A Novel Algorithm for Classifying Vegetation and Non-Vegetation Using Red Edge Slope Coefficient with Hyperspectral LCTF Imaging
crossref(2024)
摘要
Hyperspectral imaging, a rapidly evolving technique, furnishes exhaustive information on Earth's surface reflectance over an extensive spectrum of wavelengths. We have developed a more precise method for analyzing spectral data by meticulously selecting plant leaf pixels and employing a relative reference approach. This enhanced technique correlates camera pixel values with light intensity, bypassing the need for complex equations and thereby providing clearer insights into vegetation vitality. The findings of this study not only enhance the precision of hyperspectral imaging but also bolster our capability for ecological monitoring and understanding environmental health. A novel algorithm based on the red-edge slope was established to classify images, exploiting the spectral reflectance data gleaned from the hyperspectral Liquid Crystal Tunable Filter (LCTF) camera. Compared with conventional SVM and K-means algorithms, our novel algorithm demonstrated commendable performance, achieving an overall accuracy of 90% with a Kappa Coefficient of 0.86. This comparison highlights the novel algorithm's relative strength and potential for large-scale satellite and drone imagery analysis applications. The advancements facilitated by this research are instrumental in ecological assessments, conservation efforts, and resource management strategies, signaling a leap forward in the era of environmental monitoring and analysis.
更多查看译文
AI 理解论文
溯源树
样例
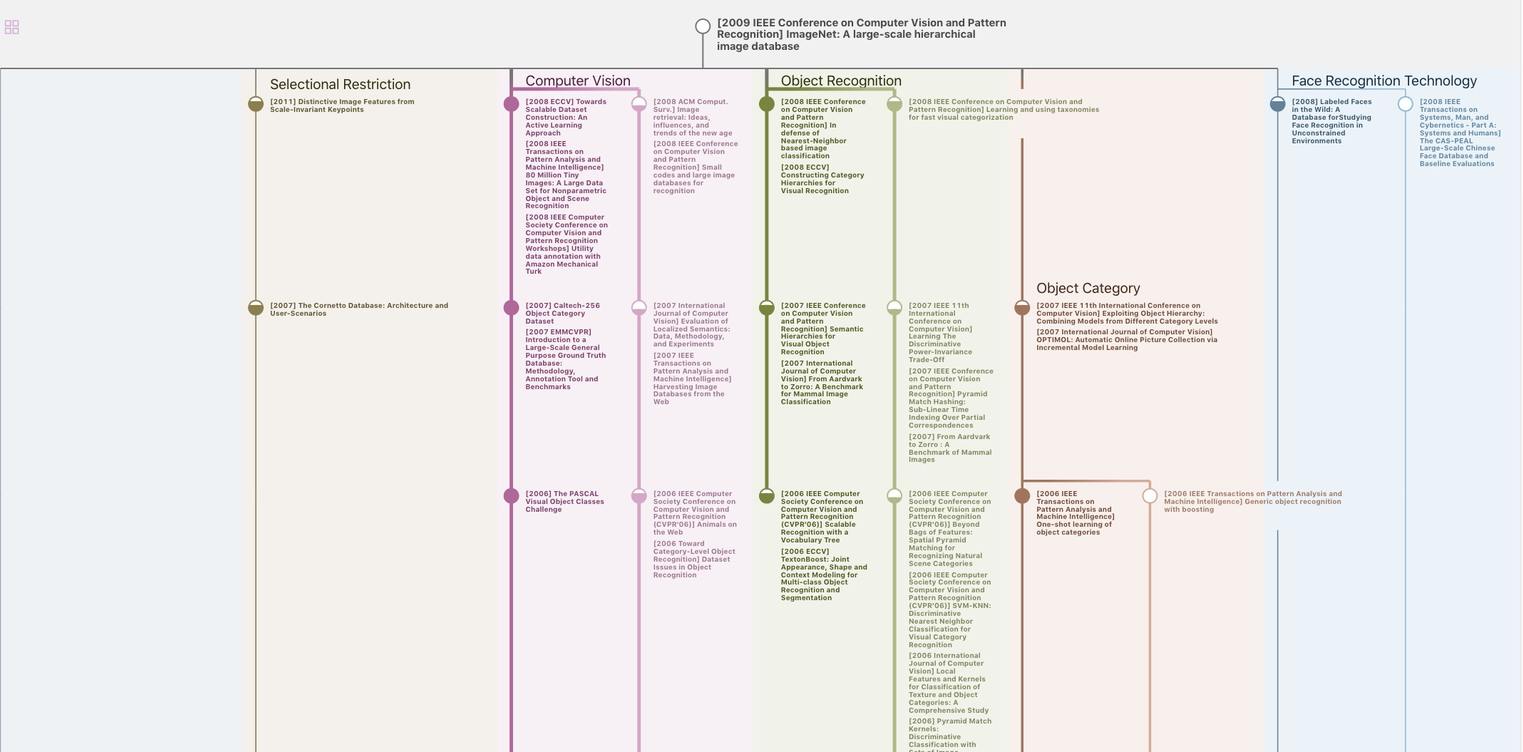
生成溯源树,研究论文发展脉络
Chat Paper
正在生成论文摘要