Joint Optimization of Model Partition and Resource Allocation for Split Federated Learning over Vehicular Edge Networks
IEEE Transactions on Vehicular Technology(2024)
摘要
Split federated learning (SFL) has been regarded as an efficient paradigm to enable both federated learning and reduce the computation burdens at the devices by allowing them to train parts of the model. However, deploying SFL over resource-constrained vehicular edge networks is challenging, and a cost-effective scheme is necessitated to minimize the total time and energy consumption of vehicular devices. To this end, we use an improved reinforcement learning method to present a joint optimization scheme that can efficiently determine the optimal model partition point for each vehicular device and the optimal allocations of the computing resource and bandwidth resource among all vehicular devices. Experimental results validate the effectiveness and performance advantages of our proposed scheme.
更多查看译文
关键词
Split federated learning,model partition,resource allocation,vehicular edge networks
AI 理解论文
溯源树
样例
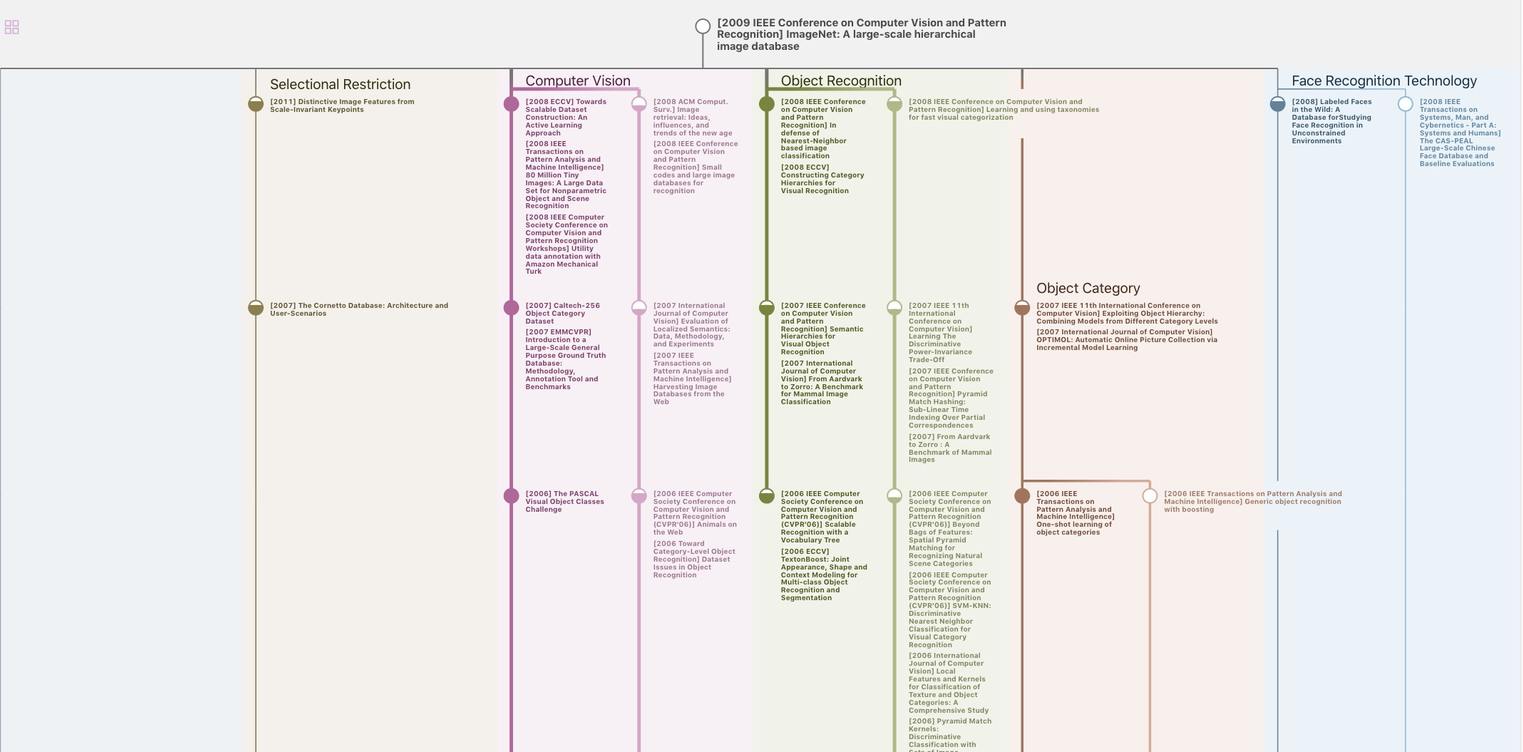
生成溯源树,研究论文发展脉络
Chat Paper
正在生成论文摘要