Mask-TS Net: Mask Temperature Scaling Uncertainty Calibration for Polyp Segmentation
CoRR(2024)
Abstract
Lots of popular calibration methods in medical images focus on
classification, but there are few comparable studies on semantic segmentation.
In polyp segmentation of medical images, we find most diseased area occupies
only a small portion of the entire image, resulting in previous models being
not well-calibrated for lesion regions but well-calibrated for background,
despite their seemingly better Expected Calibration Error (ECE) scores overall.
Therefore, we proposed four-branches calibration network with Mask-Loss and
Mask-TS strategies to more focus on the scaling of logits within potential
lesion regions, which serves to mitigate the influence of background
interference. In the experiments, we compare the existing calibration methods
with the proposed Mask Temperature Scaling (Mask-TS). The results indicate that
the proposed calibration network outperforms other methods both qualitatively
and quantitatively.
MoreTranslated text
AI Read Science
Must-Reading Tree
Example
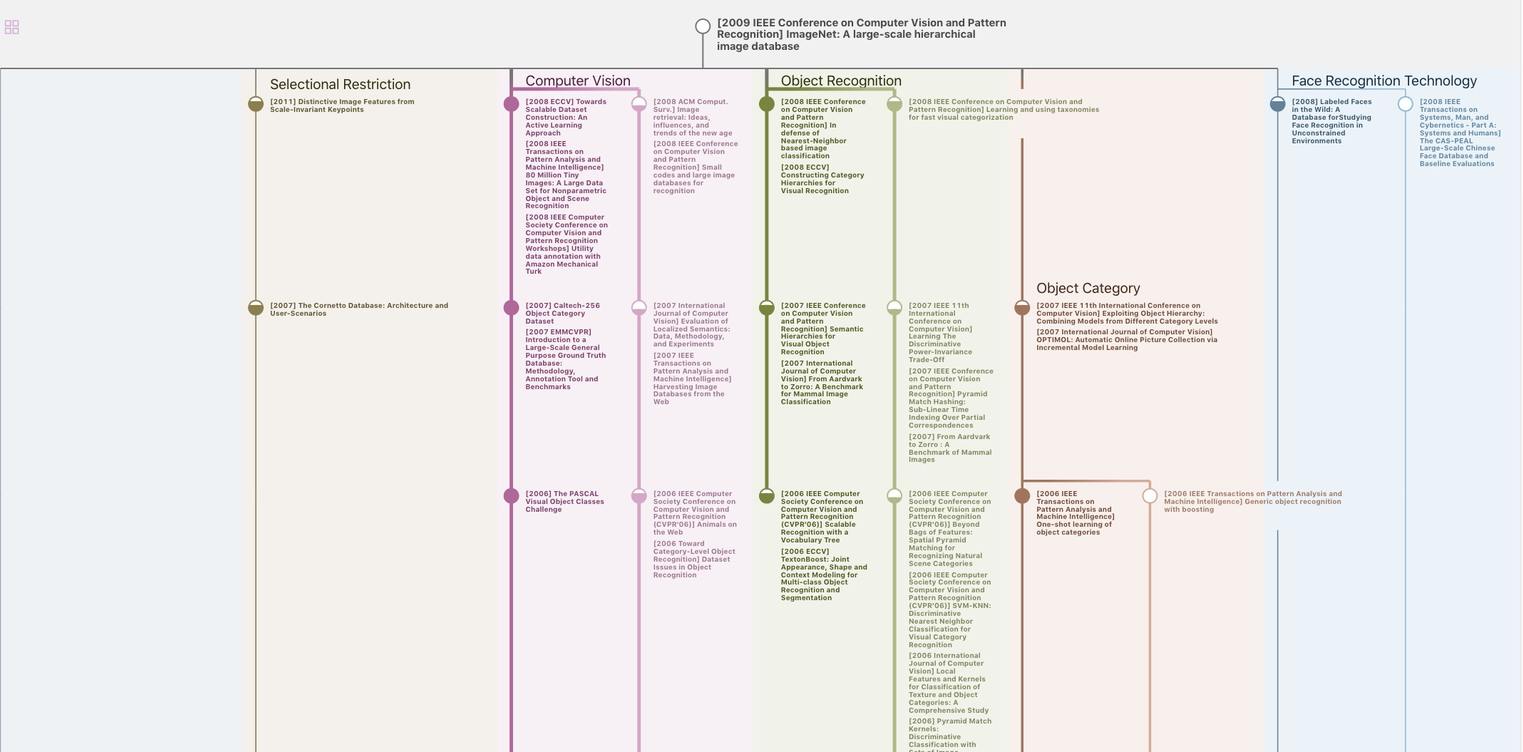
Generate MRT to find the research sequence of this paper
Chat Paper
Summary is being generated by the instructions you defined