Developing Vis–NIR libraries to predict cation exchange capacity (CEC) and pH in Australian sugarcane soil
Computers and Electronics in Agriculture(2024)
摘要
In sugarcane growing areas of Queensland Australia, management of soil condition requires information about soil chemical properties (i.e., cation exchange capacity [CEC], and pH), because they are used to make fertiliser recommendations. While laboratory analysis is cost prohibitive the development of visible near–infrared (Vis–NIR) spectroscopy libraries might be useful. The aims of this study was to compare: i) linear (i.e., Partial least squares regression [PLSR]) and machine learning (i.e., Cubist, Random Forest [RF] and Support vector machine [SVM]) algorithms in terms of their calibration strength (i.e., coefficient of determination (R2); ii) depth-specific (i.e., topsoil [0 – 0.3 m], subsurface [0.3 – 0.6 m], subsoil [0.6 – 0.9 m] and deep subsoil [0.9 – 1.2 m]) and multi-depth libraries; iii) prediction R2, agreement (Lin’s concordance correlation coefficient [LCCC]) and accuracy (ratio of performance to interquartile [RPIQ]); and iv) minimum number of calibration sample locations (i.e., n = 400, 350, …, 50). For depth-specific calibration for CEC, strong calibration R2 was achieved for the subsurface (i.e., RF [0.95], Cubist [0.93], PLSR [0.88] and SVM [0.81]), and for pH, the largest R2 was for deep subsoil (i.e., RF [0.91], followed by PLSR [0.80], Cubist [0.79] and SVM [0.56]), with subsurface, and deep subsoil similar, with topsoil R2 smaller. In terms of model prediction of depth-specific, Cubist was superior to PLSR, RF and SVM for CEC, and PLSR was the best for pH. For example, agreement for topsoil CEC was substantial for Cubist (0.80) and PLSR (0.80), but moderate for RF (0.69) and poor for SVM (0.59). For subsurface (0.9), subsoil (0.91) and deep subsoil (0.92), the agreements were perfect for CEC using Cubist. In terms of multi-depth calibration for CEC it was strong for RF (R2 = 0.94), Cubist (0.92), PLSR (0.81) and SVM (0.72), but for prediction agreement, Cubist (LCCC = 0.92) was perfect, with PLSR (0.85), RF (0.84) and SVM (0.83) substantial. This was also the case for pH (0.84) substantial. However, there are efficiencies in developing a multi-depth calibration. Moreover, the minimum number of calibration sample sites required was 300 (i.e., 1,200 samples) (1.78 sites/ha). The results have implications for using a Vis–NIR library to replace traditional soil laboratory analysis and for fertiliser recommendations for sugarcane soil.
更多查看译文
关键词
Visible and near – infrared (Vis–NIR) spectroscopy,Multi-depth libraries,Depth-specific libraries,Machine learning models,Sample size,Duplex Soil
AI 理解论文
溯源树
样例
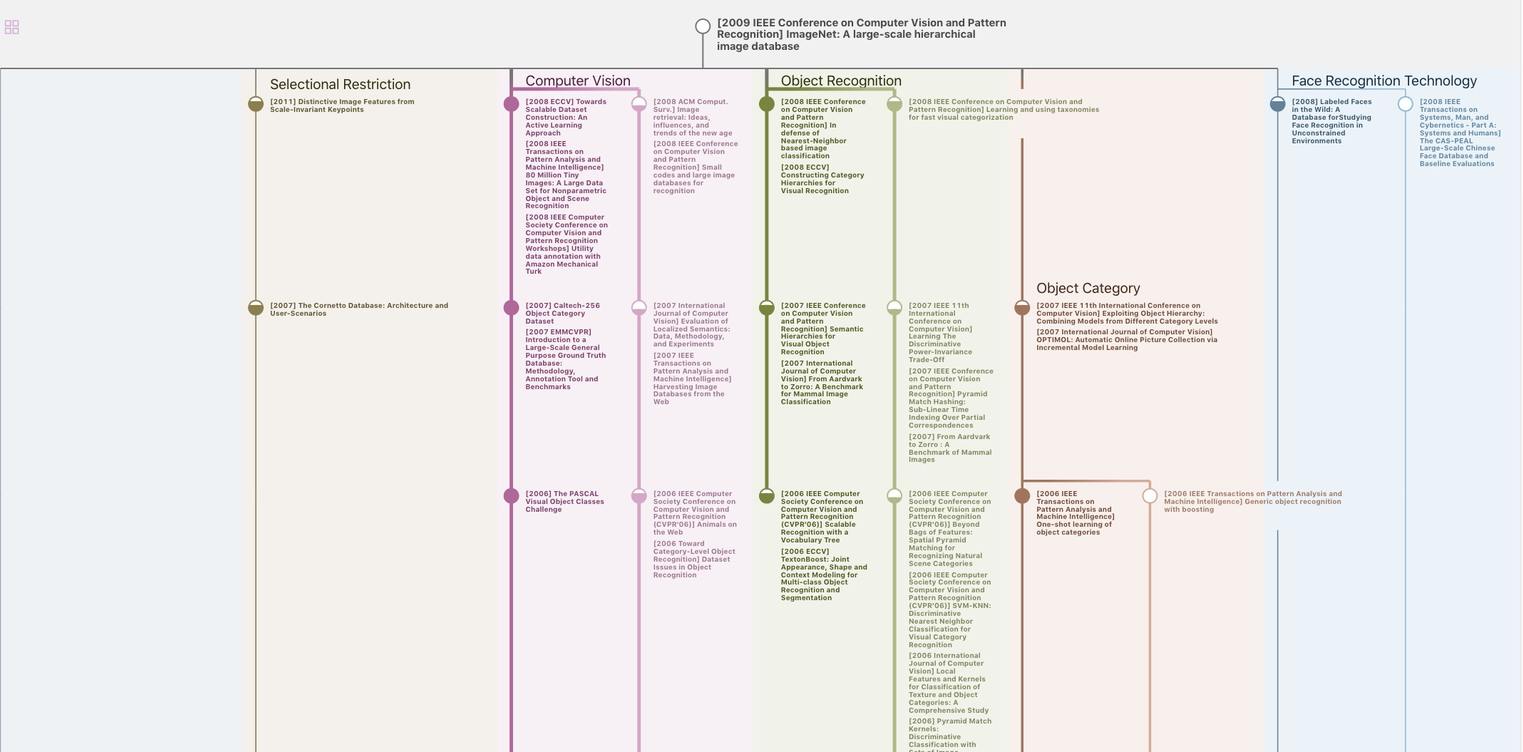
生成溯源树,研究论文发展脉络
Chat Paper
正在生成论文摘要