Fault-Tolerant Control for Multi-UAV Exploration System via Reinforcement Learning Algorithm
AEROSPACE(2024)
摘要
In the UAV swarm, the degradation in the health status of some UAVs often brings negative effects to the system. To compensate for the negative effect, we present a fault-tolerant Multi-Agent Reinforcement Learning Algorithm that can control an unstable Multiple Unmanned Aerial Vehicle (Multi-UAV) system to perform exploration tasks. Different from traditional multi-agent methods that require the agents to remain healthy during task execution, our approach breaks this limitation and allows the agents to change status during the task. In our algorithm, the agent can accept both the adjacency state matrix about the neighboring agents and a kind of healthy status vector to integrate both and generate the communication topology. During this process, the agents with poor health status are given more attention for returning to normal status. In addition, we integrate a temporal convolution module into our algorithm and enable the agent to capture the temporal information during the task. We introduce a scenario regarding Multi-UAV ground exploration, where the health status of UAVs gradually weakens over time before dropping into a fault status; the UAVs require rescues from time to time. We conduct some experiments in this scenario and verify our algorithm. Our algorithm can increase the drone's survival rate and make the swarm perform better.
更多查看译文
关键词
machine learning,swarm intelligence,UAV exploration,multi-agent system
AI 理解论文
溯源树
样例
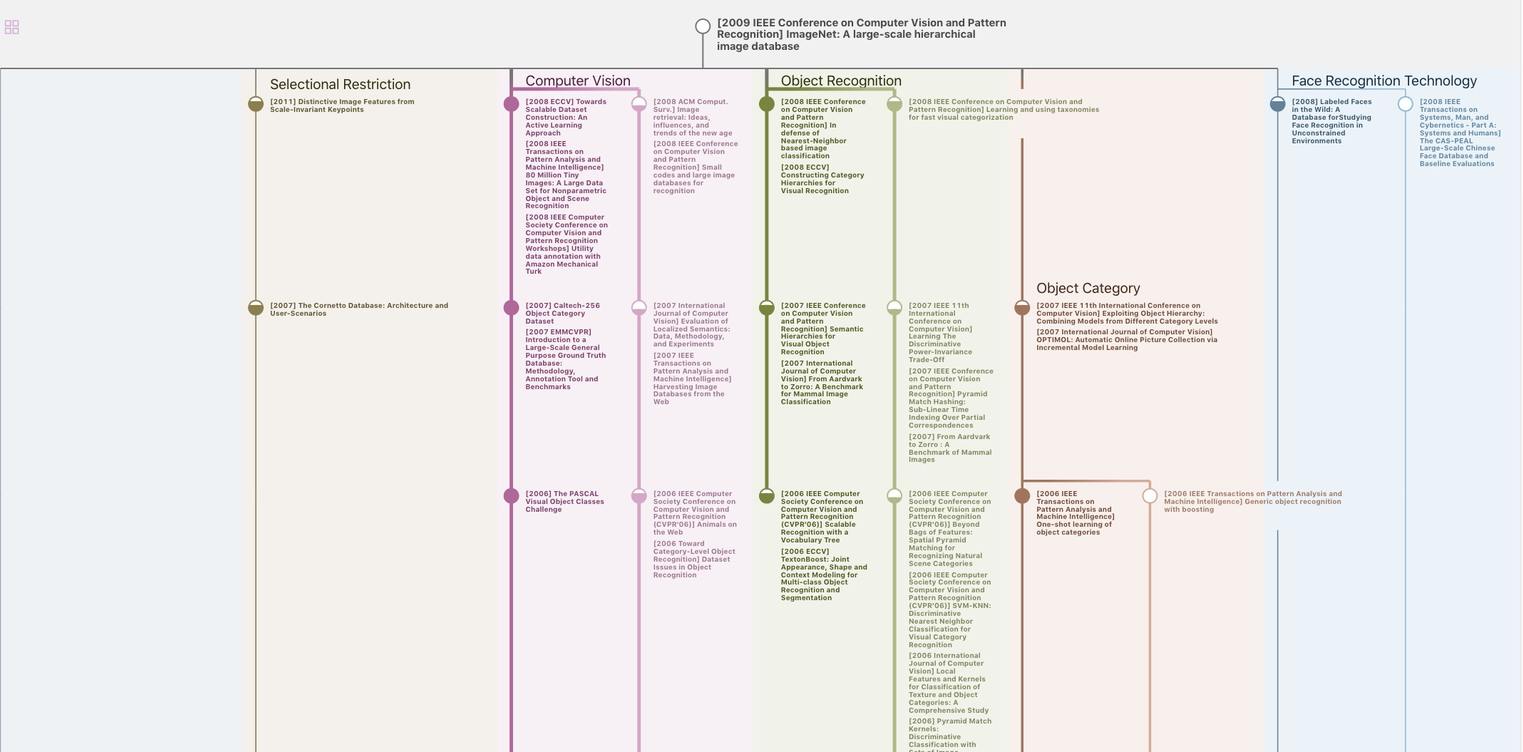
生成溯源树,研究论文发展脉络
Chat Paper
正在生成论文摘要