BiLSTM-based joint torque prediction from mechanomyogram during isometric contractions: A proof of concept study.
IEEE transactions on neural systems and rehabilitation engineering : a publication of the IEEE Engineering in Medicine and Biology Society(2024)
摘要
Quantifying muscle strength is an important measure in clinical settings; however, there is a lack of practical tools that can be deployed for routine assessment. The purpose of this study is to propose a deep learning model for ankle plantar flexion torque prediction from time-series mechanomyogram (MMG) signals recorded during isometric contractions (i.e., a similar form to manual muscle testing procedure in clinical practice) and to evaluate its performance. Four different deep learning models in terms of model architecture (based on a stacked bidirectional long short-term memory and dense layers), the number of units (from 32 to 512), and dropout ratio (from 0.0 to 0.8) were designed and then evaluated for prediction performance by conducting the leave-one-subject-out cross-validation method from the 10-subject dataset. As a result, the models explained more variance in the untrained test dataset as the error metrics (e.g., root-mean-square error) decreased and as the slope of the relationship between the measured and predicted joint torques became closer to 1.0. Although the slope estimates appear to be sensitive to an individual dataset, > 70% of the variance in nine out of 10 datasets was explained by the optimal model. These results demonstrated the feasibility of the proposed model as a potential tool to quantify average joint torque during a sustained isometric contraction.
更多查看译文
关键词
Manual muscle testing,muscle/joint strength,machine/deep learning model,clinical assessment
AI 理解论文
溯源树
样例
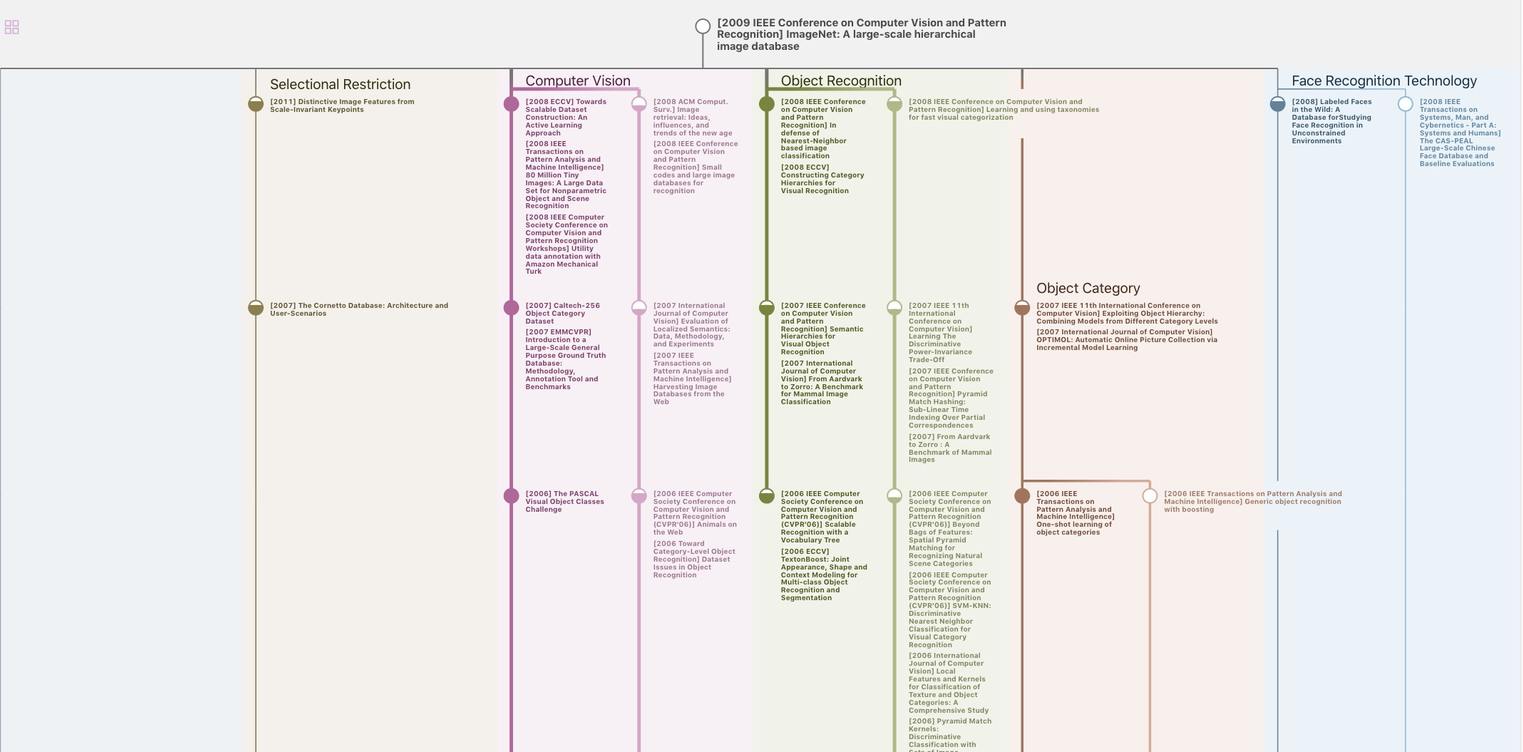
生成溯源树,研究论文发展脉络
Chat Paper
正在生成论文摘要