Non-intrusive thermal load disaggregation and forecasting for effective HVAC systems
APPLIED ENERGY(2024)
摘要
Non -Intrusive Thermal Load Monitoring (NITLM) tracks the sub -loads generated by each heat source (e.g. occupants, equipment, solar radiation etc.) from the total thermal load and indirectly provides a room's thermal properties without additional sensors. Since sub -loads can improve the efficiency of HVAC systems, NITLM is a very attractive technology for building energy management. NITLM has traditionally focused on analyzing past and present sub -loads. However, by forecasting future sub -loads, HVAC systems will be able to schedule operations that take into account the thermal properties of future rooms. This work focuses on a new NITLM framework that forecasts future sub -loads based on the current and past total thermal loads. In experiments, we selected occupant loads that are closely connected to HVAC systems and performed subload forecasting using two types of approaches. One is a two-step approach that separately performs them in turn. This approach use separately trained model for disaggregation and forecasting, this allow us to finetuning the hyper -parameter for dedicate model. Moreover, the two-step approach can take into account the different properties and difficulties of each inference, resulting in smaller errors in sub -load forecasting. The other is an integrated approach. This approach combines load disaggregation and forecasting into a single estimation process, eliminating error propagation and reducing overall error in sub -load forecasting. Moreover, this approach utilizes the Adaptive Moment Estimation (Adam) algorithm for effective parameter optimization, enabling complex training and improving the accuracy of sub -load forecasting. We conducted evaluations of thermal load disaggregation and forecasting across a range of realistic building scenarios. The findings indicate that the integrated approach predicts sub -loads with a MAE that is up to 34.9% lower than that of the two-step approach. Additionally, it identifies occupants presence with an 18.5% higher F -score. This demonstrates its enhanced suitability for accurately predicting sub -loads and estimating future occupancy schedules.
更多查看译文
关键词
Non-intrusive thermal load monitoring,Thermal load disaggregation,Neural network,Time series forecasting
AI 理解论文
溯源树
样例
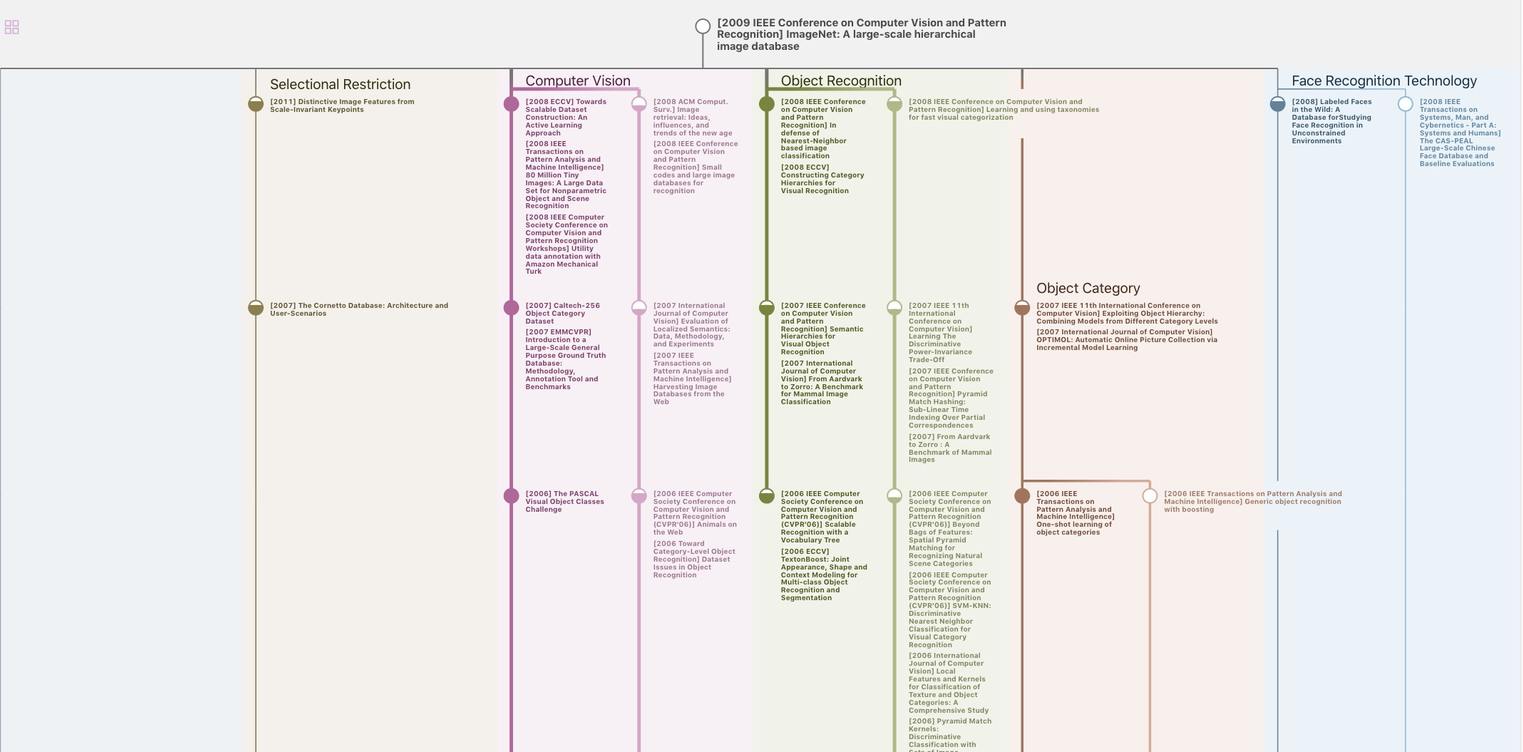
生成溯源树,研究论文发展脉络
Chat Paper
正在生成论文摘要