A Transformer-Guided Cross-Modality Adaptive Feature Fusion Framework for Esophageal Gross Tumor Volume Segmentation
Computer Methods and Programs in Biomedicine(2024)
摘要
Background and Objective
Accurate segmentation of esophageal gross tumor volume (GTV) indirectly enhances the efficacy of radiotherapy for patients with esophagus cancer. In this domain, learning-based methods have been employed to fuse cross-modality positron emission tomography (PET) and computed tomography (CT) images, aiming to improve segmentation accuracy. This fusion is essential as it combines functional metabolic information from PET with anatomical information from CT, providing complementary information. While the existing three-dimensional (3D) segmentation method has achieved state-of-the-art (SOTA) performance, it typically relies on pure-convolution architectures, limiting its ability to capture long-range spatial dependencies due to convolution's confinement to a local receptive field. To address this limitation and further enhance esophageal GTV segmentation performance, this work proposes a transformer-guided cross-modality adaptive feature fusion network, referred to as TransAttPSNN, which is based on cross-modality PET/CT scans.
Methods
Specifically, we establish an attention progressive semantically-nested network (AttPSNN) by incorporating the convolutional attention mechanism into the progressive semantically-nested network (PSNN). Subsequently, we devise a plug-and-play transformer-guided cross-modality adaptive feature fusion model, which is inserted between the multi-scale feature counterparts of a two-stream AttPSNN backbone (one for the PET modality flow and another for the CT modality flow), resulting in the proposed TransAttPSNN architecture.
Results
Through extensive four-fold cross-validation experiments on the clinical PET/CT cohort. The proposed approach acquires a Dice similarity coefficient (DSC) of 0.76 ± 0.13, a Hausdorff distance (HD) of 9.38 ± 8.76 mm, and a Mean surface distance (MSD) of 1.13 ± 0.94 mm, outperforming the SOTA competing methods. The qualitative results show a satisfying consistency with the lesion areas.
Conclusions
The devised transformer-guided cross-modality adaptive feature fusion module integrates the strengths of PET and CT, effectively enhancing the segmentation performance of esophageal GTV. The proposed TransAttPSNN has further advanced the research of esophageal GTV segmentation.
更多查看译文
关键词
Esophageal gross tumor volume,segmentation,transformer,feature fusion,PET/CT
AI 理解论文
溯源树
样例
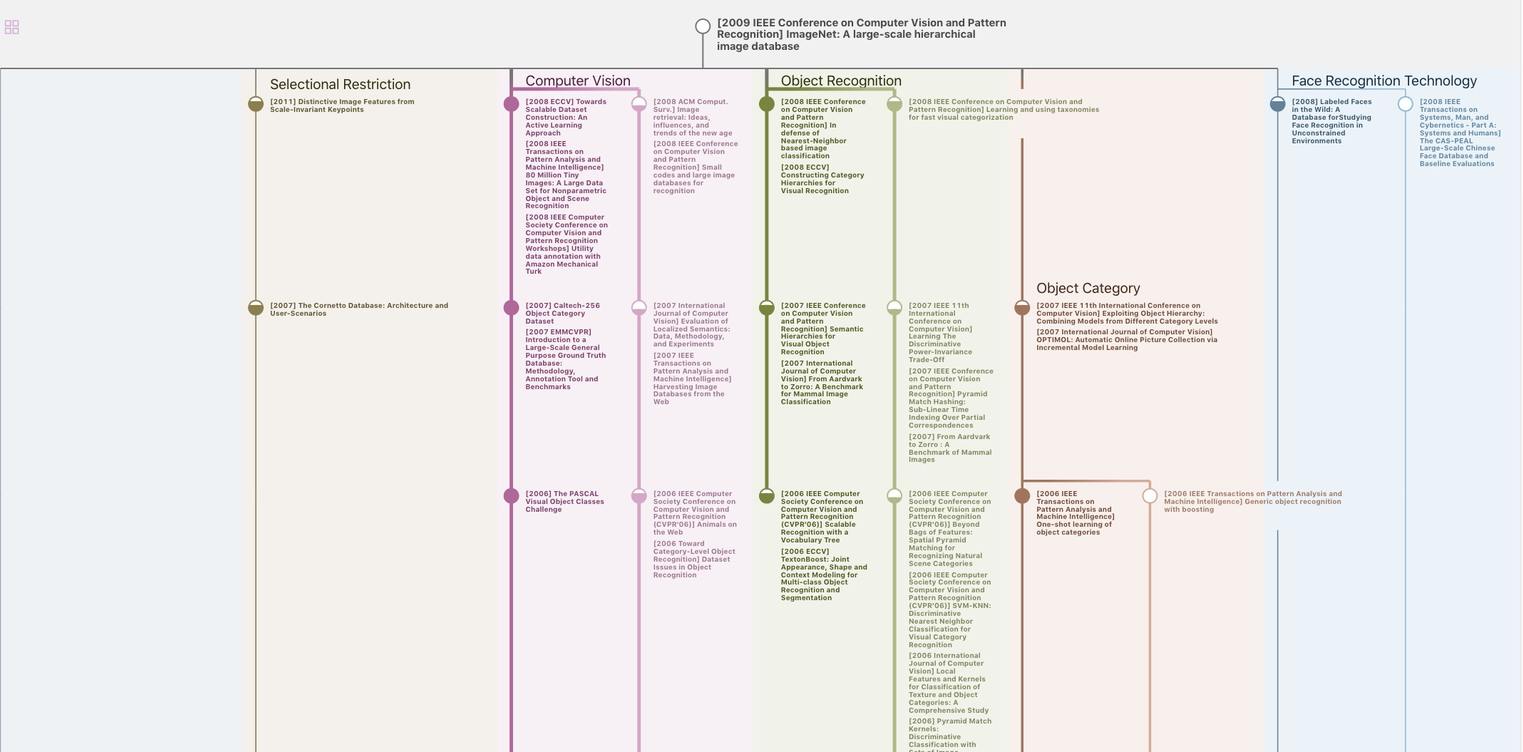
生成溯源树,研究论文发展脉络
Chat Paper
正在生成论文摘要