Adaptive fast nonlinear blind deconvolution based on nonuniform particle swarm optimization for the rolling bearing fault diagnosis
Measurement(2024)
摘要
Although superior fault extraction performance is possessed by fast nonlinear blind deconvolution (FNBD) through the introduction of penalty terms and nonlinear features, its wide application is limited by the complexity and diversity of parameter selection. The fault extraction performance of FNBD is impacted by the contingency of parameter selection. To reduce the prior experience dependence of FNBD and improve the performance of fault extraction, an end-to-end adaptive fast nonlinear blind deconvolution (AFNBD) is proposed. First, the proposed nonuniform particle swarm optimization (NPSO) is employed to assign parameters to be optimized for each particle to construct its Hankel matrix, nonlinear features and objective functions. Secondly, Gaussian fitting is applied to modify the filters. Then, NPSO is utilized to update relevant parameters and the corresponding filter of each particle to explore the optimum filter. Finally, simulations and experiments verify the effectiveness of AFNBD and the results indicate that AFNBD is a powerful tool for incipient fault feature extraction.
更多查看译文
关键词
Unsupervised learning,Fast nonlinear blind deconvolution,Particle swarm optimization,Bearing,Fault diagnosis
AI 理解论文
溯源树
样例
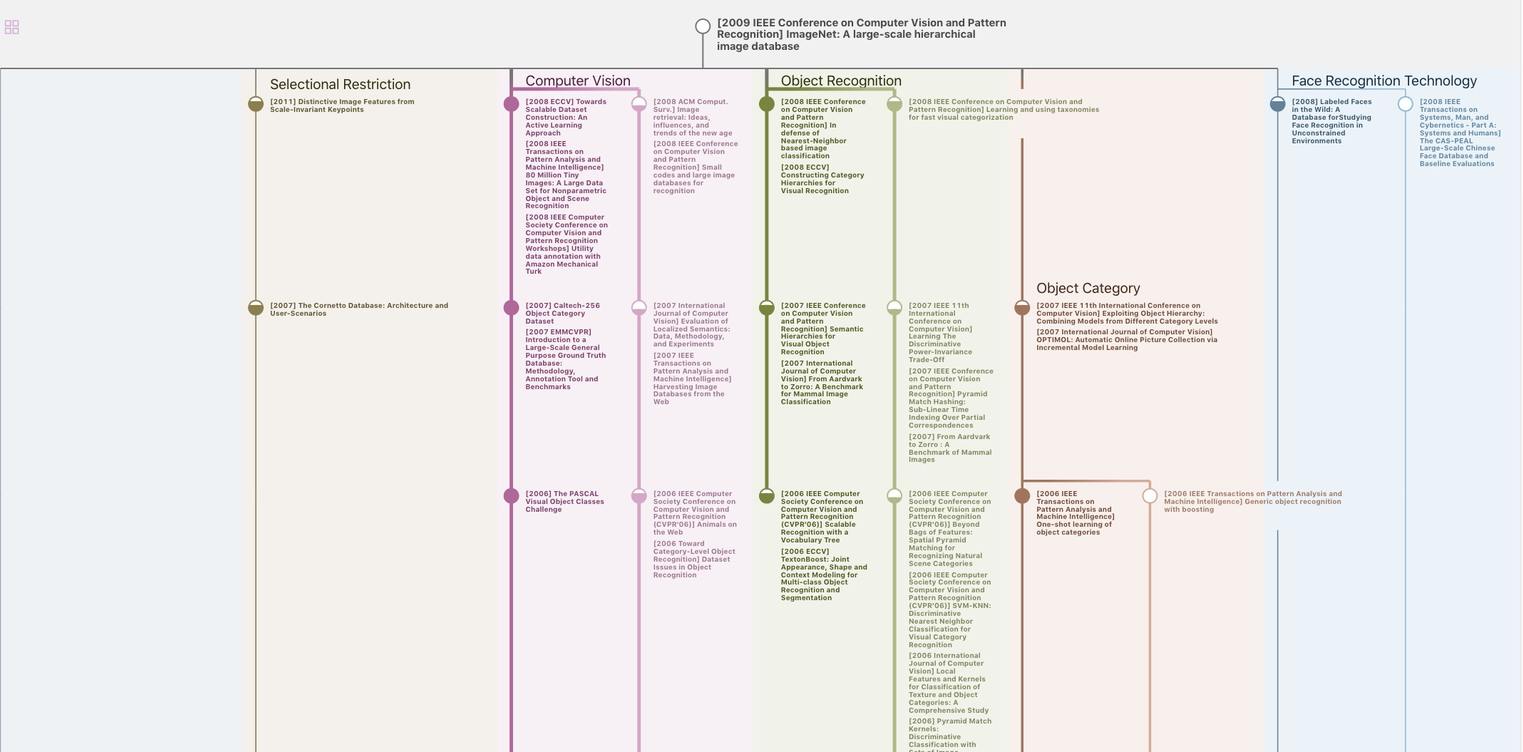
生成溯源树,研究论文发展脉络
Chat Paper
正在生成论文摘要