Advancing zero-shot semantic segmentation through attribute correlations
Neurocomputing(2024)
摘要
Zero-shot semantic segmentation aims to segment novel classes that have not been encountered during the training phase. Existing methods leverage available text features obtained from pretrained language models to produce semantic segmentation results for both base and novel classes. However, the text-based feature-producing paradigm only provides insufficient class correlations and limits the full exploitation of image features from base classes. Besides, there exists a non-negligible domain gap between the text and image domains, resulting in severe feature bias during feature production. Different from existing methods, we advance the zero-shot semantic segmentation through attribute correlations. Specifically, we introduce a set of shared-attribute labels, of which the design fully considers the structural relations between attributes and classes, to provide rational and sufficient attribute-class correlations. Besides, due to the minor intra-class variations of shared attributes, the text features are more easily mapped to image features, thereby alleviating the domain gap issue. Furthermore, we propose a hierarchical semantic segmentation framework incorporating an attribute prompt tuning method. This approach is designed to enhance the model’s adaptation to the attribute segmentation task and effectively leverage attribute features to produce better semantic segmentation results. Correspondingly, we construct a Visual Hierarchical Semantic Classes (VHSC) benchmark, meticulously annotating shared-attributes at the pixel level to conduct the experiments. Extensive experiments on the VHSC benchmark showcase the superior performance of our method compared to existing zero-shot semantic segmentation methods, achieving mIoU of 73.0% and FBIoU of 87.5%. The VHSC benchmark and our code will be released to the community.
更多查看译文
关键词
Zero-shot learning,Image segmentation,Attribute learning
AI 理解论文
溯源树
样例
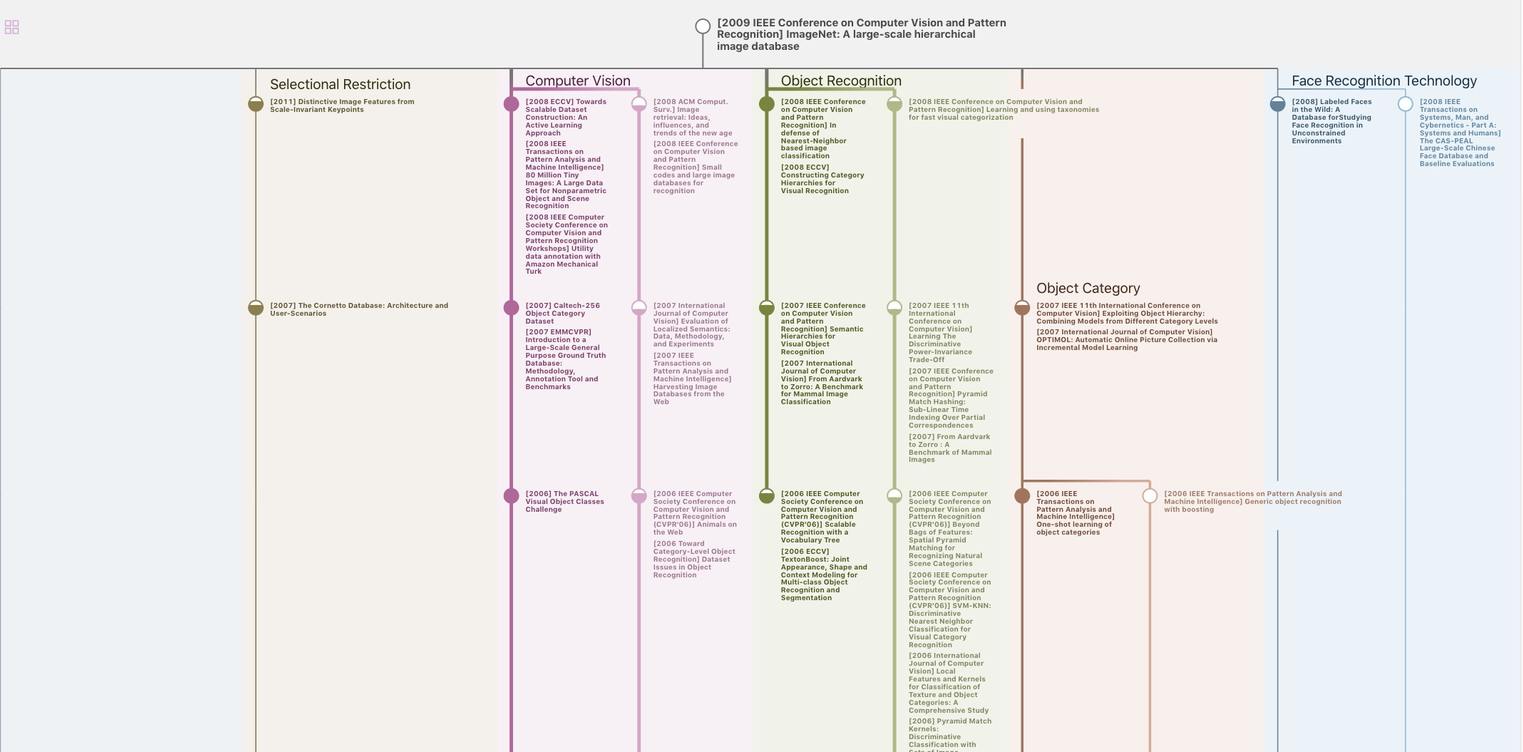
生成溯源树,研究论文发展脉络
Chat Paper
正在生成论文摘要