PU-KBS: A Robust Positive and Unlabeled Learning Framework With Key Band Selection for One-Class Hyperspectral Image Classification.
IEEE Trans. Geosci. Remote. Sens.(2024)
摘要
Positive and unlabeled (PU) learning is aimed at building a binary classifier to distinguish target from background using only the known positive samples, which is an advanced solution for the hyperspectral target detection task. However, when PU learning meets complex hyperspectral scenarios, there are two main challenges: 1) How to estimate the class prior accurately. The class prior, i.e., the target proportion, is an important prior for PU learning to learn the discriminant boundary, but it is difficult to estimate in hyperspectral imagery, due to the inter-class spectral similarity. 2) How to remove redundancy and improve the discriminative features of the target. The diagnostic spectral feature extraction is important for the weakly supervised PU learning models as it can help with separating target from background. In this paper, to tackle these challenges, a robust PU learning framework with key band selection (PU-KBS) is proposed, which is modeled as an end-to-end and class prior free PU learning framework, where the accurate class prior and the most discriminative key band subset are jointly initialized and iteratively updated until reaching the optimal result by evolutionary search. Meanwhile, a deep PU learning detector is introduced for guiding the subsequent search direction and discriminative deep feature extraction. The proposed PU-KBS framework was verified using different hyperspectral datasets, where accurate class prior estimation, diagnostic spectral characteristics, and robust detection results could be obtained simultaneously by the PU-KBS framework. Furthermore, the improvement in band selection interpretability and detection performance was proven experimentally.
更多查看译文
关键词
One-class classification,hyperspectral image,positive and unlabeled learning
AI 理解论文
溯源树
样例
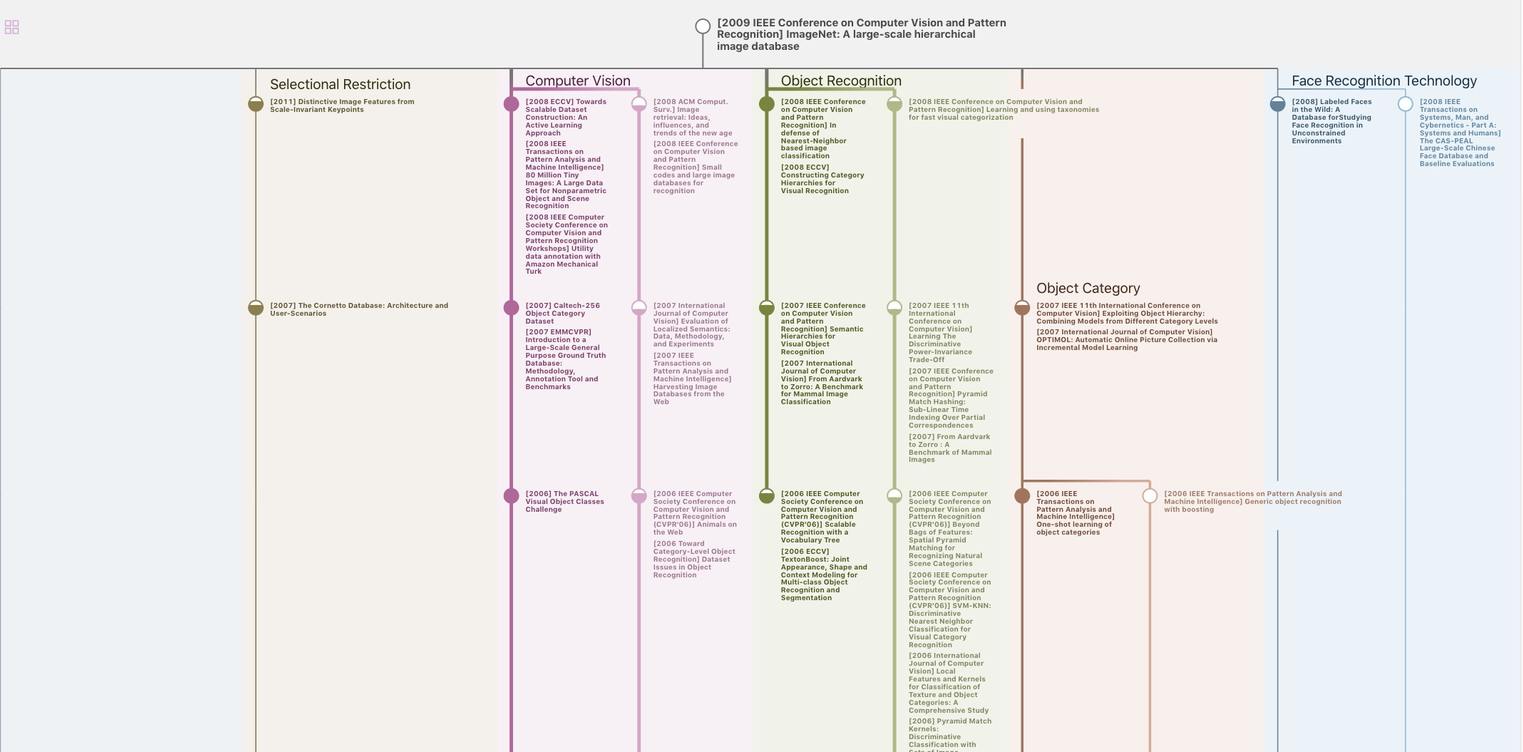
生成溯源树,研究论文发展脉络
Chat Paper
正在生成论文摘要