Pruning techniques for artificial intelligence networks: a deeper look at their engineering design and bias: the first review of its kind
Multimedia Tools and Applications(2024)
摘要
Trained Artificial Intelligence (AI) models are challenging to install on edge devices as they are low in memory and computational power. Pruned AI (PAI) models are therefore needed with minimal degradation in performance. This is the first study of its kind where we have characterized three types of PAI paradigms, namely, weight pruning (WP), channel pruning (CP), and hybrid pruning (HP). Further, the study also analyses the risk of bias (RoB) in PAI models, and explainable PAI (XPAI) networks. PRISMA model was adapted for the selection of the best 145 studies. The review had two hypotheses: (i) PAI had bias and (ii) the order of performance for the three PAI is: HP > CP > WP in terms of performance. Thirty-two AI attributes using each of two experts were categorized into four clusters, namely, architecture, optimization, dataset design, and scientific validation. The RoB was analyzed in these PAI studies using AP(ai)Bias 2.0 (AtheroPoint™, CA, USA), which was based on a scoring-and-ranking strategy. This was then compared against the Butterfly model, composed of radial and regional biases, and analyzed using a Venn diagram in three independent bins based on low-moderate and moderate-high cut-offs. Recommendations were laid out for low-bias studies. PAI studies that lack adequate AI attributes were more inclined to the RoB. It has been observed that 19.64
更多查看译文
关键词
Artificial Intelligence,Resource constraint devices,Training model sizes,Pruning,Quantization,Risk of bias,Explainability
AI 理解论文
溯源树
样例
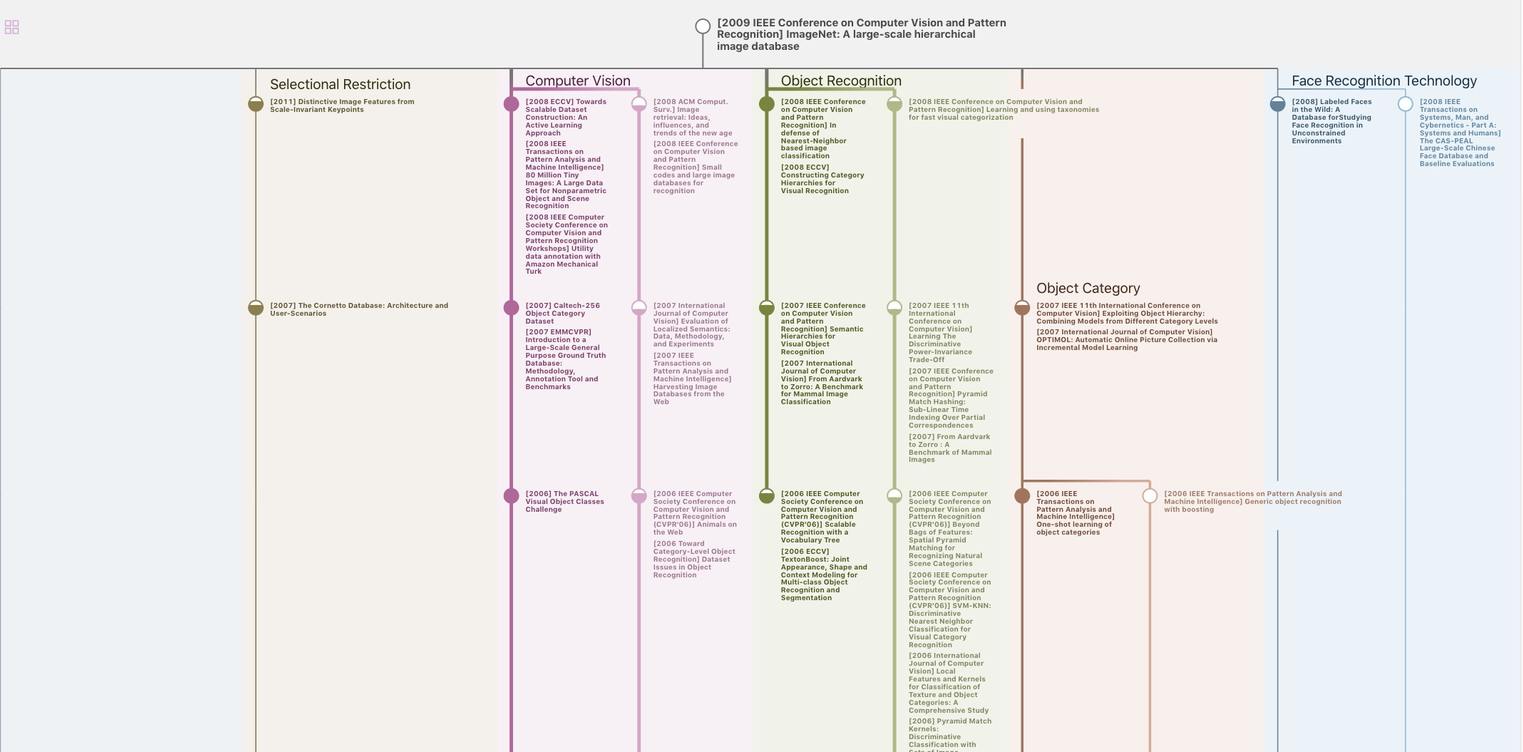
生成溯源树,研究论文发展脉络
Chat Paper
正在生成论文摘要