Uncertainty-Aware Physical Simulation of Neural Radiance Fields for Fluids
CMES-COMPUTER MODELING IN ENGINEERING & SCIENCES(2024)
Abstract
This paper presents a novel framework aimed at quantifying uncertainties associated with the 3D reconstruction of smoke from 2D images. This approach reconstructs color and density fields from 2D images using Neural Radiance Field (NeRF) and improves image quality using frequency regularization. The NeRF model is obtained via joint training of multiple artificial neural networks, whereby the expectation and standard deviation of density fields and RGB values can be evaluated for each pixel. In addition, customized physics-informed neural network (PINN) with residual blocks and two-layer activation functions are utilized to input the density fields of the NeRF into NavierStokes equations and convection-diffusion equations to reconstruct the velocity field. The velocity uncertainties are also evaluated through ensemble learning. The effectiveness of the proposed algorithm is demonstrated through numerical examples. The present method is an important step towards downstream tasks such as reliability analysis and robust optimization in engineering design.
MoreTranslated text
Key words
Uncertainty quantification,neural radiance field,physics-informed neural network,frequency regularization,two,layer activation function,ensemble learning
AI Read Science
Must-Reading Tree
Example
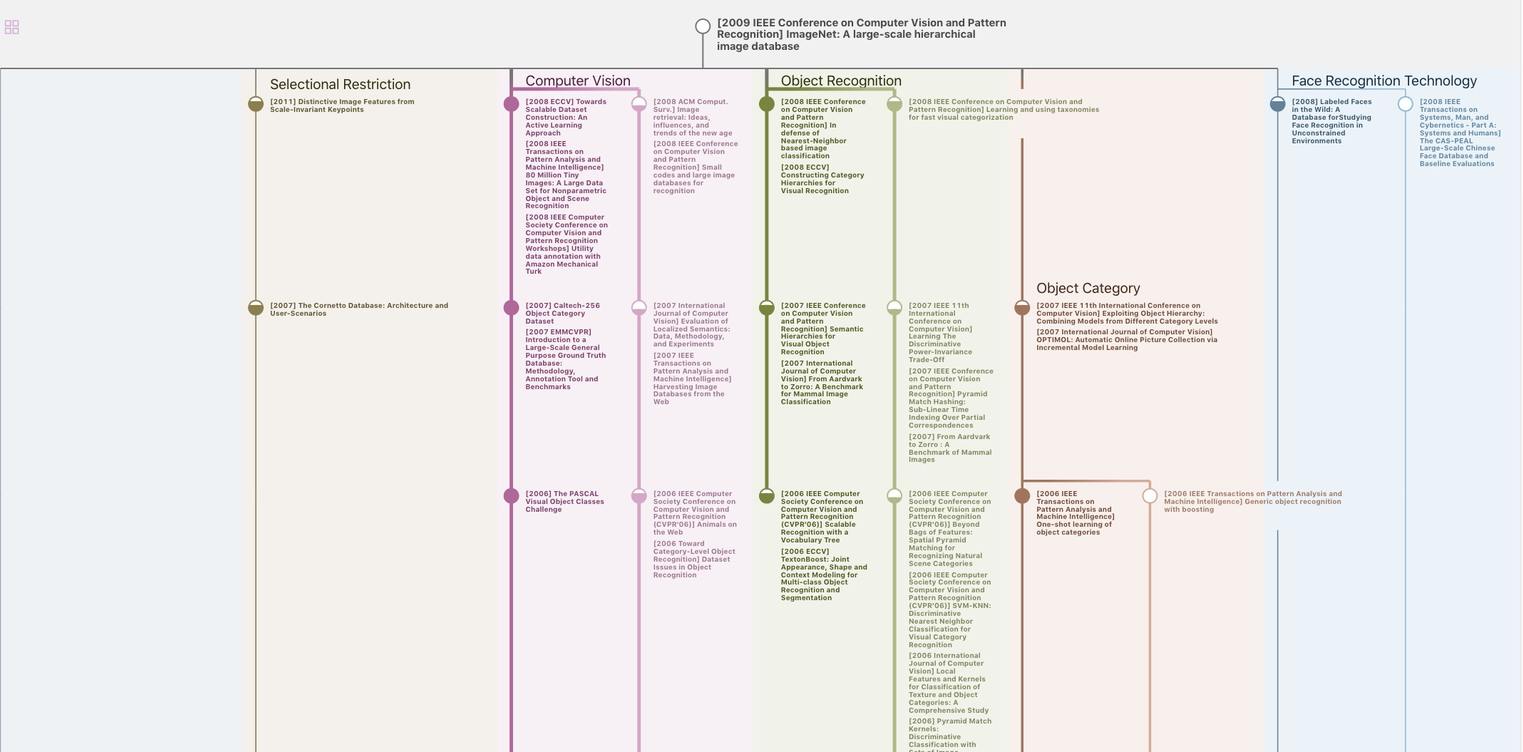
Generate MRT to find the research sequence of this paper
Chat Paper
Summary is being generated by the instructions you defined