Medium- and Long- Term Electricity Load Forecasting Based on ARIMA-GM-LSTM
2023 IEEE 7th Conference on Energy Internet and Energy System Integration (EI2)(2023)
摘要
Accurate medium- and long-term electricity load forecasting plays an important role in the operation and planning of power system. This paper proposes an ARIMA-GM-LSTM model based on the weighted average method. The model uses the autoregressive integrated moving average (ARIMA) model to capture the periodicity and the grey model (1,1) (GM(1,1)) to fit the trend of the load curve. Besides, the long short-term memory (LSTM) network is used for getting the long-term correlation. Several criteria are used for evaluating the forecasting result. It shows that the prediction of the ARIMA-GM-LSTM model is 11.16%,26.38%, and 29.0% higher than that of the based models. That means the ARIMA-GM-LSTM model improves the accuracy and has more advantages in medium- and long-term electricity load forecasting.
更多查看译文
关键词
medium- and long-term electricity load forecasting,ARIMA model,grey model,LSTM,combined forecasting
AI 理解论文
溯源树
样例
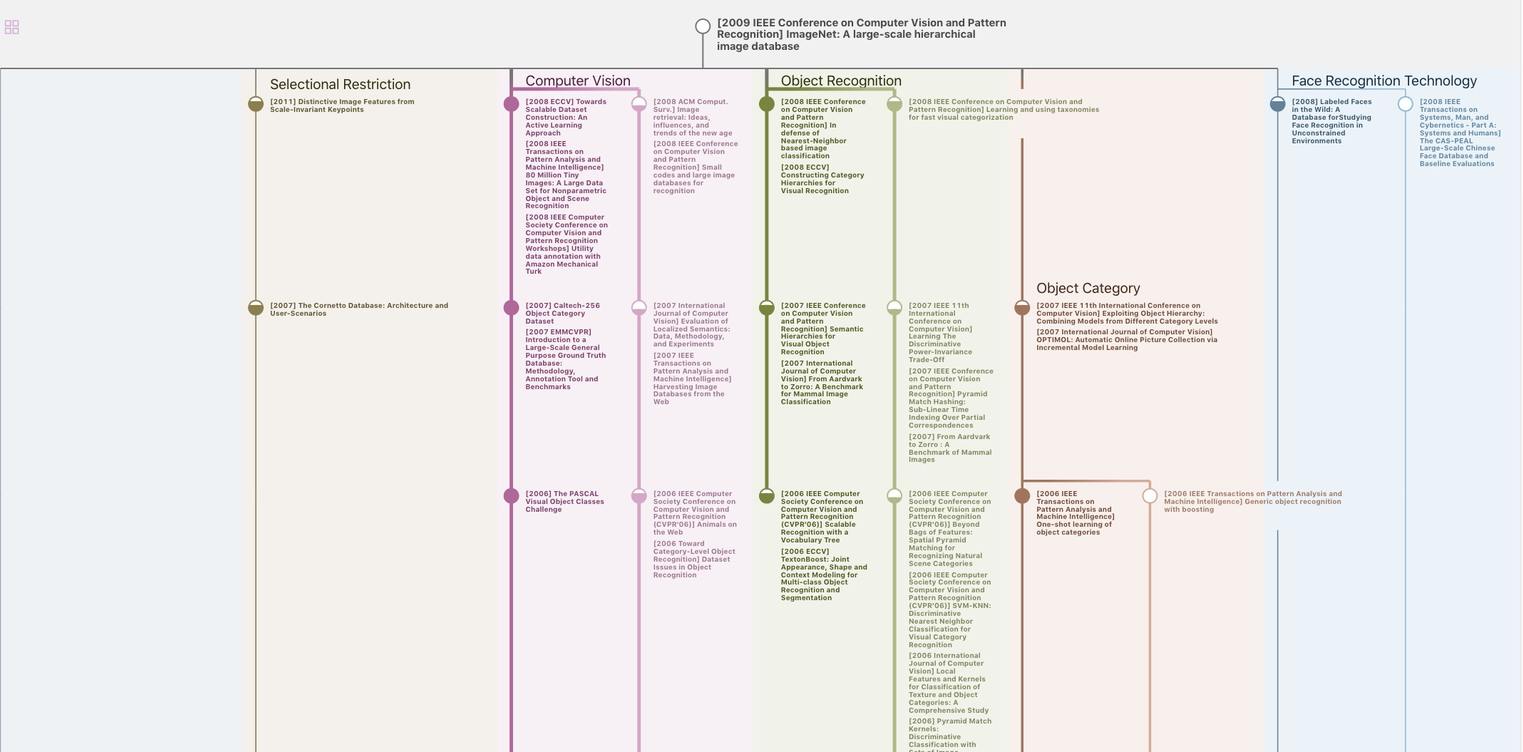
生成溯源树,研究论文发展脉络
Chat Paper
正在生成论文摘要