TFPred: Learning discriminative representations from unlabeled data forfew-label rotating machinery fault diagnosis
CONTROL ENGINEERING PRACTICE(2024)
摘要
Recent advances in intelligent rotating machinery fault diagnosis have been enabled by the availability ofmassive labeled training data. However, in practical industrial applications, it is often challenging and costly toannotate a large amount of data. To address the few-label fault diagnosis problem, a time-frequency prediction(TFPred) self-supervised learning framework is proposed to extract latent fault representations from unlabeledfault data. Specifically, the TFPred framework consists of a time encoder and a frequency encoder, with thefrequency encoder to predict the low-dimensional representations of time domain signals generated by the timeencoder with randomly augmented data. Subsequently, the pre-trained network is hooked with a classificationhead and fine-tuned with limited labeled data. Finally, the proposed framework is evaluated on a run-to-failurebearing dataset and a hardware-in-the-loop high-speed train simulation platform. The experiments demonstratethat the self-supervised learning framework TFPred achieved competitive performance with only 1% and 5%labeled data. Code is available at https://github.com/Xiaohan-Chen/TFPred.
更多查看译文
关键词
Fault diagnosis,Self-supervised learning,Contrastive learning,Weakly label,Few-labeled data
AI 理解论文
溯源树
样例
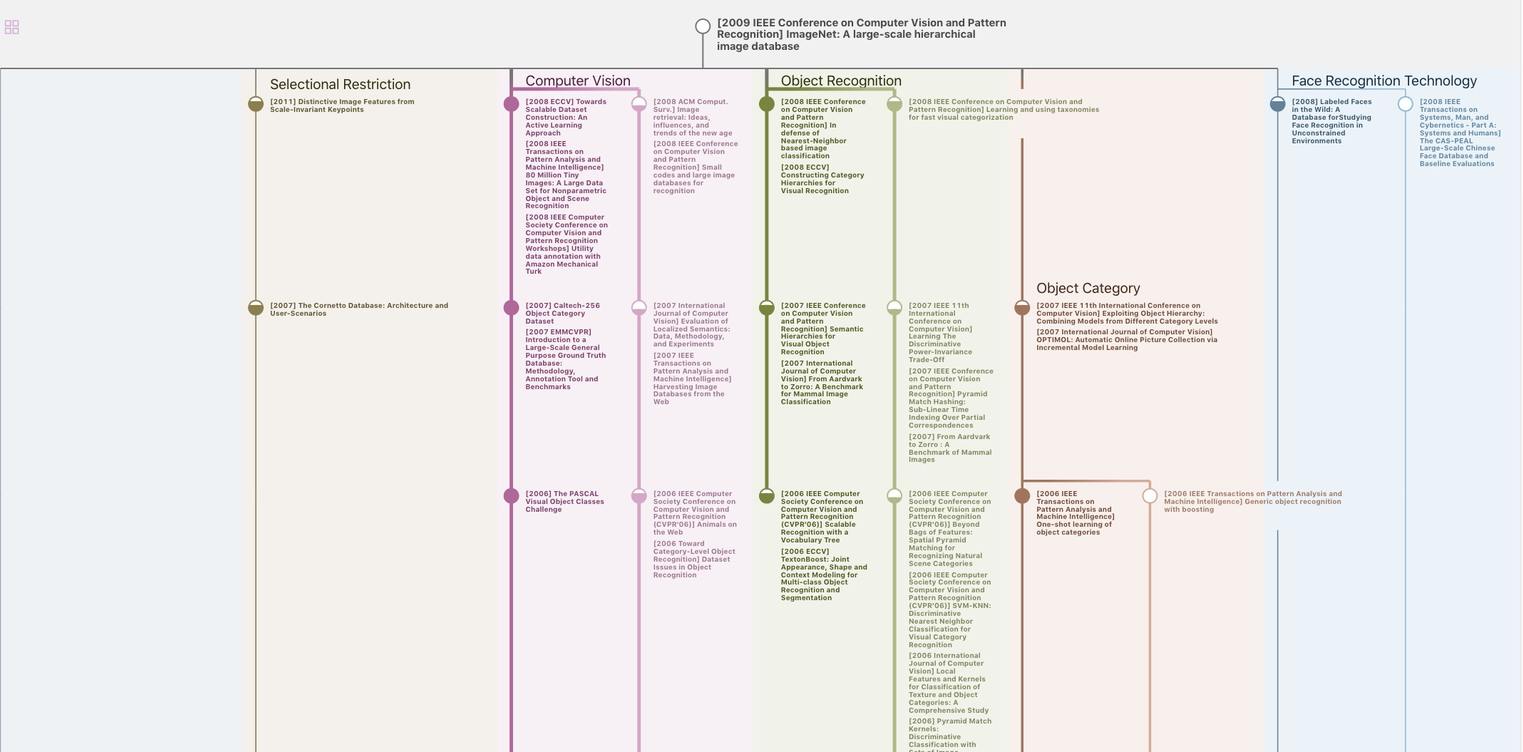
生成溯源树,研究论文发展脉络
Chat Paper
正在生成论文摘要