Aligning Credit for Multi-Agent Cooperation via Model-based Counterfactual Imagination.
International Joint Conference on Autonomous Agents & Multiagent Systems(2024)
Abstract
Recent years have witnessed considerable progress in model-based reinforcement learning research. Inspired by the significant improvement in sample efficiency, researchers have explored its application in multi-agent scenarios to mitigate the huge demands in training data of multi-agent reinforcement learning (MARL) approaches. However, existing methods retain the training framework designed for single-agent settings, resulting in inadequate promotion of multi-agent cooperation. In this work, we propose a novel model-based MARL method called Multi-Agent Counterfactual Dreamer (MACD). MACD introduces a centralized imagination with decentralized execution (CIDE) framework to generate higher-quality pseudo data for policy learning, thus further improving the algorithm's sample efficiency. Moreover, we address the credit assignment and non-stationary challenges by performing an additional counterfactual trajectory based on the learned world model. We provide a theoretical proof that this counterfactual policy update rule maximizes the multi-agent learning objective. Empirical studies validate the superiority of our method in terms of sample efficiency, training stability, and final cooperation performance when compared with several state-of-the-art model-free and model-based MARL algorithms. Ablation studies and visualization demonstration further underscore the significance of both the CIDE framework and the counterfactual module in our approach.
MoreTranslated text
AI Read Science
Must-Reading Tree
Example
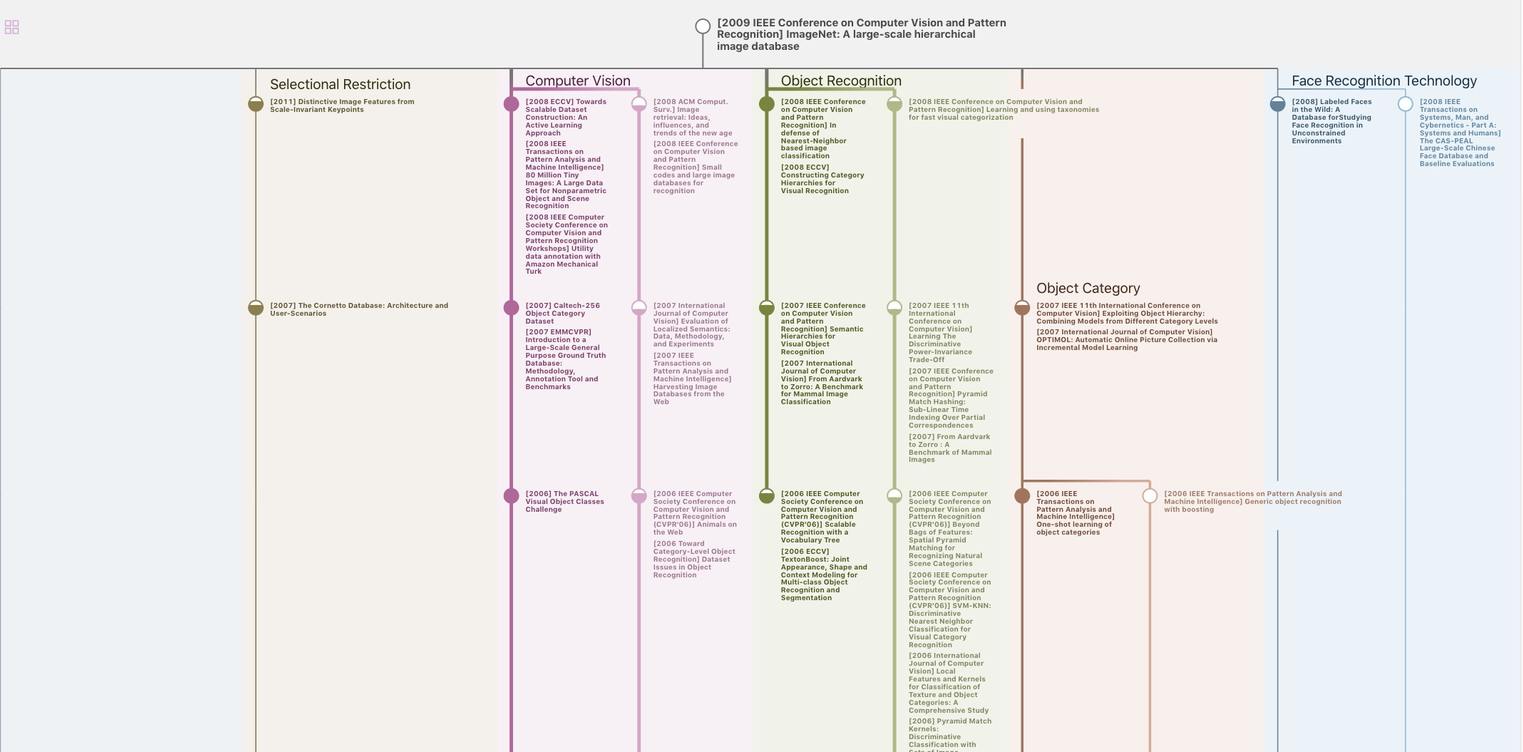
Generate MRT to find the research sequence of this paper
Chat Paper
Summary is being generated by the instructions you defined