CrossPrune: Cooperative pruning for camera-LiDAR fused perception models of autonomous driving
KNOWLEDGE-BASED SYSTEMS(2024)
Abstract
Deep neural network pruning is effective in enabling high-performance perception models to be deployed on autonomous driving platforms with limited computation and memory resources. With their rapid development, state-of-the-art autonomous driving perception (ADP) models have advocated the use of multimodal sensors to extract diverse feature categories. However, existing pruning studies in the ADP area focus on singlemodal models and neglect multimodal models. Compared with conventional pruning, multimodal pruning presents a new type of redundancy, namely modal -wise redundancy that is caused by multimodal branches extracting similar perception information. When a specific type of information is extracted by more than one modal branch, although this extraction is deemed essential from an individual single -modal standpoint, it becomes redundant when viewed from a modal -wise perspective. Therefore, modal branches must be handled cooperatively to eliminate modal -wise redundancy while concurrently preserving the original perception accuracy. Building on this, we propose CrossPrune, a modal cooperative pruning framework designed for camera-LiDAR fused perception in autonomous driving. The primary objective of CrossPrune is to effectively eliminate redundancy and achieve nondestructive pruning for multimodal ADP models. This was accomplished by approaching the problem as a multi -objective optimization task, encompassing both weight pruning and the restriction of feature distortions caused by pruning. Experiments conducted on the nuScenes and KITTI datasets demonstrated that CrossPrune attained superior pruning ratios while minimizing accuracy loss, surpassing the performance of the baselines. The key results indicated that the proposed CrossPrune achieved relative improvements of 9.6% in mAP and 11.5% in NDS under 89.8% pruning sparsity.
MoreTranslated text
Key words
Camera-LiDAR fusion,DNN pruning,Perception of autonomous driving
AI Read Science
Must-Reading Tree
Example
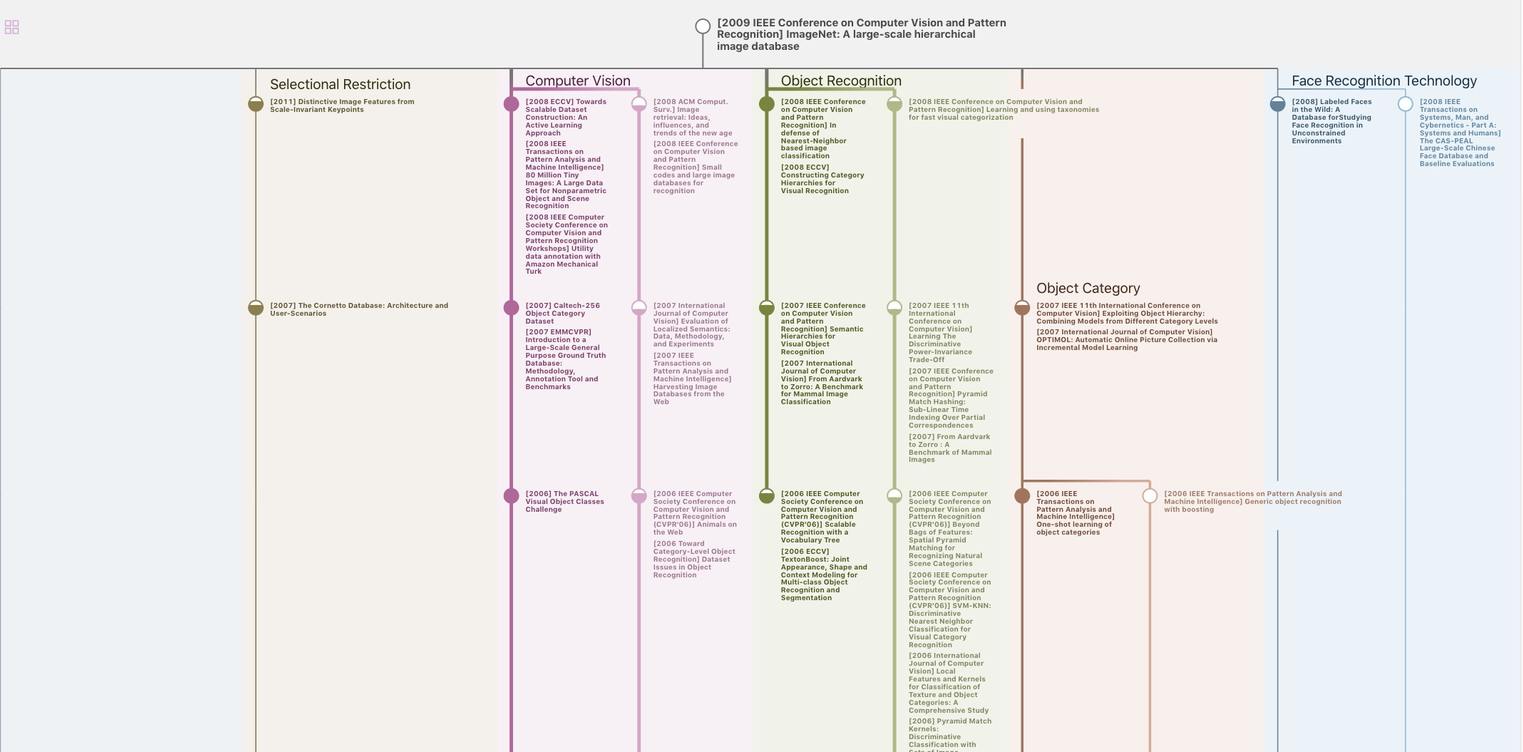
Generate MRT to find the research sequence of this paper
Chat Paper
Summary is being generated by the instructions you defined