Mild cognitive impairment detection from facial video interviews by applying spatial-to-temporal attention module
Expert Systems with Applications(2024)
摘要
Early detection of Mild Cognitive Impairment (MCI) leads to early interventions to slow the progression from MCI into dementia. Deep Learning (DL) algorithms could help achieve early non-invasive and low-cost detection of MCI. This paper presents the detection of MCI in older adults using DL models based only on facial features extracted from video-recorded conversations at home. We used the data collected from the I-CONECT behavioral intervention study (NCT02871921), where several sessions of semi-structured interviews between socially isolated older individuals and interviewers were video recorded. We develop a framework that extracts holistic spatial facial features using a convolutional autoencoder and temporal information using transformers. We proposed the Spatial-to-Temporal Attention Module (STAM) to detect the I-CONECT study participants’ cognitive conditions (MCI vs. those with normal cognition (NC)) using facial and interaction features. The interaction features of the facial features improved the prediction performance compared with applying facial features solely. The detection accuracy using this combined method reached 88%, whereas the accuracy without applying the segments and sequences information of the facial features within a video on a certain theme was 84%. Overall, the results show that spatiotemporal facial features modeled using DL algorithms have a discriminating power for the detection of MCI.
更多查看译文
关键词
Facial features,Deep learning,Mild cognitive impairment,Causal conversation,Older adults
AI 理解论文
溯源树
样例
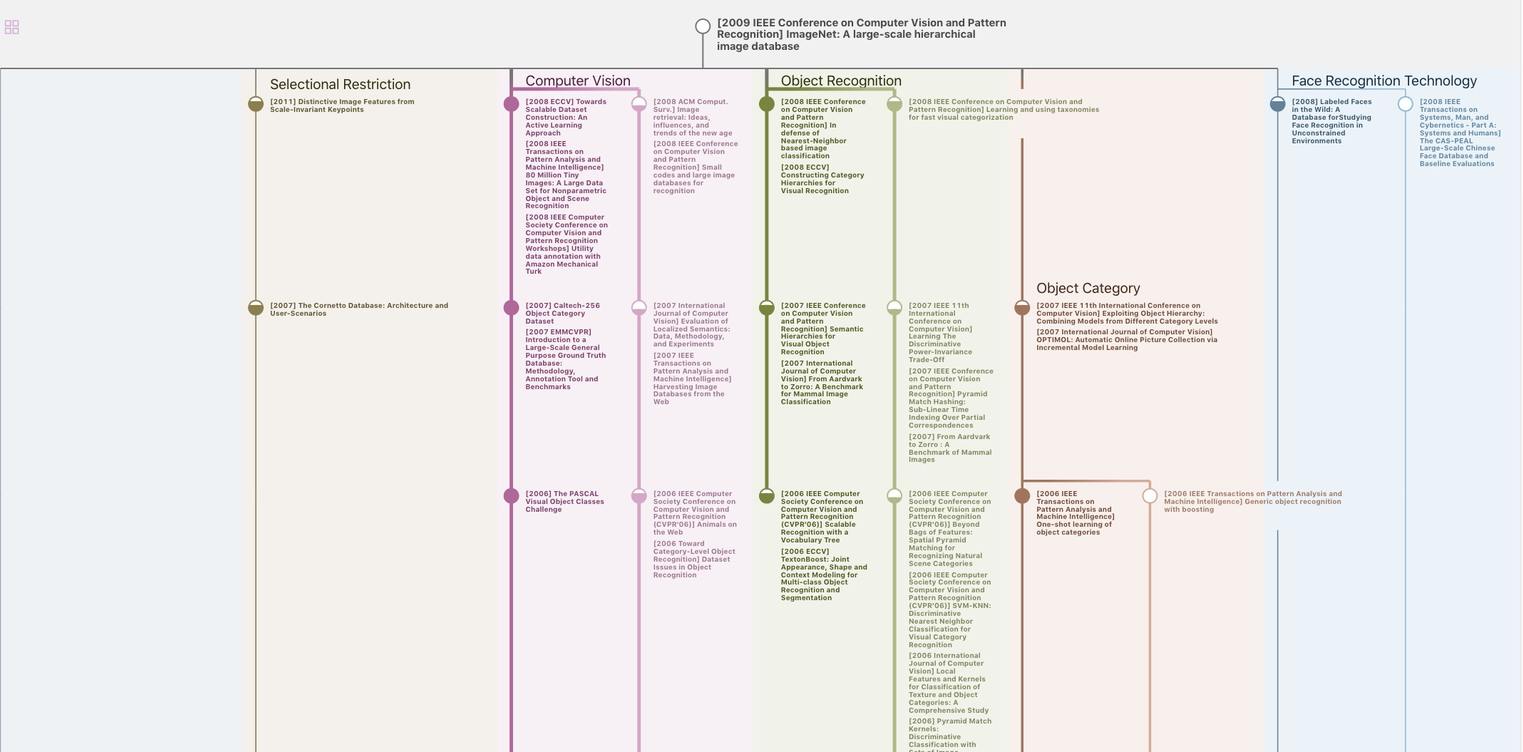
生成溯源树,研究论文发展脉络
Chat Paper
正在生成论文摘要