A reinforcement learning approach for adaptive tracking control of a reusable rocket model in a landing scenario
NEUROCOMPUTING(2024)
摘要
This work explores the application of reinforcement learning to control the descend/landing phase of a reusable rocket model, where the rocket must track a nominal/planar reference path with adequate attitude. To tackle the control problem, which is multivariable, a cascade control architecture is proposed, consisting of three single -input single -output controllers. The inner control loop is responsible for controlling the attitude using a reference for attitude provided by the outer loop and the thrust vectoring, where the gimbal angle is the manipulated variable. The position error is handled by the external loop, that handles the altitude error, used to adjust thrust. Several configurations, using Linear Quadratic Gaussian (LQG) control (LQ regulator with a Kalman observer), PID control and adaptive control based on Reinforcement Learning (optimal control with learning capability based on a linear regression model), are evaluated and compared.
更多查看译文
关键词
Reinforcement learning,GNC,Reentry rocket,Attitude control,Trajectory control
AI 理解论文
溯源树
样例
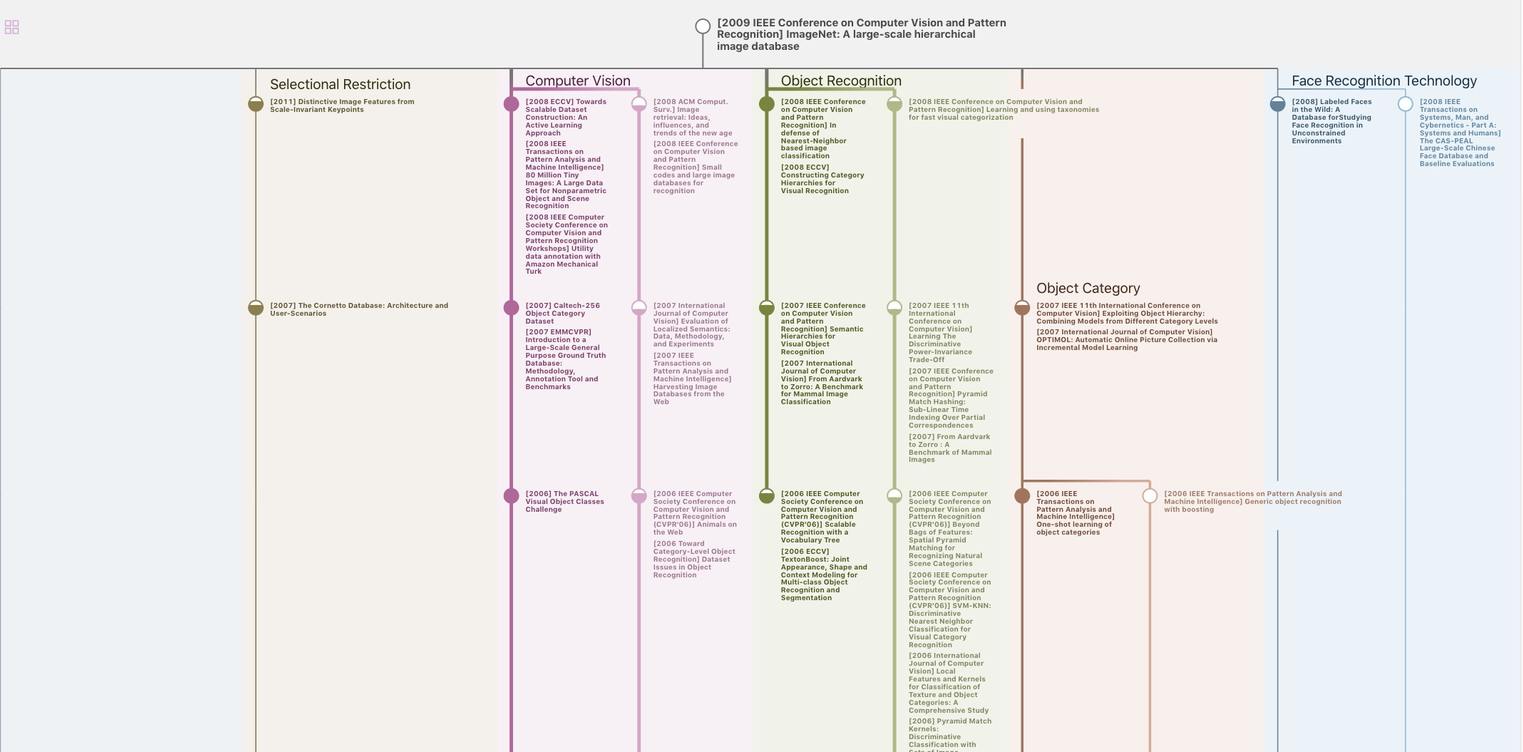
生成溯源树,研究论文发展脉络
Chat Paper
正在生成论文摘要