Learning De-biased prototypes for few-shot medical image segmentation
Pattern Recognition Letters(2024)
摘要
Prototypical networks have emerged as the dominant method for Few-shot Medical image Segmentation (FSMIS). Despite their success, the commonly used Masked Average Pooling (MAP) approach in prototypical networks computes the mean of the masks, resulting in imprecise and inadequate prototypes that fail to capture the subtle nuances and variations in the data. To address this issue, we propose a simple yet effective module called De-biasing Masked Average Pooling (DMAP) to generate more accurate prototypes from filtered foreground support features. Specifically, our approach introduces a Learnable Threshold Generation (LTG) module that adaptively learns a threshold based on the extracted features from both support and query images, and then choose partial foreground pixels that have larger similarity than the threshold to generate prototypes. Our proposed method is evaluated on three popular medical image segmentation datasets, and the results demonstrate the superiority of our approach over some state-of-the-art methods. Code is available at https://github.com/YazhouZhu19/DMAP.
更多查看译文
关键词
Few-shot learning,Medical image segmentation,De-biased prototypes,Learnable threshold
AI 理解论文
溯源树
样例
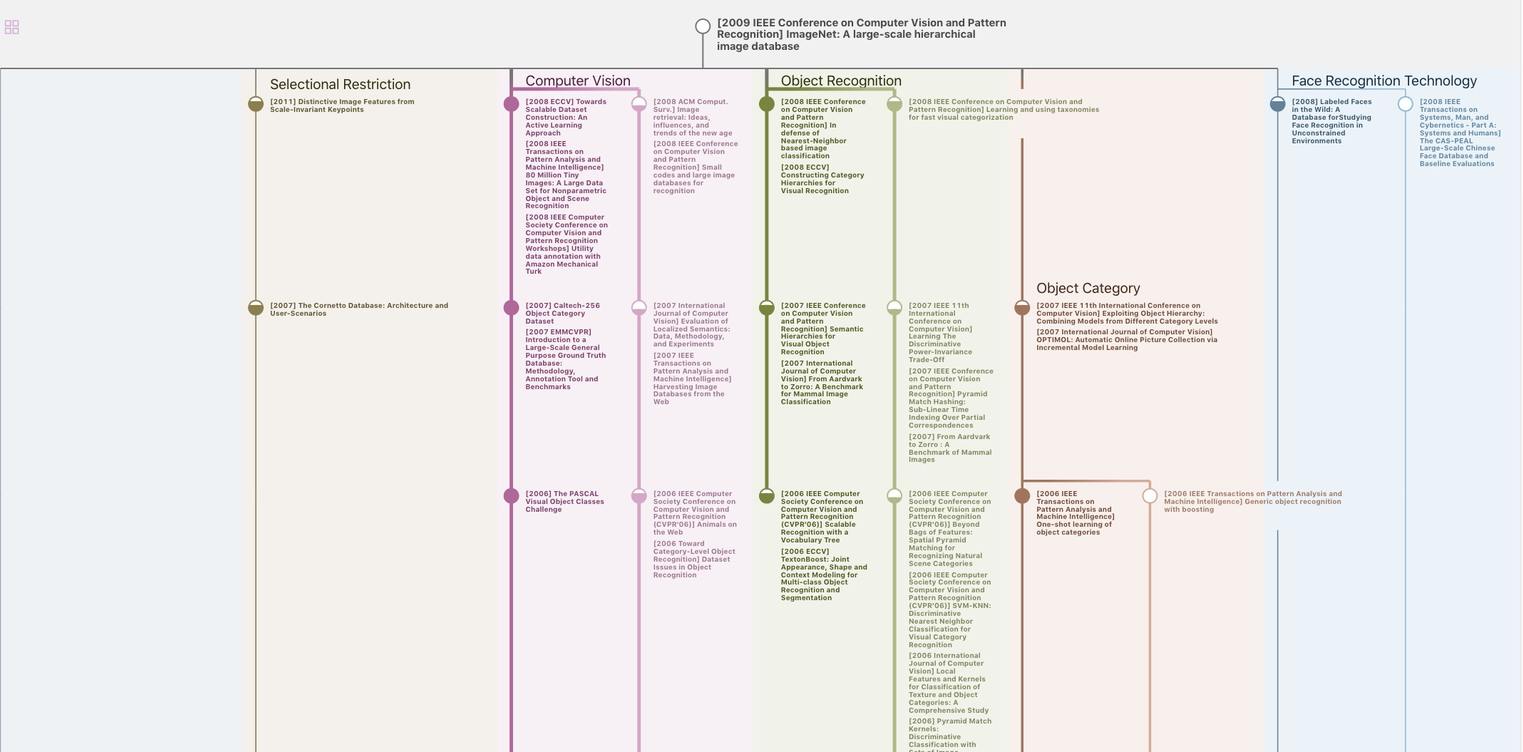
生成溯源树,研究论文发展脉络
Chat Paper
正在生成论文摘要