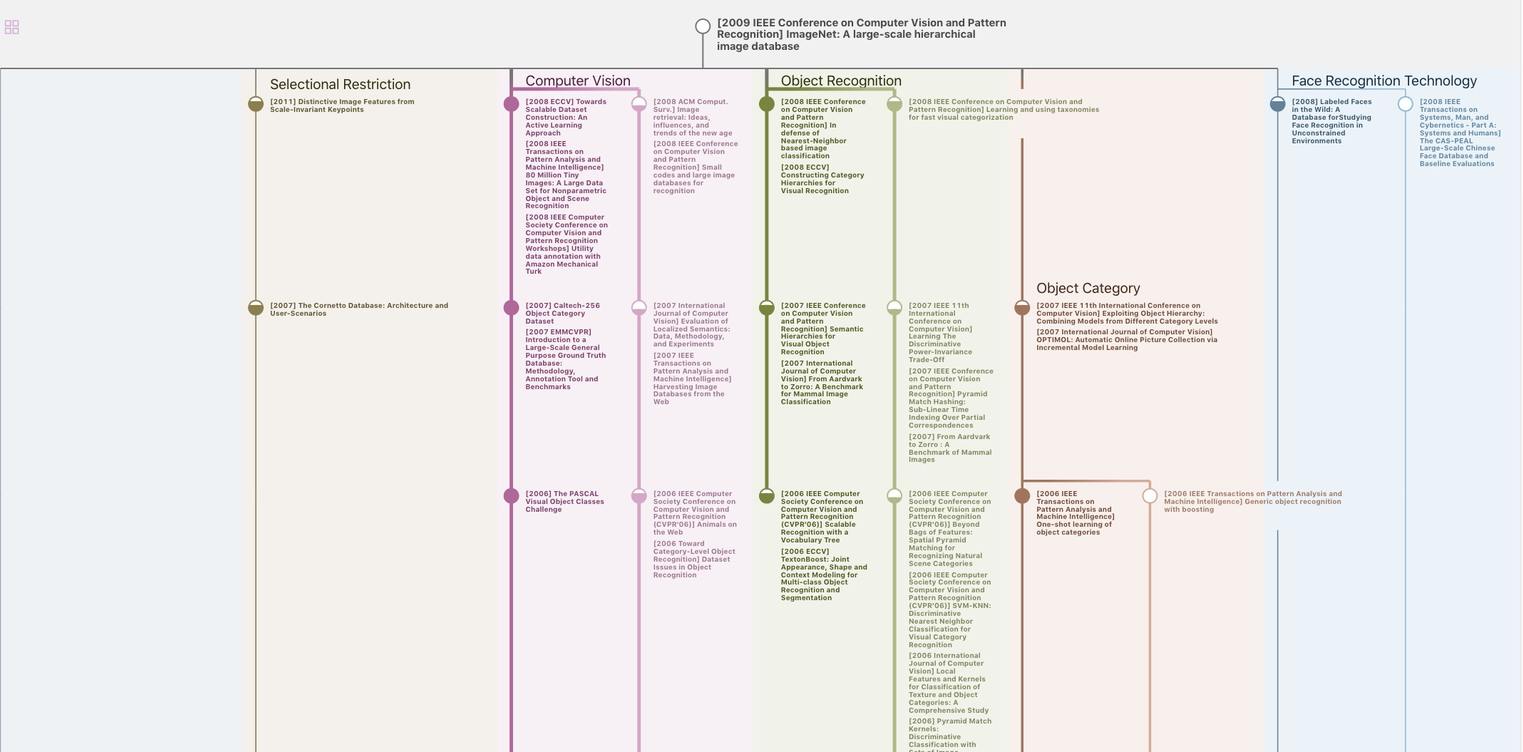
生成溯源树,研究论文发展脉络
Chat Paper
正在生成论文摘要