A long short term memory network-based, global navigation satellite system/inertial navigation system for unmanned surface vessels
JOURNAL OF MARINE ENGINEERING AND TECHNOLOGY(2024)
Abstract
In recent years, research on unmanned surface vessels has entered a new phase, demanding the increased accuracy and reliability of integrated navigation and positioning systems. However, in complex environments, due to intermittent sensor failures or communication limitations, Global Navigation Satellite System (GNSS) signals are unreliable. Various errors that emerge in the system make it impossible to complete the measurement update process of filtering algorithms. This study provides a mechanism for improving the accuracy of combined GNSS/Shipborne Inertial Navigation System (SINS) integrated navigation when a GNSS outage occurs. Firstly, a SINS denoising method combining Complementary Ensemble Empirical Mode Decomposition (CEEMD) and an improved wavelet threshold algorithm is proposed. Secondly, a method based on Long Short-Term Memory (LSTM) network assisted GNSS/SINS integrated navigation is proposed to address the issue of poor information fusion performance when GNSS outages occur. The trained LSTM model is combined with SINS data to predict velocity and position during GNSS outages, providing high-precision velocity and position information. This ensures continuous, high-precision, navigation of unmanned surface vessels. Finally, to confirm the validity of the proposed methodology, a sea trial is performed to compare the different methods, and the results reveal the feasibility and effectiveness of the proposed methodology.
MoreTranslated text
Key words
Unmanned surface vessels,machine learning,long short term memory network,integrated navigation system,experimental evaluation
AI Read Science
Must-Reading Tree
Example
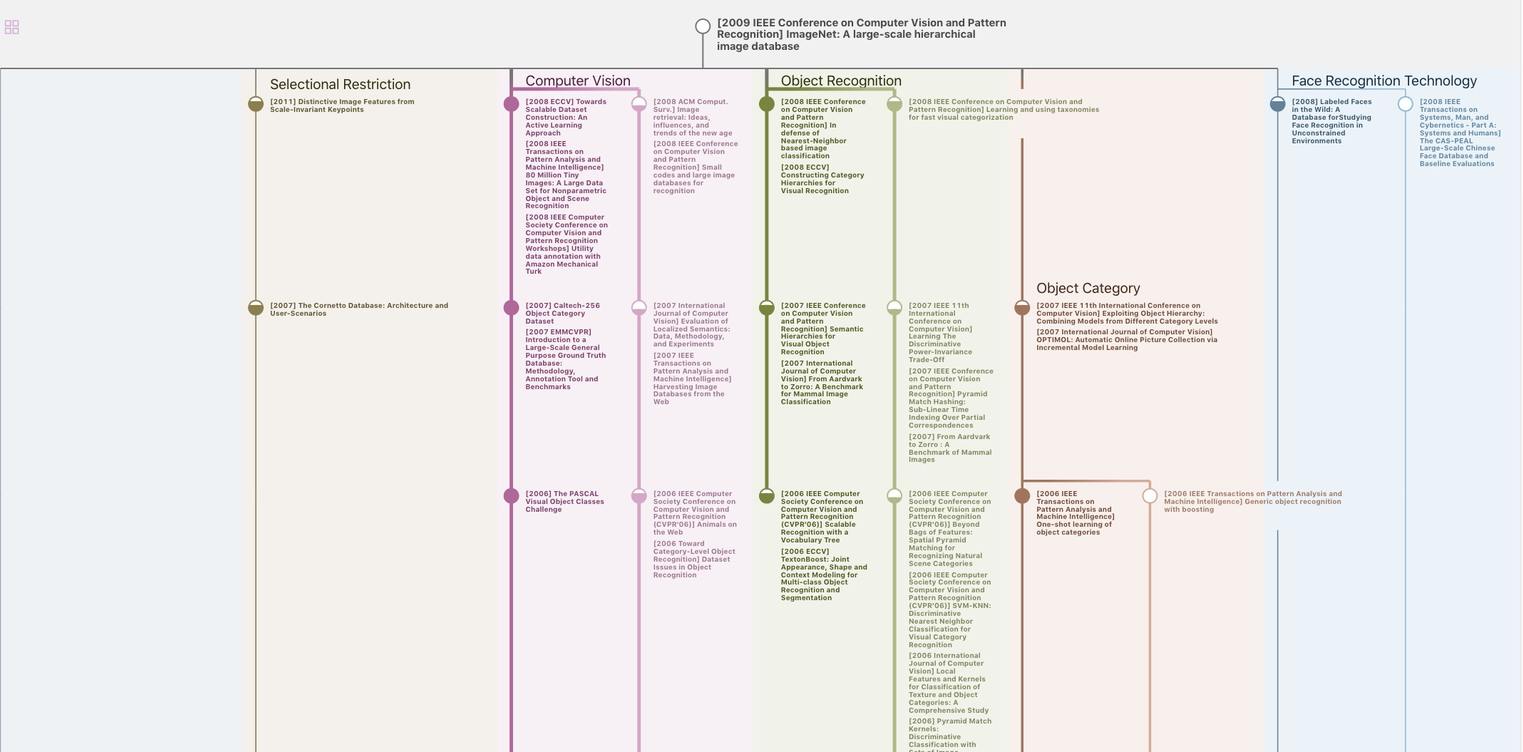
Generate MRT to find the research sequence of this paper
Chat Paper
Summary is being generated by the instructions you defined