Reducing Confounding Bias in Nonexperimental Evaluation: An Application of Empirical Bayes Residuals
JOURNAL OF THE SOCIETY FOR SOCIAL WORK AND RESEARCH(2024)
摘要
Objective: In social service settings, client exposure to an intervention is often facilitated by workers (e.g., case managers, care coordinators) who have some discretion over the services their clients receive. This paper demonstrates how the empirical Bayes residuals (EBRs) of predicted random effects can reliably estimate these workers' latent decision-making tendencies, such as the tendency to refer clients to a particular intervention. As such, in nonexperimental evaluation studies, the EBR can provide a measured value for an unobserved assignment determinant, which can be included in treatment effect models to reduce confounding bias. Method: We used Monte Carlo simulation to generate data for a hypothetical evaluation scenario in which child welfare caseworkers nonrandomly referred youth to a behavioral health intervention. Model variations assessed for bias in treatment effect estimates when instrumenting for nonrandom assignment with the EBR. Results: When caseworker referral effects were evident, the EBR consistently performed well as an instrument to obtain unbiased treatment effects and generally outperformed other methods, such as partially adjusted regression and instrumenting with simple caseworker means. Conclusions: The EBR offers a useful measurement tool when estimating causal effects from nonexperimental data. Study conditions that do not support instrumental variable estimation are also considered.
更多查看译文
关键词
program evaluation,causal inference,quasi-experimental,empirical Bayes,random effects
AI 理解论文
溯源树
样例
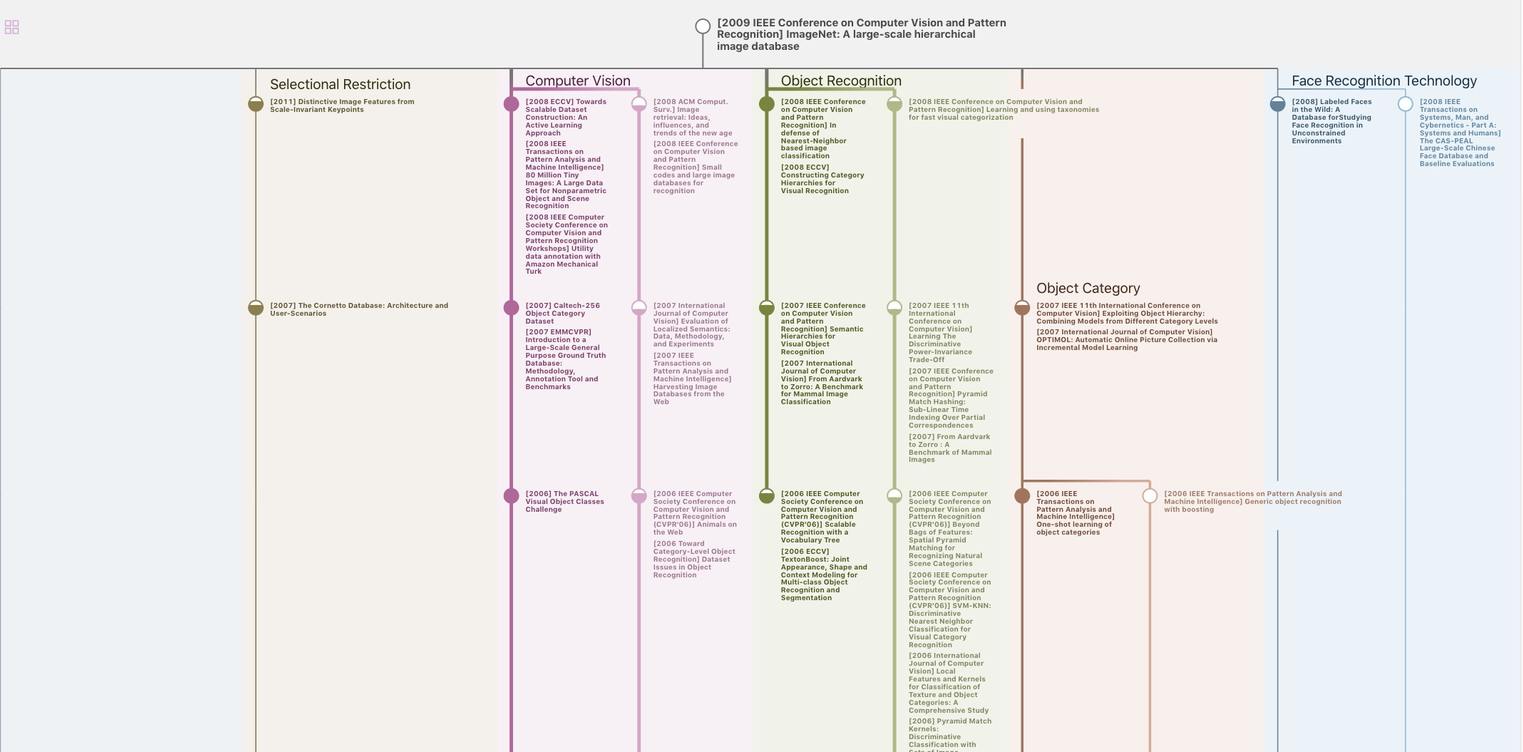
生成溯源树,研究论文发展脉络
Chat Paper
正在生成论文摘要