A meta reinforcement learning account of behavioral adaptation to volatility in recurrent neural networks
crossref(2024)
摘要
Natural environments often exhibit various degrees of volatility, ranging from slowly changing to rapidly changing contingencies. How learners adapt to changing environments is a central issue in both reinforcement learning theory and psychology. For example, learners may adapt to changes in volatility by increasing learning if volatility increases, and reducing it if volatility decreases. Computational neuroscience and neural network modeling work suggests that this adaptive capacity may result from a meta-reinforcement learning process (implemented for example in the prefrontal cortex), where past experience endows the system with the capacity to rapidly adapt to environmental conditions in new situations. Here we provide direct evidence for a meta-reinforcement learning account of adaptation to environmental volatility. Recurrent neural networks (RNNs) were trained on a restless four-armed bandit reinforcement learning problem under three different training regimes (low volatility training only, medium volatility training only, or meta-volatility training across a range of volatilities). We show that, in contrast to RNNs trained in the low volatility regime, RNNs trained under the medium or meta-volatility regimes exhibited a superior adaption to environmental volatility. This was reflected in a better performance, and computational modeling of the networks behavior revealed a more adaptive adjustment of learning and exploration to varying levels of volatility during test. Results extend the meta-RL account to volatility adaptation and confirm past experience as a crucial factor of adaptive decision-making.
### Competing Interest Statement
The authors have declared no competing interest.
更多查看译文
AI 理解论文
溯源树
样例
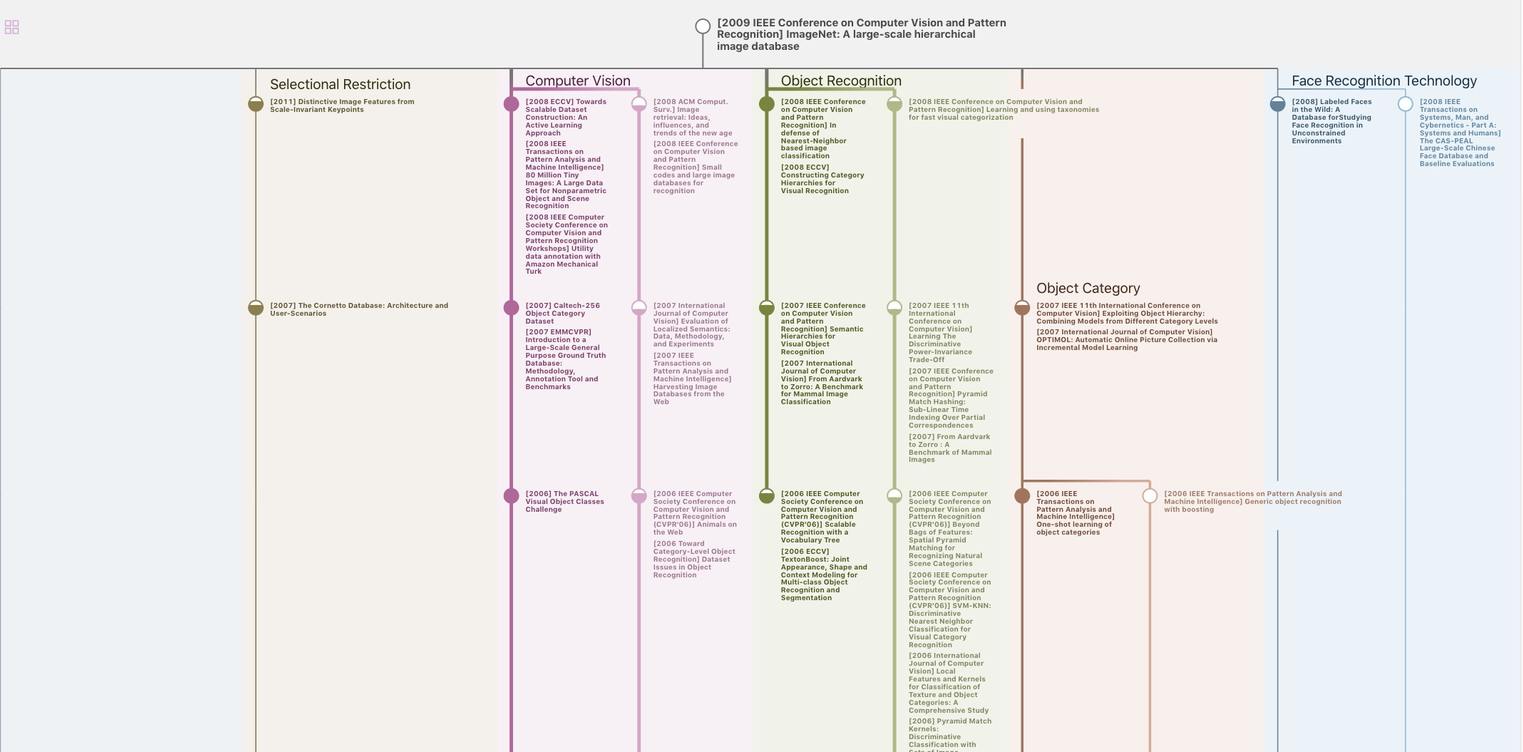
生成溯源树,研究论文发展脉络
Chat Paper
正在生成论文摘要