Testing the predictive power of reverse screening to infer drug targets, with the help of machine learning
COMMUNICATIONS CHEMISTRY(2024)
Abstract
Estimating protein targets of compounds based on the similarity principle-similar molecules are likely to show comparable bioactivity-is a long-standing strategy in drug research. Having previously quantified this principle, we present here a large-scale evaluation of its predictive power for inferring macromolecular targets by reverse screening an unprecedented vast external test set of more than 300,000 active small molecules against another bioactivity set of more than 500,000 compounds. We show that machine-learning can predict the correct targets, with the highest probability among 2069 proteins, for more than 51% of the external molecules. The strong enrichment thus obtained demonstrates its usefulness in supporting phenotypic screens, polypharmacology, or repurposing. Moreover, we quantified the impact of the bioactivity knowledge available for proteins in terms of number and diversity of actives. Finally, we advise that developers of such approaches follow an application-oriented benchmarking strategy and use large, high-quality, non-overlapping datasets as provided here. Ligand-based reverse screening plays an important role in predicting molecular targets of bioactive compounds, however, its predictive ability might be overlooked due to the limitations of existing external test sets. Here, the authors assess the predictive power of reverse screening with a large diverse external bioactivity dataset.
MoreTranslated text
AI Read Science
Must-Reading Tree
Example
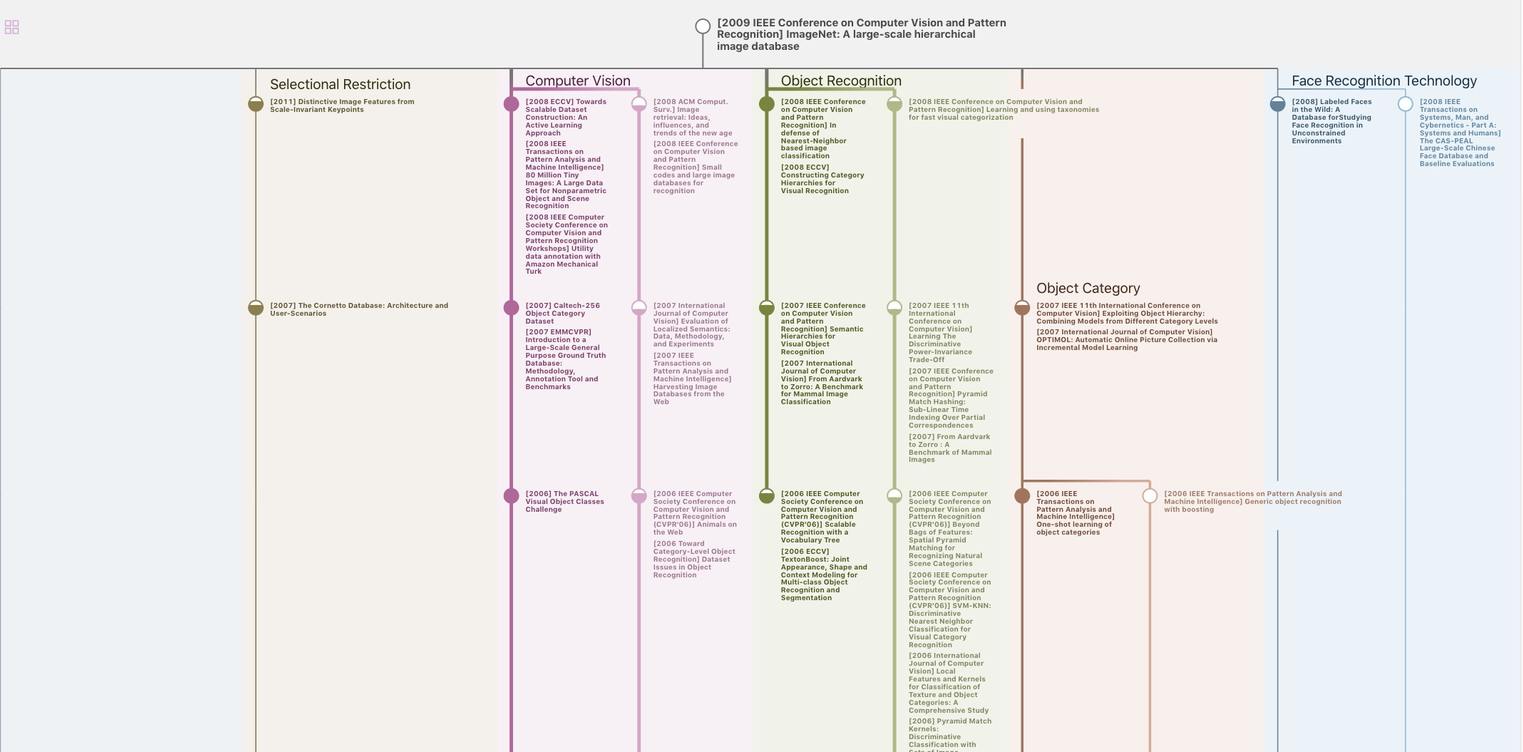
Generate MRT to find the research sequence of this paper
Chat Paper
Summary is being generated by the instructions you defined