Multi-scale iterative domain adaptation for specific emitter identification
Applied Intelligence(2024)
摘要
Specific emitter identification (SEI) is a technology that identifies different emitters through their unique characteristics. Research on traditional specific emitter identification systems focuses on general feature extraction. However, its effectiveness significantly decreases when faced with various influencing factors, such as time changes. Existing domain adaptation SEI methods also suffer from limitations such as the single domain scale and the poor performance against unpredictable changing factors. In this paper, we propose a multi-scale iterative domain adaptation (MSIDA) method for SEI based on domain adaptation, targeting the time variation factor specifically. The proposed method collects signals across different domain scales (seconds and days) and uses an improved domain adaptation method to align signals based on their domain characteristics and source properties at different scales, thereby eliminating the influence of the time factor. Given that the impact of certain factors such as time on SEI is unpredictable and irregular, our proposed method continuously tracks the influence of these factors through iterative domain adaptation, thereby improving the model’s generalization ability to these influencing factors. Furthermore, considering the difficulty of labeling data, our proposed flexible loss function can effectively mine the available information from both unlabeled and labeled data. Whether compared with traditional SEI methods or existing domain adaptation SEI methods, experimental results show that our proposed MSIDA method achieved at least a 6
更多查看译文
关键词
Deep learning,Specific emitter identification,Domain adaptation,Radio-frequency fingerprint
AI 理解论文
溯源树
样例
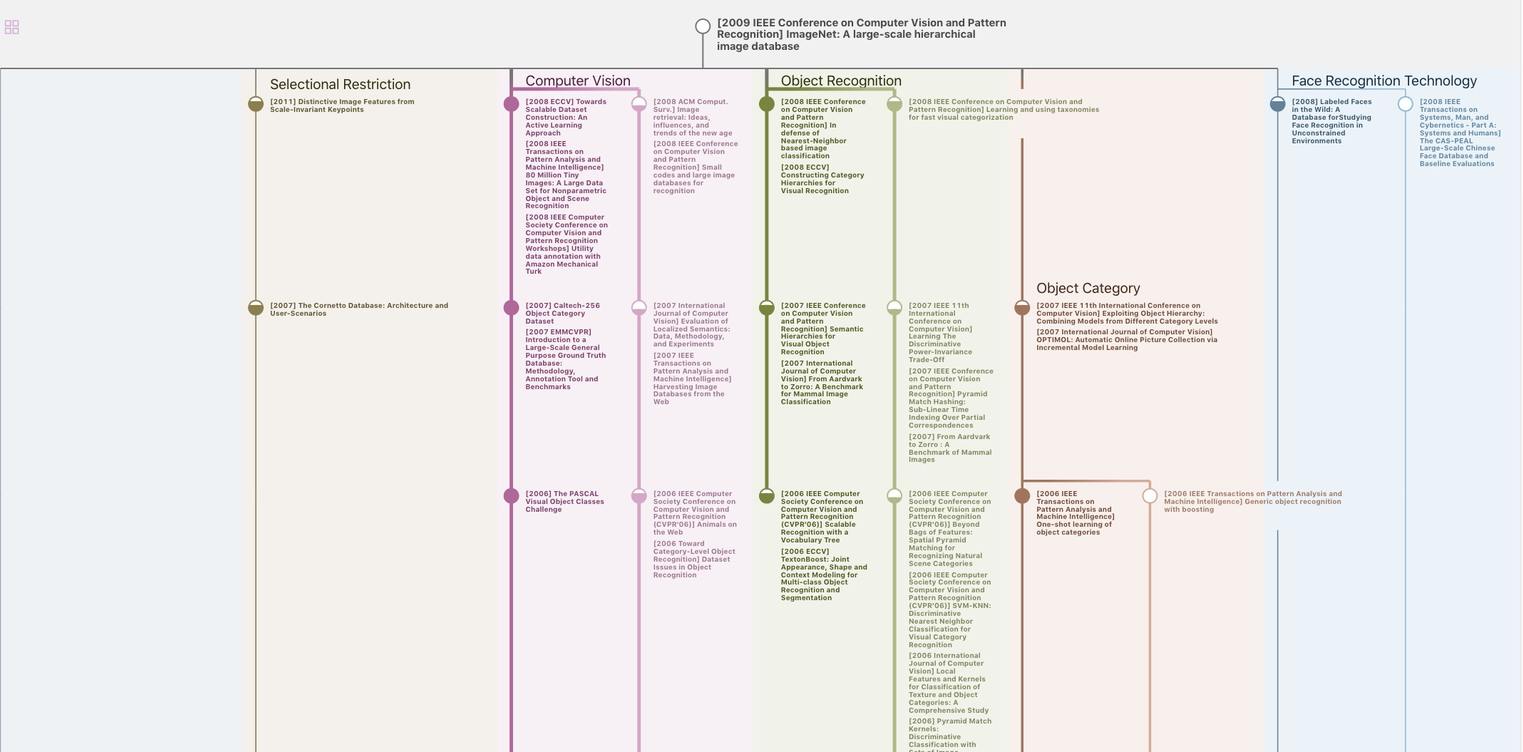
生成溯源树,研究论文发展脉络
Chat Paper
正在生成论文摘要