A Grouper Fish Mortality Prediction Model Using Transfer Learning Approach
2024 IEEE International Conference on Artificial Intelligence and Mechatronics Systems (AIMS)(2024)
摘要
The grouper species holds considerable potential for aquaculture due to its high value. The significant challenge of high mortality rates among groupers is addressed by utilizing buoy devices for water quality monitoring. Technical issues with buoys frequently result in data loss, impacting the dataset. Machine learning techniques, particularly transfer learning, are being explored as a potential solution to address the challenges posed by limited datasets. The objective of this study is to create a fish mortality prediction model by utilizing transfer learning from the Habs event model in Lampung in situations where data is limited. Observational discrepancies exist in the distribution patterns of fish mortality across different study parameters. This observation implies that certain parameters, such as current, salinity, conductivity, chlorophyll, and turbidity, have a notable impact on the risk of fish mortality. The transfer learning model with fine-tuning has achieved excellent results in model evaluation metrics, such as area under curve (AUC) (94.545%) and mean squared error (MSE) (0.1108). The proposed model demonstrates superior performance compared to deep learning without transfer learning and transfer learning model without fine-tuning.
更多查看译文
关键词
deep learning,fine-tuning,fish mortality,prediction model,transfer learning
AI 理解论文
溯源树
样例
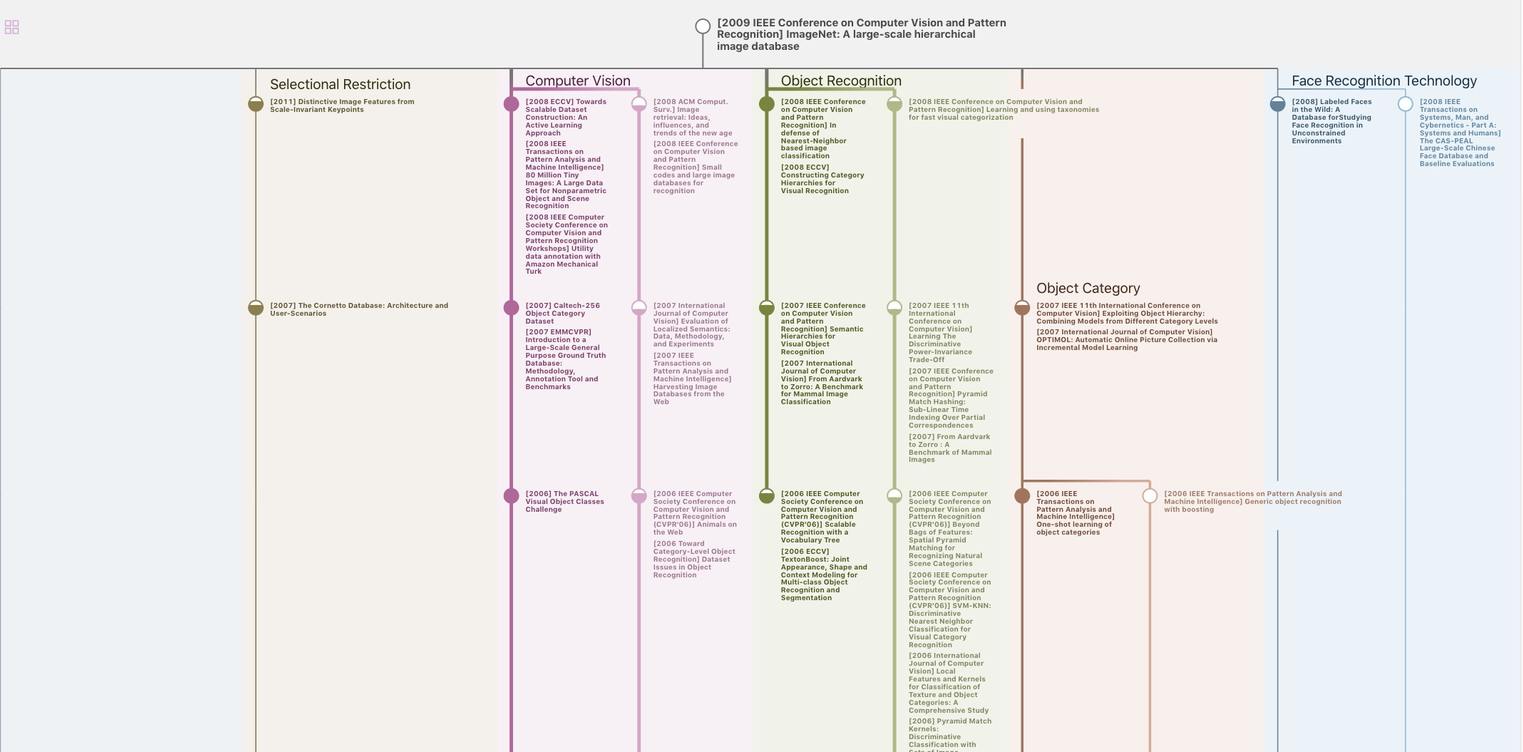
生成溯源树,研究论文发展脉络
Chat Paper
正在生成论文摘要