Causally Debiased Time-aware Recommendation
WWW '24 Proceedings of the ACM on Web Conference 2024(2024)
摘要
Time-aware recommendation has been widely studied for modeling the user dynamic preference and a lot of models have been proposed. However, these models often overlook the fact that users may not behave evenly on the timeline, and observed datasets can be biased by user intrinsic preferences or previous recommender systems, leading to degraded model performance. We propose a causally debiased time-aware recommender framework to accurately learn user preference. We formulate the task of time-aware recommendation by a causal graph, identifying two types of biases on the item and time levels. To optimize the ideal unbiased learning objective, we propose a debiased framework based on the inverse propensity score (IPS) and extend it to the doubly robust method. Considering that the user preference can be diverse and complex, which may result in unmeasured confounders, we develop a sensitivity analysis method to obtain more accurate IPS. We theoretically draw a connection between the proposed method and the ideal learning objective, which to the best of our knowledge, is the first time in the research community. We conduct extensive experiments on three real-world datasets to demonstrate the effectiveness of our model. To promote this research direction, we have released our project at https://paitesanshi.github.io/CDTR/.
更多查看译文
AI 理解论文
溯源树
样例
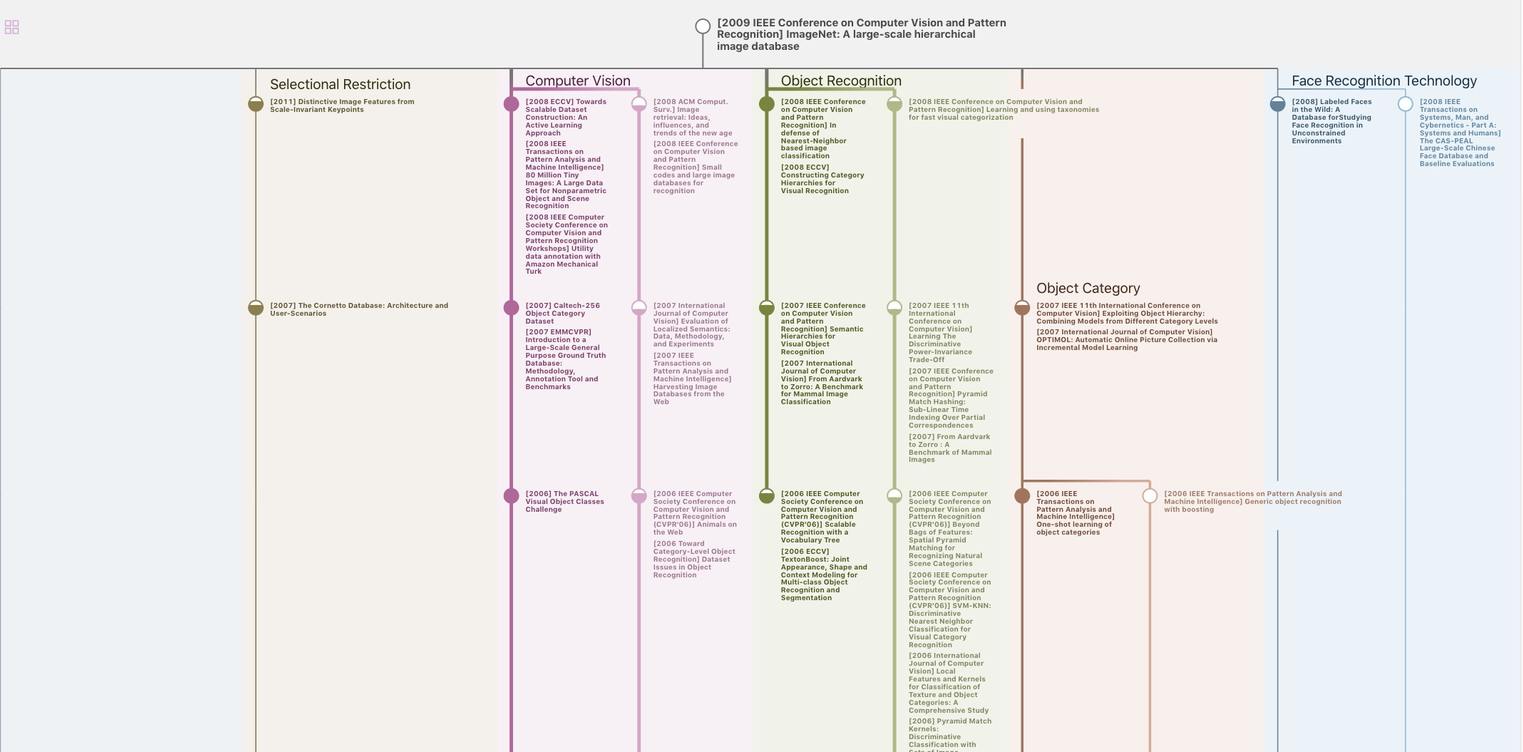
生成溯源树,研究论文发展脉络
Chat Paper
正在生成论文摘要