Aligned Side Information Fusion Method for Sequential Recommendation
WWW 2024(2024)
Abstract
Combining contextual information (i.e., side information) of items beyond IDs has become an important way to improve the performance in recommender systems. Existing self-attention-based side information fusion methods can be categorized into early, late, and hybrid fusion. In practice, naive early fusion may interfere with the representation of IDs, resulting in negative effects, while late fusion misses effective interactions between IDs and side information. Some hybrid methods have been proposed to address these issues, but they only utilize side information in calculating attention scores, which may lead to information loss. To harness the full potential of side information without noisy interference, we propose an Aligned Side Information Fusion (ASIF) method for sequential recommendation, consisting of two parts: Fused Attention with Untied Positions and Representation Alignment. Specifically, we first decouple the positions to exclude the noisy interference in the attention scores. Secondly, we adopt the contrastive objective to maintain the semantic consistency between IDs and side information and then employ orthogonal decomposition to extract the homogeneous parts. By aligning the representations and fusing them together, ASIF makes full use of the side information without interfering with IDs. Offline experimental results on four datasets demonstrate the superiority of ASIF. Additionally, we successfully deployed the model in Alipay's advertising system and achieved 1.09% and 1.86% improvements on clicks and Cost Per Mille (CPM).
MoreTranslated text
AI Read Science
Must-Reading Tree
Example
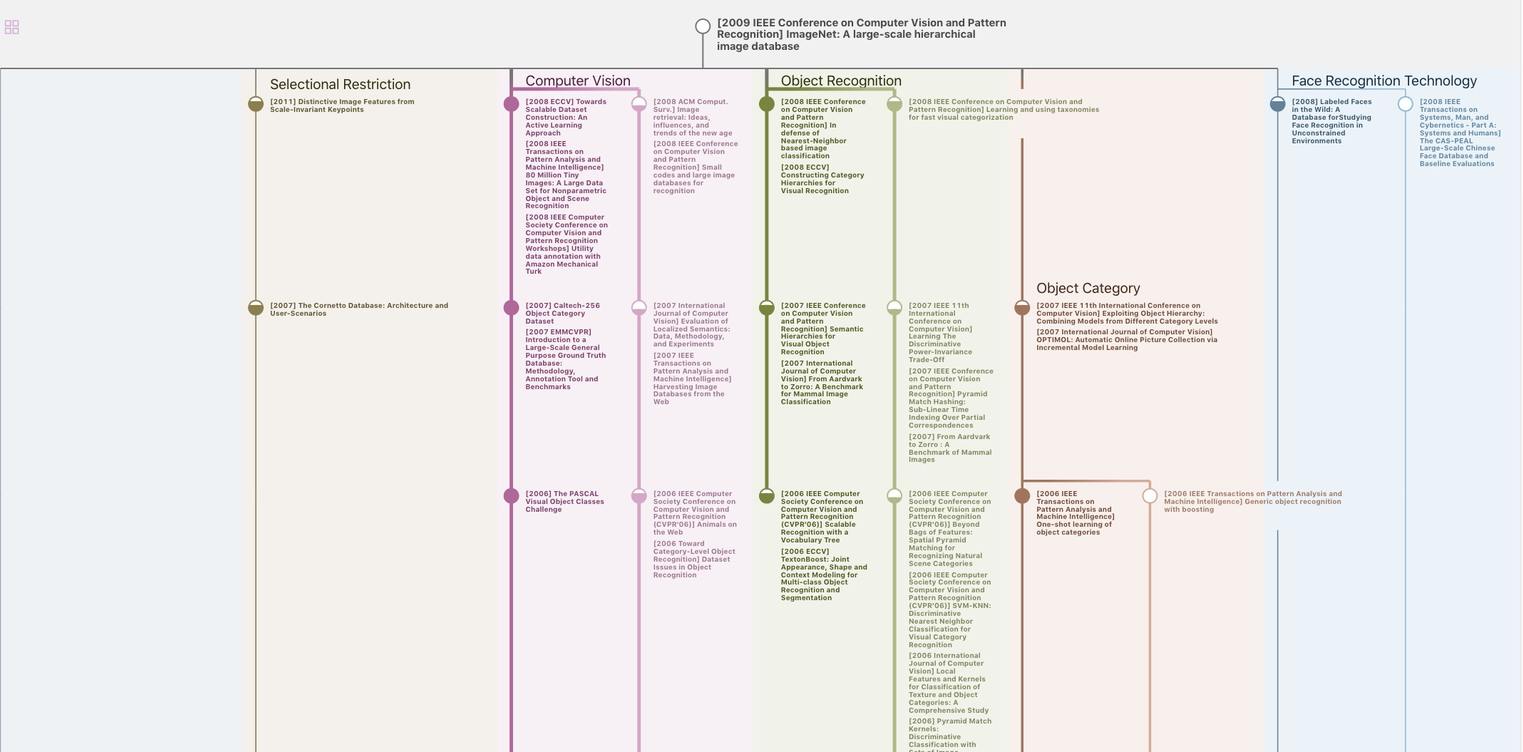
Generate MRT to find the research sequence of this paper
Chat Paper
Summary is being generated by the instructions you defined