Short-Term Wind Power Prediction Based on DTW Error Diagnosis and Transformer Optimization Model
2023 IEEE 7th Conference on Energy Internet and Energy System Integration (EI2)(2023)
摘要
Wind power prediction is significant in the modern electrical systems. Current wind power prediction always uses a single deep learning model, which will lead to issues such as reduced volatility and overly smooth curves in the results. Consequently, short-term wind power prediction method based on DTW error diagnosis and Transformer optimization model is proposed in this paper. The method involves segmenting historical databases, utilizing long-short term memory (LSTM) neural network for prediction and performing validation set error scenario partitioning. Then, high-error scenarios in both the test and validation sets are matched by using the Dynamic Time Warping (DTW) method. Finally, for diagnosing the high error scenarios identified in the test set, a separate Transformer model was constructed. The results show that, using the proposed method, the prediction accuracy can increase by 1.53%, 1.05% and 0.52% compared to using a single LSTM model, using a single Transformer model, and using a Backpropagation Neural Network (BPNN) after error diagnosis. This research provides valuable insights for error diagnosis and short-term power prediction in wind power clusters.
更多查看译文
关键词
Short-term wind power forecast,Error diagnosis,DTW,Transformer
AI 理解论文
溯源树
样例
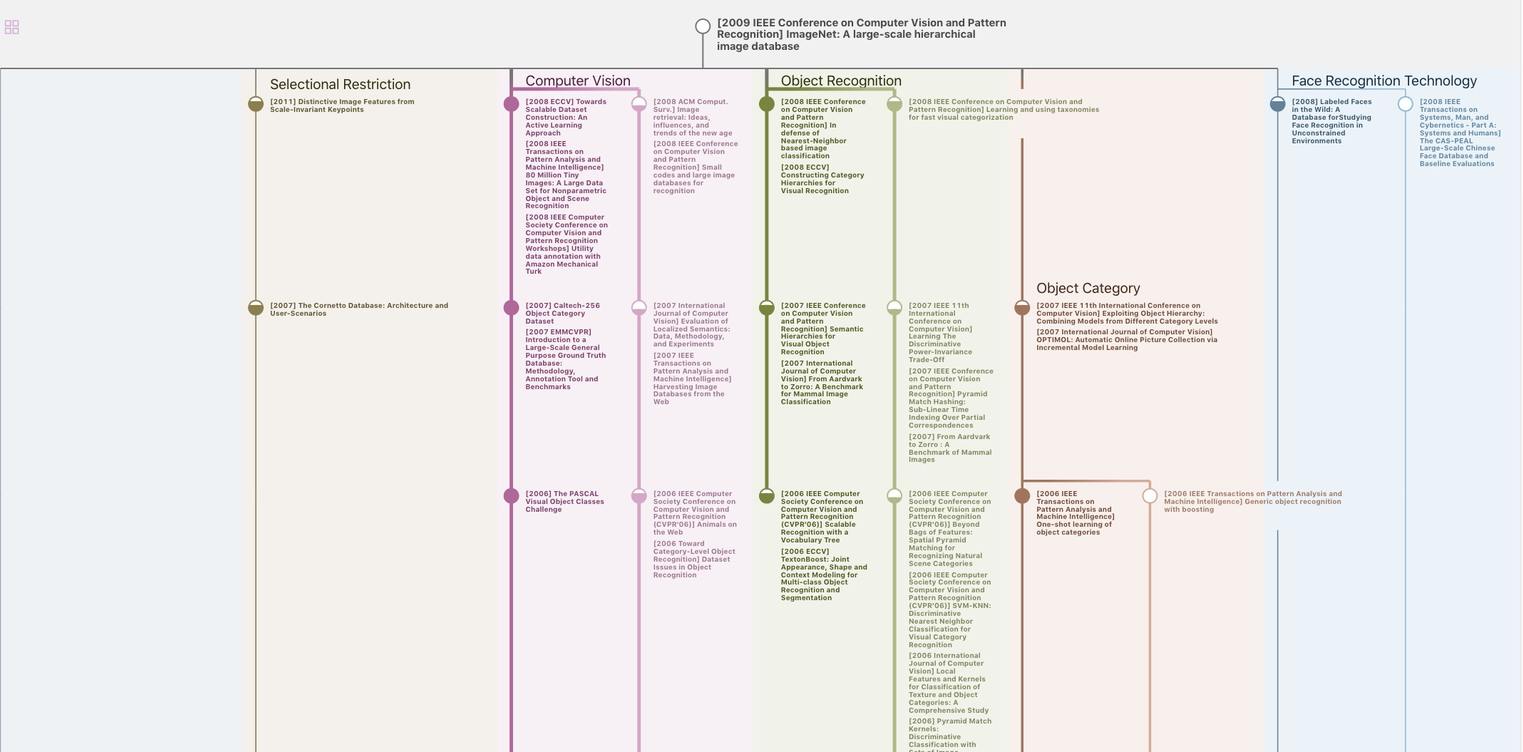
生成溯源树,研究论文发展脉络
Chat Paper
正在生成论文摘要