Transformer neural networks for closed-loop adaptive optics using non-modulated pyramid wavefront sensors
arxiv(2024)
摘要
The Pyramid Wavefront Sensor (PyWFS) is highly nonlinear and requires the use
of beam modulation to successfully close an AO loop under varying atmospheric
turbulence conditions, at the expense of a loss in sensitivity. In this work we
train, analyse, and compare the use of deep neural networks (NNs) as non-linear
estimators for the non-modulated PyWFS, identifying the most suitable NN
architecture for reliable closed-loop AO. We develop a novel training strategy
for NNs that seeks to accommodate for changes in residual statistics between
open and closed-loop, plus the addition of noise for robustness purposes.
Through simulations, we test and compare several deep NNs, from classical to
new convolutional neural networks (CNNs), plus a state-of-the-art transformer
neural network (TNN, Global Context Visual Transformer, GCViT), first in
open-loop and then in closed-loop. Using open-loop simulated data, we observe
that a TNN (GCViT) largely surpasses any CNN in estimation accuracy in a wide
range of turbulence conditions. Also, the TNN performs better in simulated
closed-loop than CNNs, avoiding estimation issues at the pupil borders. When
closing the loop at strong turbulence and low noise, the TNN using
non-modulated PyWFS data is able to close the loop similar to a PyWFS with
12λ/D of modulation. When raising the noise only the TNN is able to
close the loop, while the standard linear reconstructor fails, even with
modulation. Using the GCViT, we close a real AO loop in the optical bench
achieving a Strehl ratio between 0.28 and 0.77 for turbulence conditions
ranging from 6cm to 20cm, respectively. In conclusion, we demonstrate that a
TNN is the most suitable architecture to extend the dynamic range without
sacrificing sensitivity for a non-modulated PyWFS. It opens the path for using
non-modulated Pyramid WFSs under an unprecedented range of atmospheric and
noise conditions.
更多查看译文
AI 理解论文
溯源树
样例
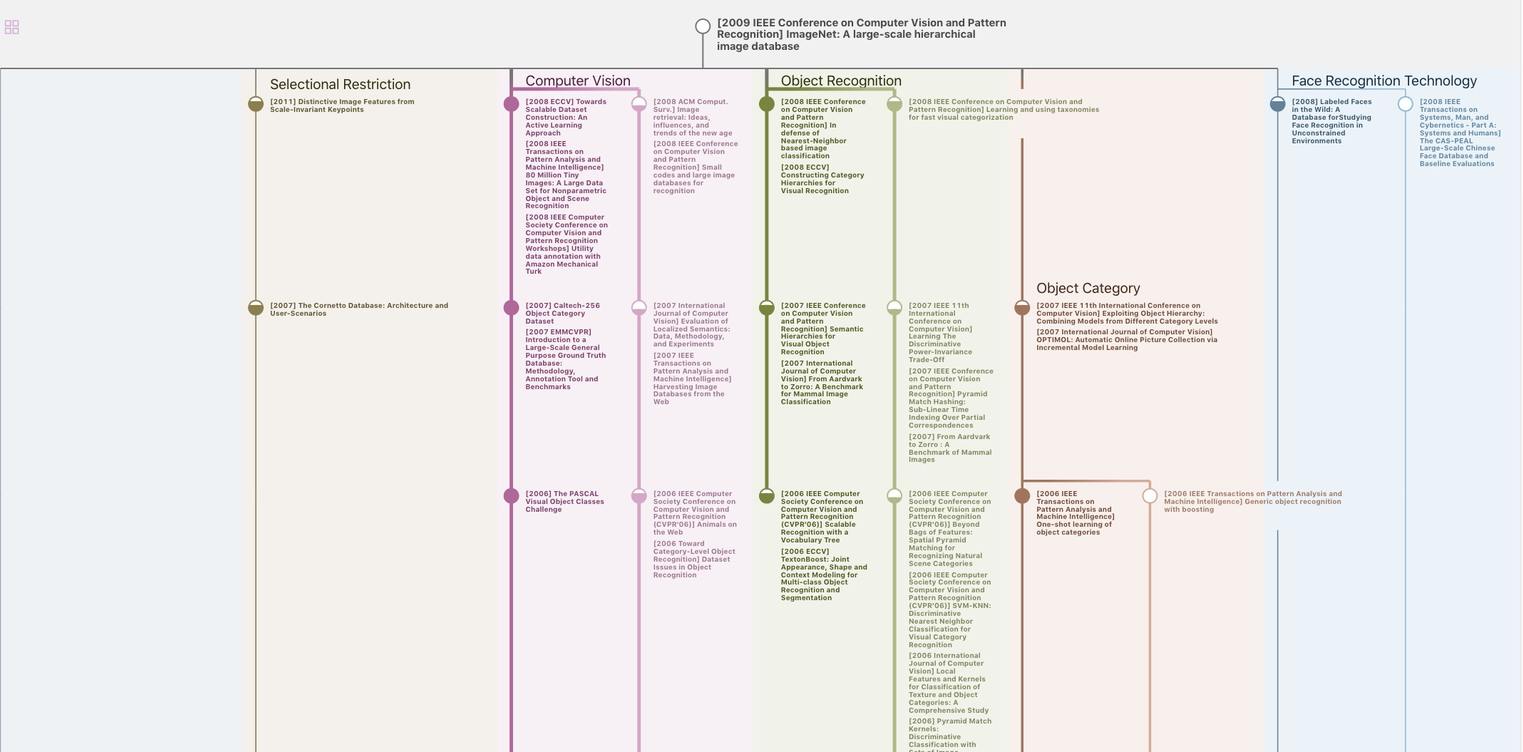
生成溯源树,研究论文发展脉络
Chat Paper
正在生成论文摘要