Geometry design for gravimetry and magnetometry surveys using deep learning
BOLETIN DE GEOLOGIA(2024)
Abstract
In geophysical exploration, terrain analysis is fundamental for planning the gravity and magnetic surveys. However, current analyses necessitate supervised use of extensive secondary information. Consequently, this study proposes a methodology that leverages deep learning to assess the impact of vegetation coverage and topography in geophysical acquisition. An artificial neural network multilayer perceptron was used to consider five terrain -related variables, while a convolutional neural network is used for automated classification of vegetation cover on satellite imagery. From this, geophysical acquisition points are obtained, adhering to criteria involving accessibility favourability, distances, restrictions imposed by bodies of water, and forested cover. The obtained geometry was tested in the exploration of the Granito de Durania in Colombia, and the results were analysed using acquisition transects and computational modelling of gravity and magnetic anomalies. Interpolation techniques were applied, with the Inverse Distance Weighting (IDW) method yielding the most informative map for interpreting the delineation of the intrusive body.
MoreTranslated text
Key words
Deep learning,Geophysical exploration,Forward modeling,Acquisition design
AI Read Science
Must-Reading Tree
Example
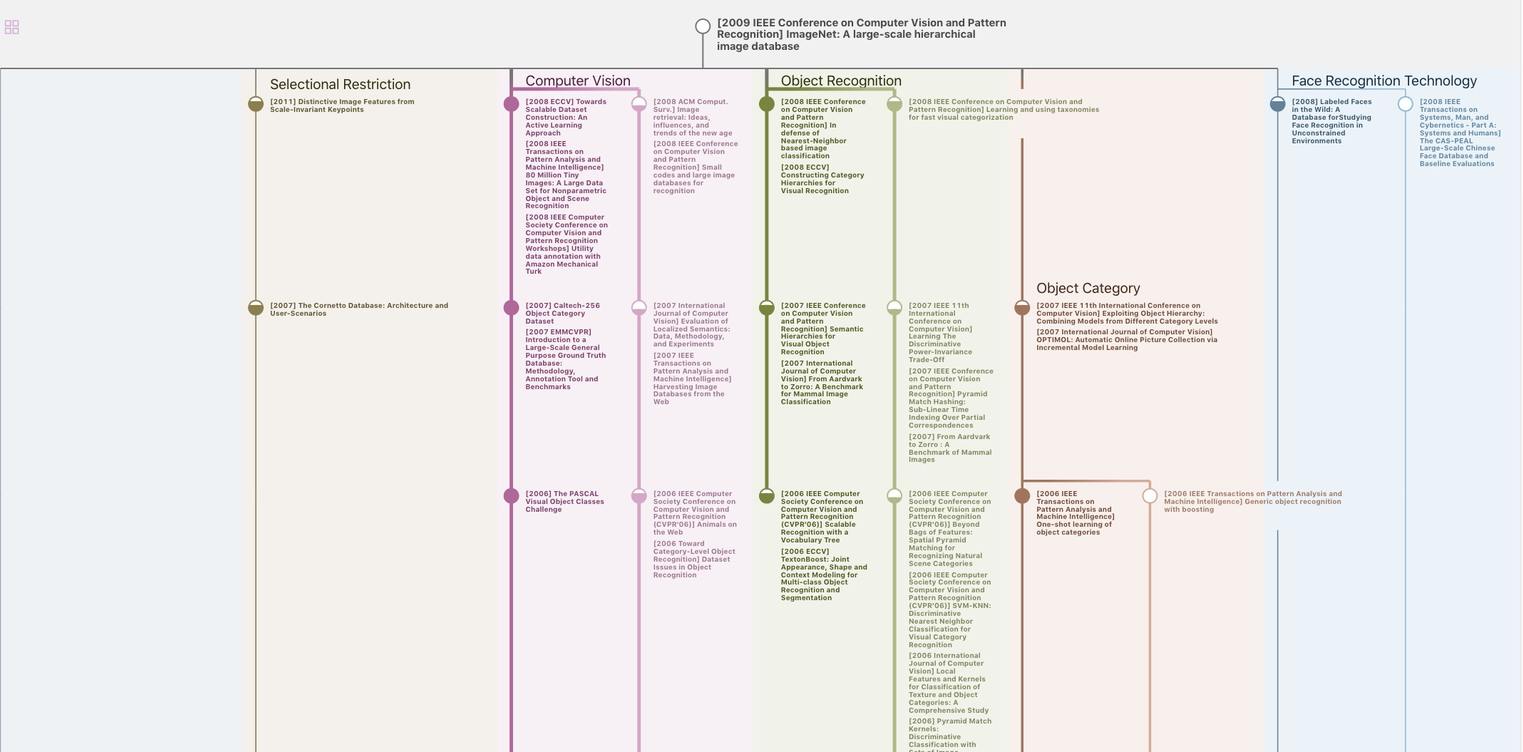
Generate MRT to find the research sequence of this paper
Chat Paper
Summary is being generated by the instructions you defined