A multifeature fusion model for surface roughness measurement of cold-rolled strip steel based on laser speckle
MEASUREMENT(2024)
摘要
The online measurement of the surface roughness of cold-rolled strip steel plays a significant role in the steel manufacturing process. However, the traditional mechanism method based on laser speckle is not sufficient for image processing, and the precision of online measurement is not high. Therefore, this paper proposes a multifeature fusion model for the surface roughness of cold-rolled strip steel to improve the measurement efficiency of existing speckle methods. Cold-rolled strip steel is irradiated by a laser beam to produce speckle images. Statistical features are extracted using a gray-level cooccurrence matrix (GLCM). The deep information of an image is extracted using convolutional neural networks (CNN) and a convolutional block attention mechanism module (CBAM). The features extracted from the GLCM and CNN-CBAM methods are combined to create a multifeature fusion dataset. The fused features are predicted by a support vector regression (SVR) algorithm. Then, the SVR model is compared with three machine learning prediction models: classification and regression tree (CART), random forest (RF), and k-nearest neighbor (KNN). Experimental results confirm that the proposed multifeature fusion model predicts surface roughness with a minimum mean square error of 0.237%.
更多查看译文
关键词
Surface roughness,Multifeature fusion,Laser speckle,Convolution block attention mechanism,module,Support vector machine
AI 理解论文
溯源树
样例
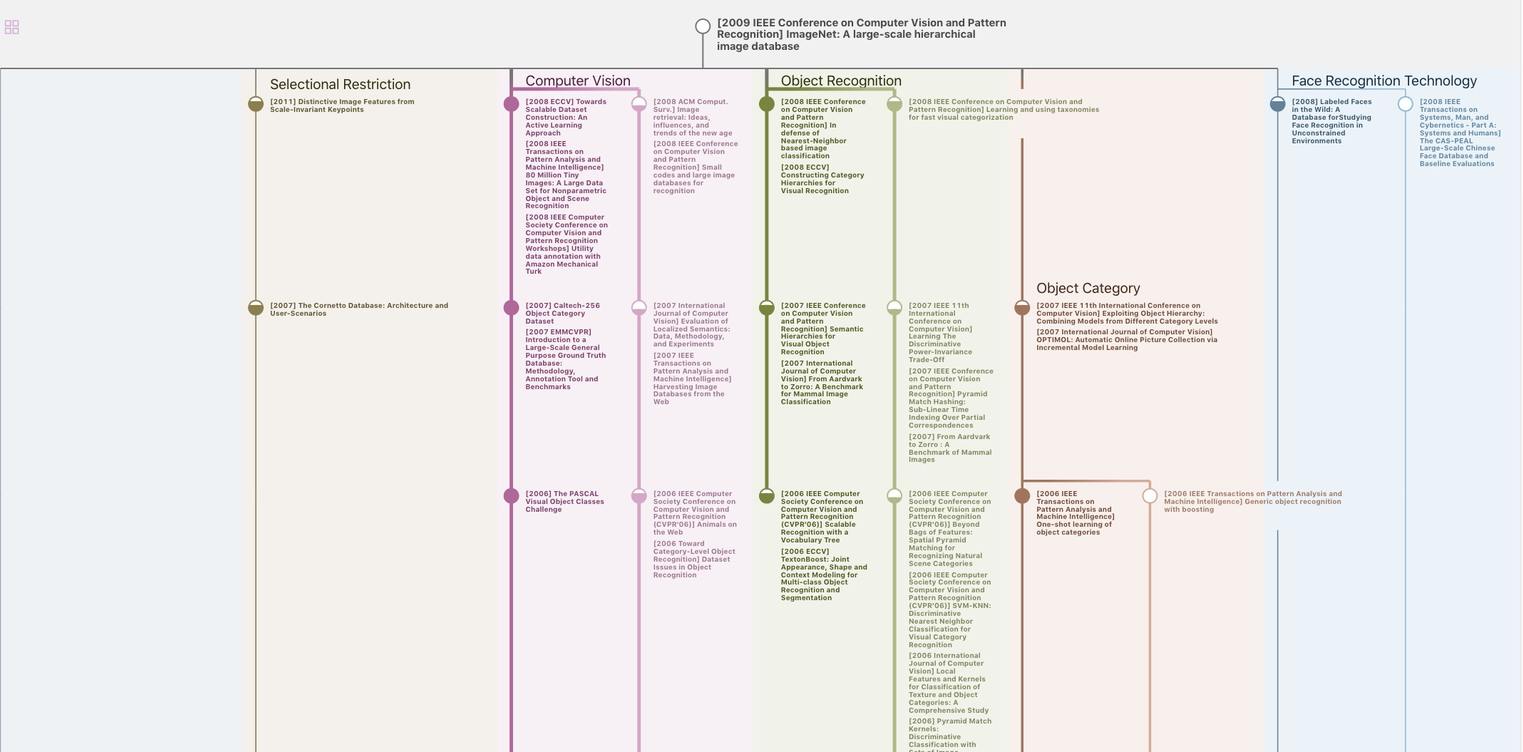
生成溯源树,研究论文发展脉络
Chat Paper
正在生成论文摘要