Data-driven discovery of carbonyl organic electrode molecules: machine learning and experiment
JOURNAL OF MATERIALS CHEMISTRY A(2024)
摘要
Carbonyl organic electrode molecules have broad prospects for application in lithium-ion batteries due to their environmental friendliness and cost-effective merit. To overcome the drawbacks associated with traditional time-consuming and costly trial and error experiments, herein, high-throughput calculations and machine learning methods have been employed to accelerate the development of high-performance carbonyl organic electrode molecules by evaluating one million molecules. Hierarchical clustering has been introduced into the selection process to find those target molecules and help us eliminate non-ring molecules. As the reduction potential is a crucial factor in evaluating the performance of electrode materials, based on the created dataset of organic electrode molecules by high-throughput calculations, we have built a machine learning model whose coefficient of determination can reach 0.88 for predicting the reduction potential. With the above efforts, naphthalene-1,4,5,8-tetraone with high reduction potential and energy density has been screened out and indeed exhibits a long cycle life of 2500 cycles at 1 A g-1 and a high discharge voltage of 2.5 V. The approach developed in this work offers new insight to filter advanced organic electrode molecules accurately and rapidly for Li-ion batteries. In this work, a universal strategy for the identification of high-performance OEMs for LIBs has been illustrated. The predicted molecule, naphthalene-1,4,5,8-tetraone, exhibits excellent electrochemical performance in terms of capacity and lifetime.
更多查看译文
AI 理解论文
溯源树
样例
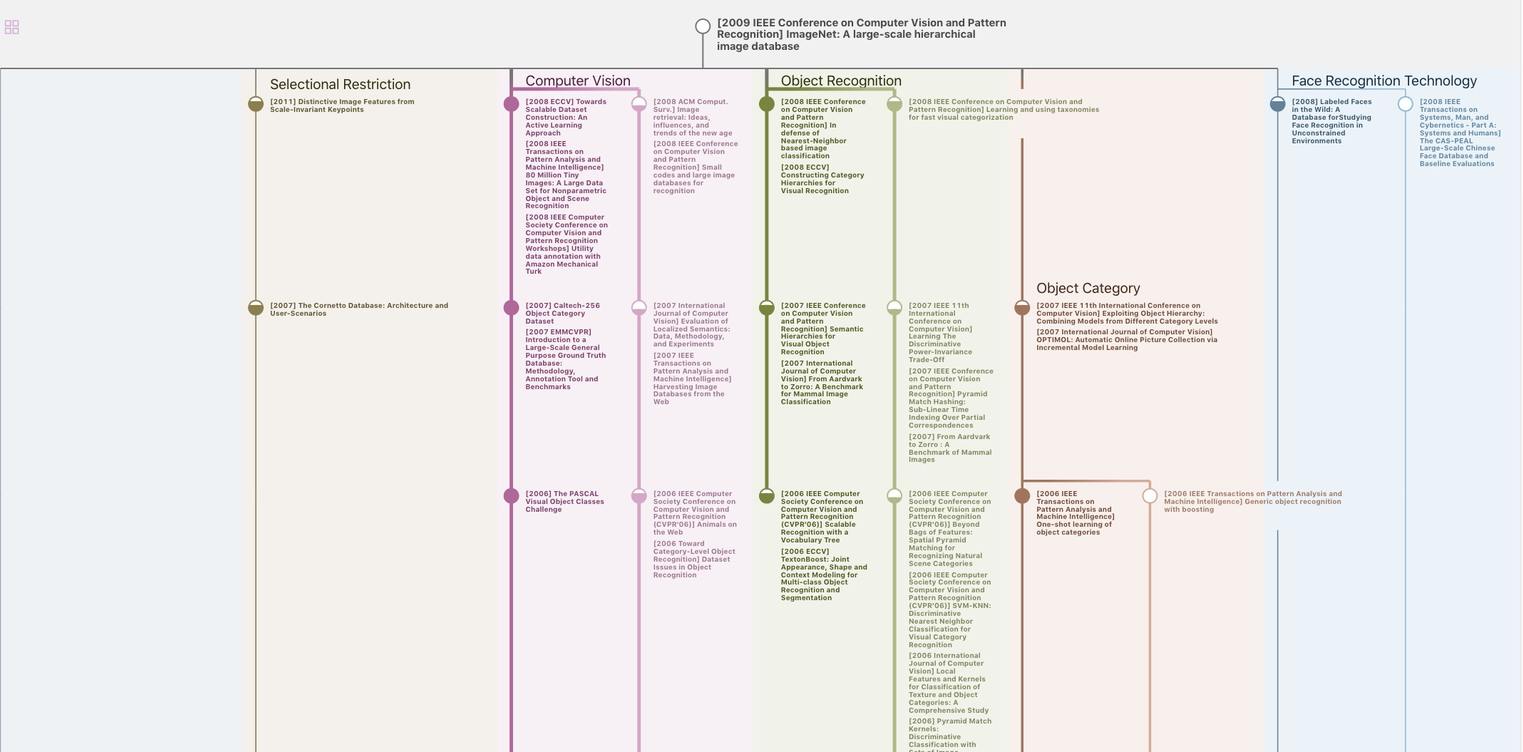
生成溯源树,研究论文发展脉络
Chat Paper
正在生成论文摘要