Assessing Fairness of Residential Dynamic Pricing for Electricity Using Active Learning with Agent-based Simulation.
AAMAS '24 Proceedings of the 23rd International Conference on Autonomous Agents and Multiagent Systems(2024)
摘要
Extreme weather events and fast-paced adoption of green energy technologies have led to new challenges in demand-side management, maintaining grid reliability, and fulfilling variable consumer demands One of the effective ways to address these difficulties is by introducing economic incentives - replacing the flat rate tariffs with dynamic tariffs. However, dynamic pricing schemes need to be designed carefully to consider fairness and benefits for consumers as well as power companies. This paper describes an ML-based simulation framework for exploring two fairness constructs of dynamic pricing for residential electricity with behavioral agent-based models based on social theory combined with active learning. As an example, we simulate behavior adaptations in response to changes in electricity prices to study cost savings through monthly bills and peak demand reduction in synthetic household agents in a Time Of Use (TOU) pricing scheme in Virginia, USA. Further, we can show that there exists a region in the parameter space that corresponds to a fair TOU pricing scheme for both entities: all income-stratified communities and power companies.
更多查看译文
AI 理解论文
溯源树
样例
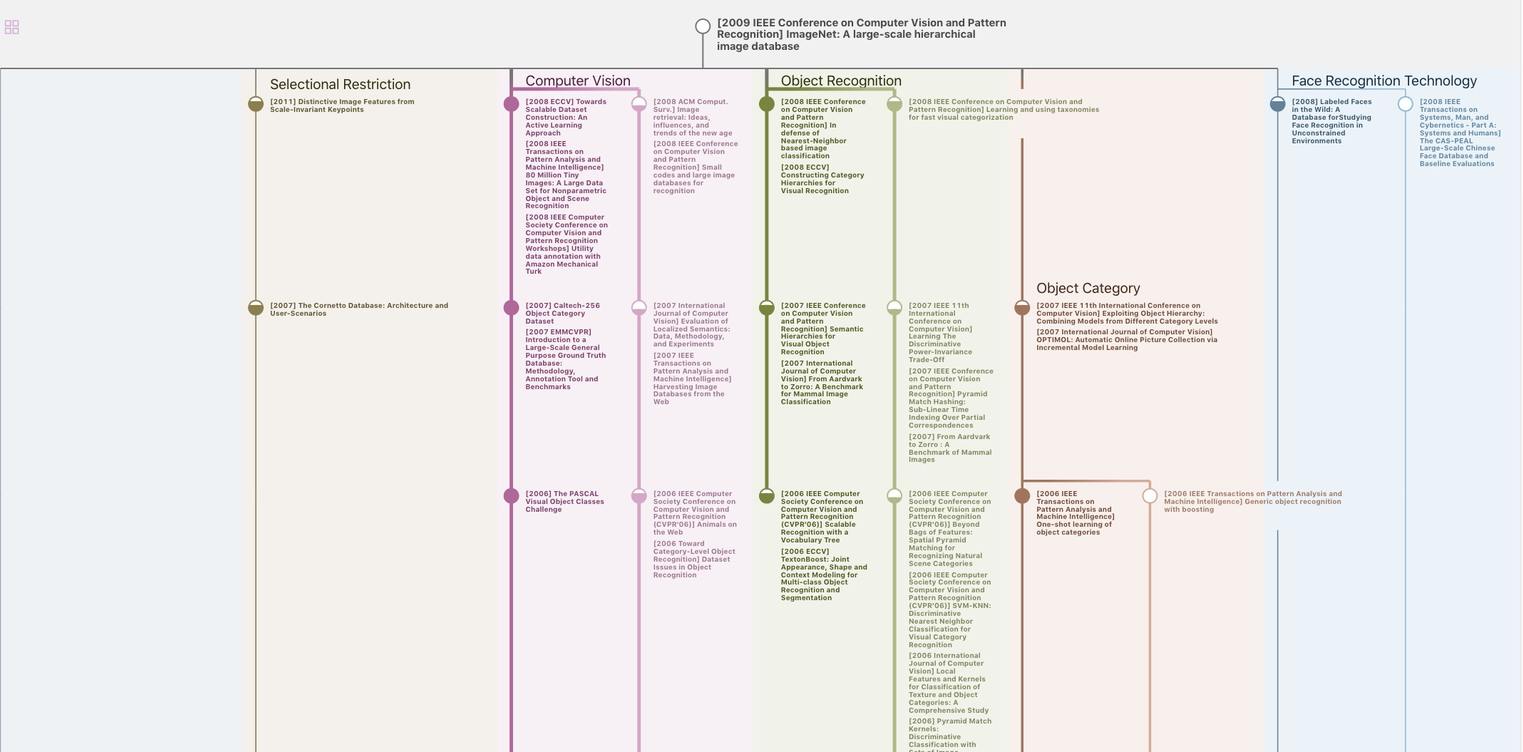
生成溯源树,研究论文发展脉络
Chat Paper
正在生成论文摘要