Distribution shift detection for the postmarket surveillance of medical AI algorithms: a retrospective simulation study
NPJ DIGITAL MEDICINE(2024)
Abstract
Distribution shifts remain a problem for the safe application of regulated medical AI systems, and may impact their real-world performance if undetected. Postmarket shifts can occur for example if algorithms developed on data from various acquisition settings and a heterogeneous population are predominantly applied in hospitals with lower quality data acquisition or other centre-specific acquisition factors, or where some ethnicities are over-represented. Therefore, distribution shift detection could be important for monitoring AI-based medical products during postmarket surveillance. We implemented and evaluated three deep-learning based shift detection techniques (classifier-based, deep kernel, and multiple univariate kolmogorov-smirnov tests) on simulated shifts in a dataset of 130'486 retinal images. We trained a deep learning classifier for diabetic retinopathy grading. We then simulated population shifts by changing the prevalence of patients' sex, ethnicity, and co-morbidities, and example acquisition shifts by changes in image quality. We observed classification subgroup performance disparities w.r.t. image quality, patient sex, ethnicity and co-morbidity presence. The sensitivity at detecting referable diabetic retinopathy ranged from 0.50 to 0.79 for different ethnicities. This motivates the need for detecting shifts after deployment. Classifier-based tests performed best overall, with perfect detection rates for quality and co-morbidity subgroup shifts at a sample size of 1000. It was the only method to detect shifts in patient sex, but required large sample sizes (> 30'000). All methods identified easier-to-detect out-of-distribution shifts with small (<= 300) sample sizes. We conclude that effective tools exist for detecting clinically relevant distribution shifts. In particular classifier-based tests can be easily implemented components in the post-market surveillance strategy of medical device manufacturers.
MoreTranslated text
AI Read Science
Must-Reading Tree
Example
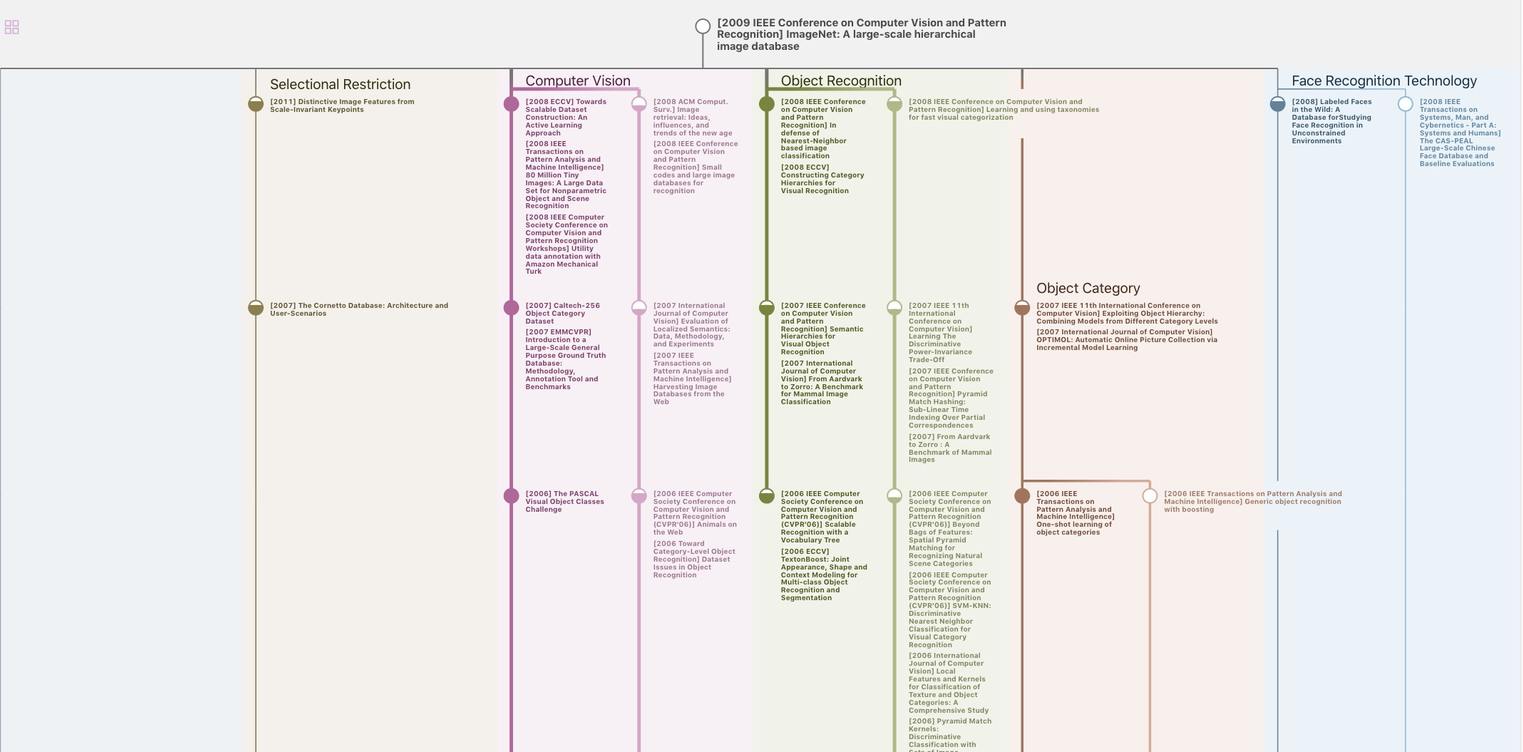
Generate MRT to find the research sequence of this paper
Chat Paper
Summary is being generated by the instructions you defined