Are Supervised Learning Methods Suitable for Estimating Crop Water Consumption under Optimal and Deficit Irrigation?
AGRONOMY-BASEL(2024)
Abstract
This study examined the performance of random forest (RF), support vector machine (SVM) and adaptive boosting (AB) machine learning models used to estimate daily potato crop evapotranspiration adjusted (ETc-adj) under full irrigation (I100), 50% of full irrigation supply (I50) and rainfed cultivation (I0). Five scenarios of weather, crop and soil data availability were considered: (S1) reference evapotranspiration and precipitation, (S2) S1 and crop coefficient, (S3) S2, the fraction of total available water and root depth, (S4) S2 and total soil available water, and (S5) S3 and total soil available water. The performance of machine learning models was compared with the standard FAO56 calculation procedure. The most accurate ETc-adj estimates were observed with AB4 for I100, RF3 for I50 and AB5 for I0 with coefficients of determination (R2) of 0.992, 0.816 and 0.922, slopes of 1.004, 0.999 and 0.972, modelling efficiencies (EF) of 0.992, 0.815 and 0.917, mean absolute errors (MAE) of 0.125, 0.405 and 0.241 mm day-1, root mean square errors (RMSE) of 0.171, 0.579 and 0.359 mm day-1 and mean squared errors (MSE) of 0.029, 0.335 and 0.129 mm day-1, respectively. The AB model is suggested for ETc-adj prediction under I100 and I0 conditions, while the RF model is recommended under the I50 condition.
MoreTranslated text
Key words
irrigation,water stress,random forest,support vector machine,adaptive boosting,machine learning
AI Read Science
Must-Reading Tree
Example
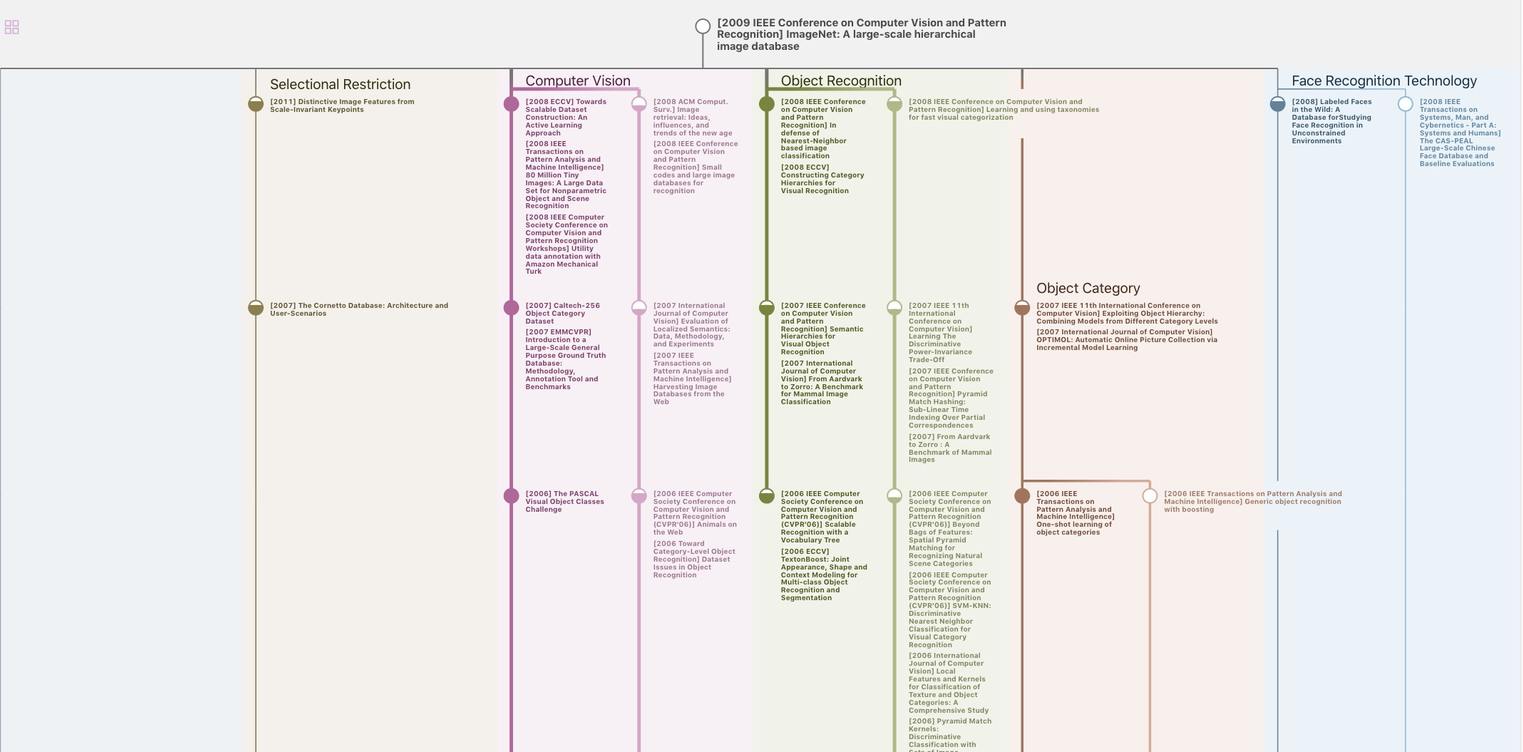
Generate MRT to find the research sequence of this paper
Chat Paper
Summary is being generated by the instructions you defined