Strategic Feature Augmentation in TabNet for Unified Regional Wind Power Forecasting
2023 IEEE 7th Conference on Energy Internet and Energy System Integration (EI2)(2023)
Abstract
Accurate wind power forecasting plays a pivotal role in the effective integration of renewable energy into power systems. This paper proposes a unified TabNet model tailored to predict across multiple wind farms within a region, negating the need for individual wind farm-specific models, which can induce computational burdens and inconsistencies in prediction quality. Our innovation hinges on the integration of TabNet's sparse-max feature selector with our advanced feature augmentation approach. Specifically, we employ numerical weather predictions (NWP) and introduce a novel radix one-hot encoding for wind farm indexing, providing enhanced sparsity and capturing farm-specific traits. By extending NWP input from neighboring grid points, our model gains an augmented spatial and temporal perception field. The proposed GeoNWP-Index Hybrid model for WPF outperforms traditional techniques, reducing nRMSE and nMAE to 14.72% and 11.67%, respectively, from the benchmarks set by XGBoost at 16.46% and 13.55%. This approach under-scores deep learning's potential in revolutionizing regional WPF, pointing towards more cohesive renewable energy management.
MoreTranslated text
Key words
TabNet,Wind Power Forecasting,Feature Augmentation,Radix Encoding
AI Read Science
Must-Reading Tree
Example
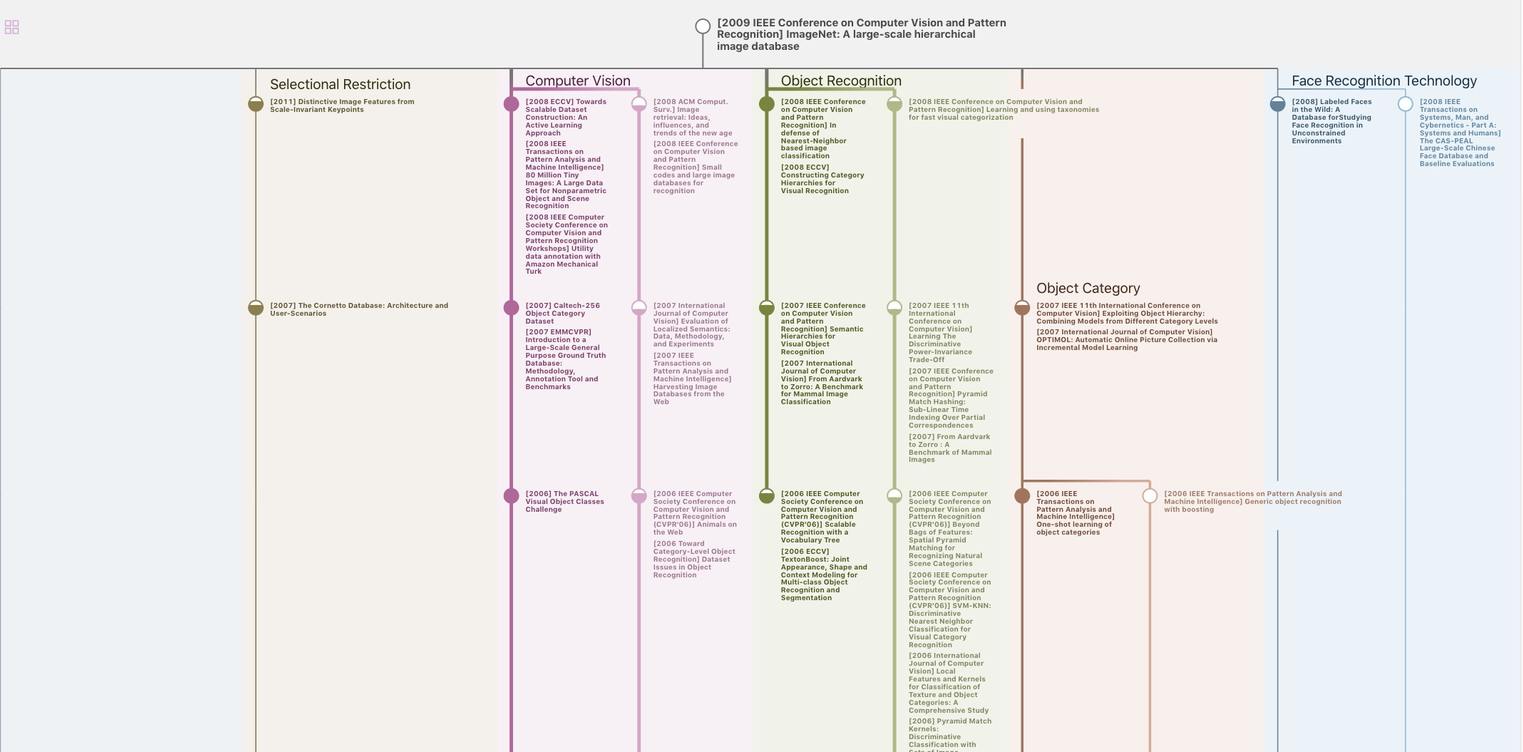
Generate MRT to find the research sequence of this paper
Chat Paper
Summary is being generated by the instructions you defined