EEG-Based Classification of Cognitive Load and Task Conditions for AR Supported Construction Assembly: A Deep Learning Approach
COMPUTING IN CIVIL ENGINEERING 2023-VISUALIZATION, INFORMATION MODELING, AND SIMULATION(2024)
摘要
Cognitive load is a metric that reflects construction workers' mental effort during task performance for perceiving information and stimuli from the working environment. Potential cognitive impacts of human-system interaction must be evaluated carefully, specifically when proposing innovative display methods to improve traditional construction assembly patterns. However, identifying cognitive load during task performance is difficult because of the lack of directly observable features. This research proposed a deep learning classification of cognitive load and task conditions to address this problem based on an electroencephalogram (EEG)-centered physiological measurement. The study recruited 30 subjects to assemble medium-sized wood frame walls with an augmented reality (AR) conformal model. EEG was used as a neuroimaging technique to collect subjects' brain rhythm signals. The task conditions (relax and observe, and assemble) were assigned to represent two different levels of cognitive load. The one-dimensional convolutional neural network-long short-term memory (1D CNN-LSTM) was deployed to classify the subtasks based on the EEG signal. Additionally, comparison tests for performance evaluation with other deep learning networks (1D CNN and LSTM) were conducted. The outcomes validated the usability of the proposed approach for identifying different task conditions with cognitive loads, which provides a prediction accuracy of 93.60%. Moreover, it suggested a promising application of workers' activity recognition based on the EEG signal classification.
更多查看译文
AI 理解论文
溯源树
样例
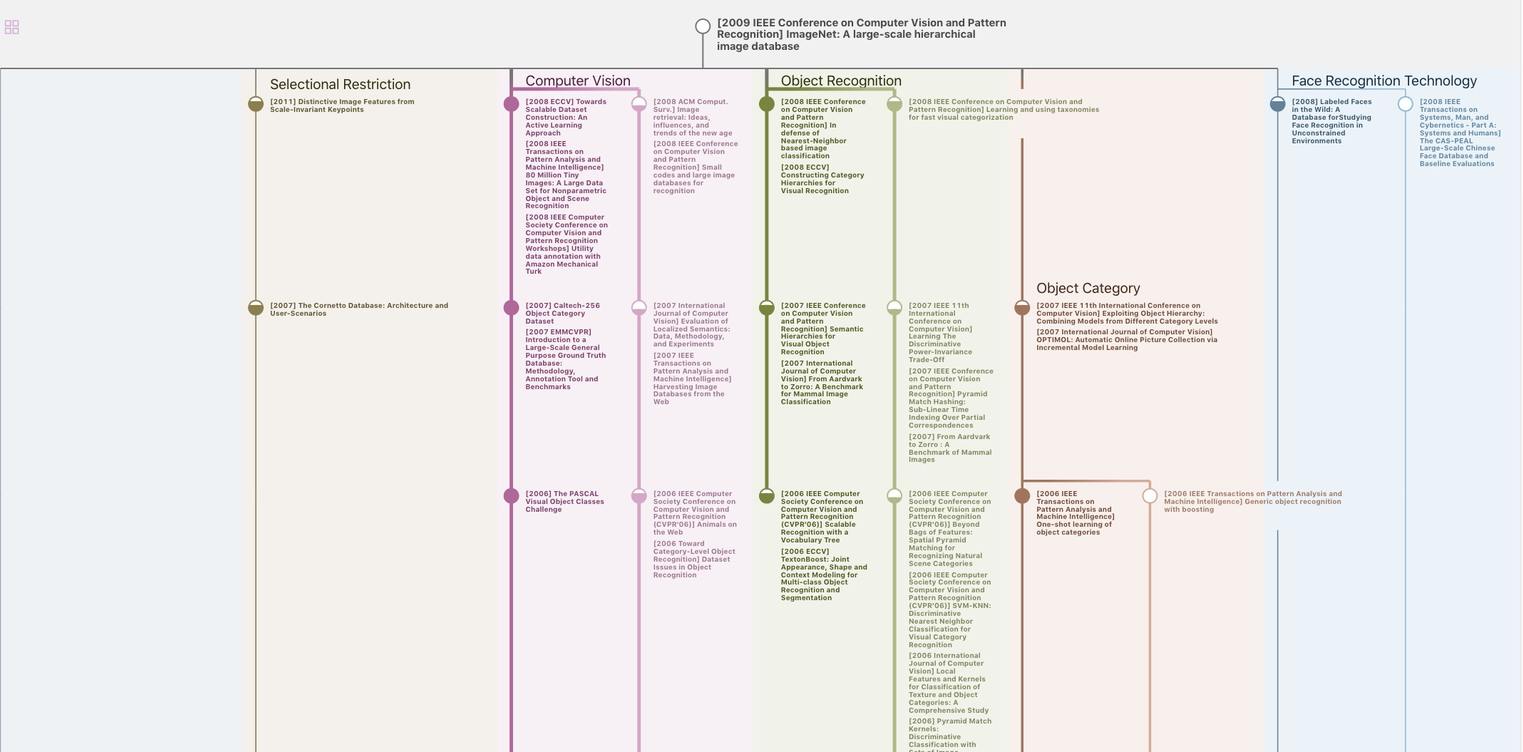
生成溯源树,研究论文发展脉络
Chat Paper
正在生成论文摘要