Model construction and multi-objective performance optimization of a biodiesel-diesel dual-fuel engine based on CNN-GRU
Energy(2024)
Abstract
To tackle the challenges of low efficiency and excessive NOx emissions in biodiesel-diesel dual-fuel engines, this paper presents an optimization method using the Pareto-multiple objective snake optimizer (Pareto-MOSO) to drive a convolutional neural network-gated recurrent unit (CNN-GRU). In the Pareto-MOSO, brake specific fuel consumption (BSFC), NOx, Soot, and CO are optimized by changing the engine control parameters, including injection timing (Tinject), engine speed (n), biodiesel blending ratio, torque (Ttorque), exhaust gas recirculation (EGR rate), intake pressure (Pin), and rail pressure (Prail). The experiment generates training data for CNN-GRU and verifies the accuracy of the Pareto-MOSO optimization results. The findings suggest that the optimized engine exhibits a balanced correlation between economy and emissions under propulsion characteristics. Furthermore, the NOx emissions are all in accordance with the IMO Tier III emission regulations. Under the rated condition, scheme 2 demonstrates a significant reduction of 76.57% in NOx emissions. However, this optimization has resulted in a 1.63% increase in BSFC compared to its pre-optimized state. Therefore, the adoption of suitable control strategies proves advantageous in addressing the trade-off between economy and emissions of engines.
MoreTranslated text
Key words
Dual fuel,CNN-GRU,Pareto-MOSO,Multi-objective optimization
AI Read Science
Must-Reading Tree
Example
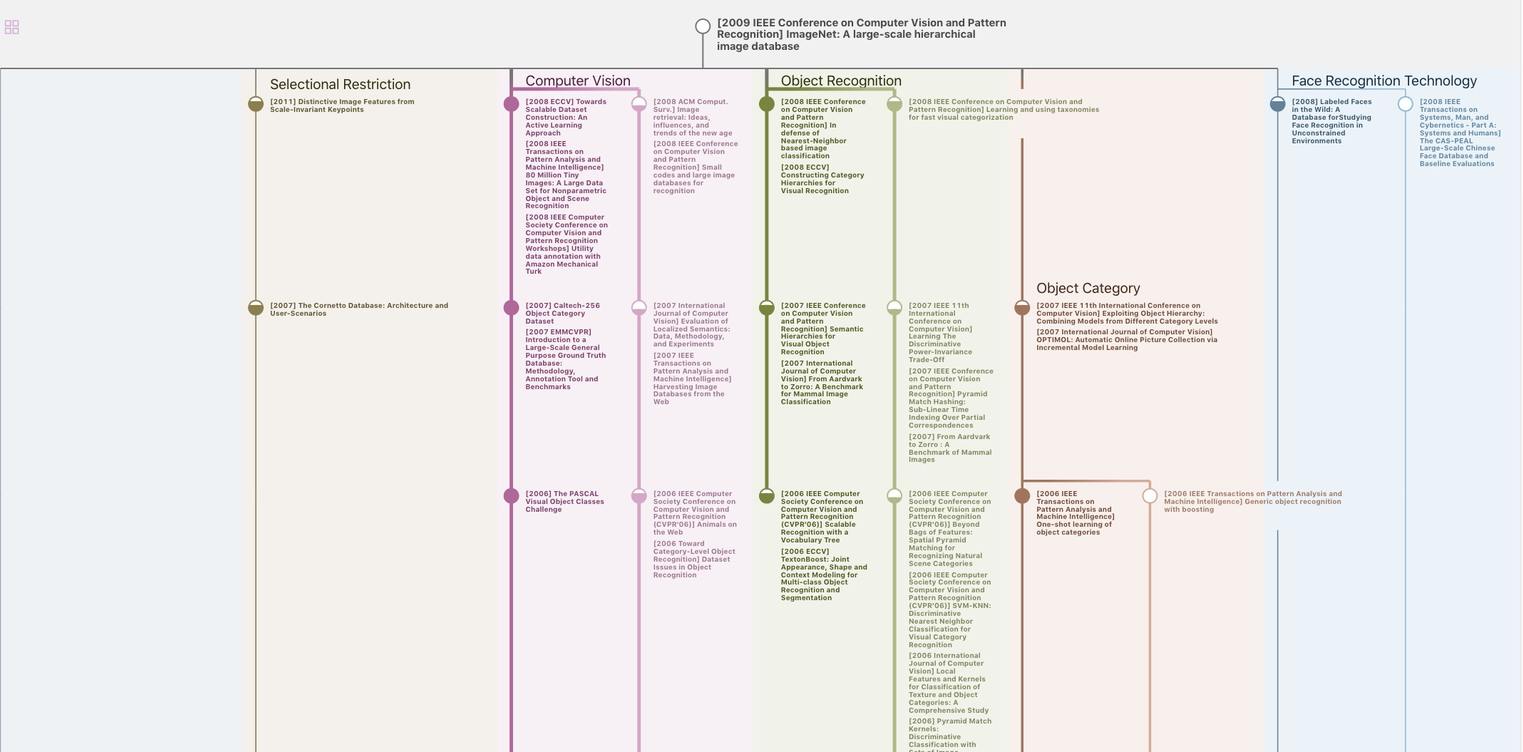
Generate MRT to find the research sequence of this paper
Chat Paper
Summary is being generated by the instructions you defined