Efficient Pretraining Model based on Multi-Scale Local Visual Field Feature Reconstruction for PCB CT Image Element Segmentation
CoRR(2024)
摘要
Element segmentation is a key step in nondestructive testing of Printed
Circuit Boards (PCB) based on Computed Tomography (CT) technology. In recent
years, the rapid development of self-supervised pretraining technology can
obtain general image features without labeled samples, and then use a small
amount of labeled samples to solve downstream tasks, which has a good potential
in PCB element segmentation. At present, Masked Image Modeling (MIM)
pretraining model has been initially applied in PCB CT image element
segmentation. However, due to the small and regular size of PCB elements such
as vias, wires, and pads, the global visual field has redundancy for a single
element reconstruction, which may damage the performance of the model. Based on
this issue, we propose an efficient pretraining model based on multi-scale
local visual field feature reconstruction for PCB CT image element segmentation
(EMLR-seg). In this model, the teacher-guided MIM pretraining model is
introduced into PCB CT image element segmentation for the first time, and a
multi-scale local visual field extraction (MVE) module is proposed to reduce
redundancy by focusing on local visual fields. At the same time, a simple
4-Transformer-blocks decoder is used. Experiments show that EMLR-seg can
achieve 88.6
by the baseline model, and the training time is reduced by 29.6 hours, a
reduction of 17.4
advantage of EMLR-seg in terms of performance and efficiency.
更多查看译文
AI 理解论文
溯源树
样例
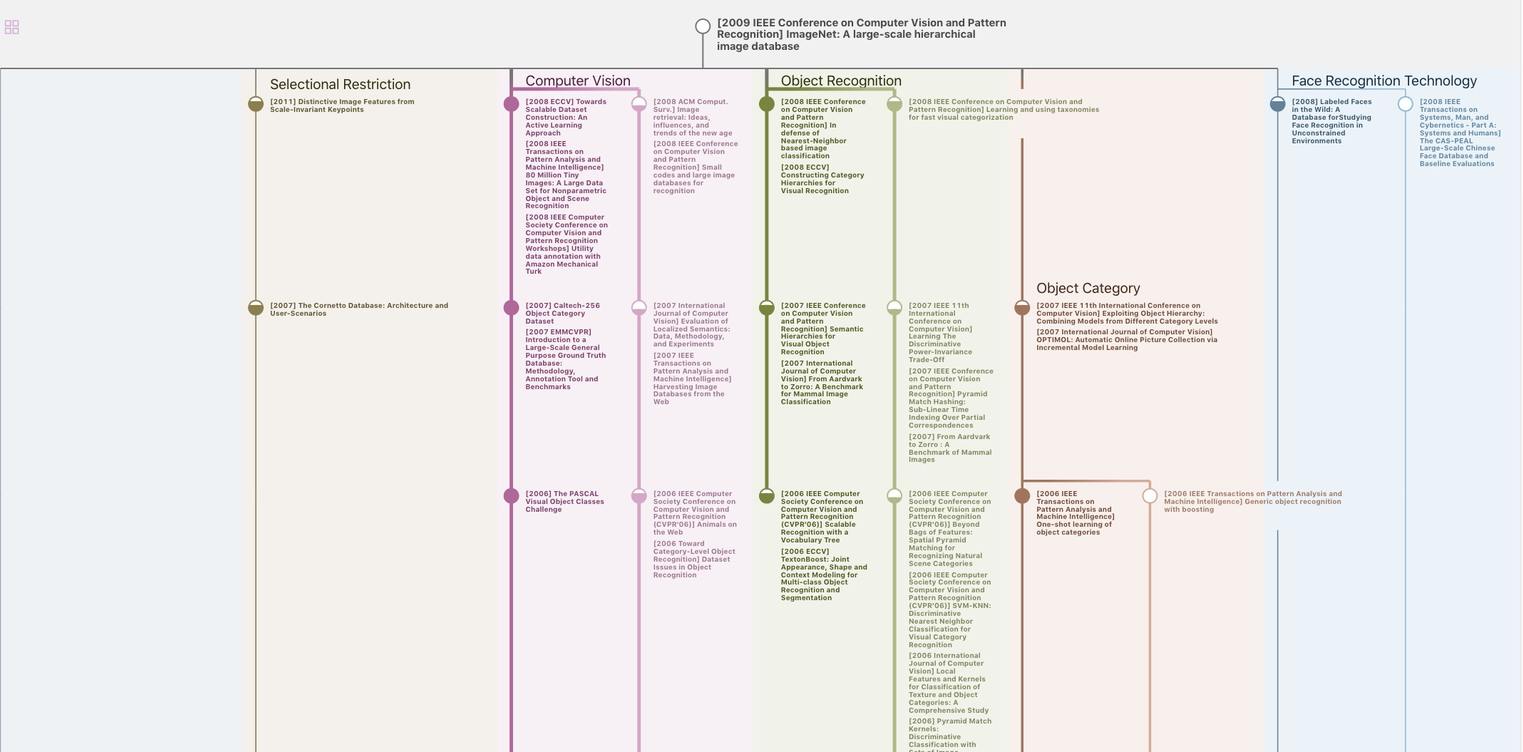
生成溯源树,研究论文发展脉络
Chat Paper
正在生成论文摘要