A Video Captioning Method by Semantic Topic-Guided Generation
CMC-COMPUTERS MATERIALS & CONTINUA(2024)
Abstract
In the video captioning methods based on an encoder -decoder, limited visual features are extracted by an encoder, and a natural sentence of the video content is generated using a decoder. However, this kind of method is dependent on a single video input source and few visual labels, and there is a problem with semantic alignment between video contents and generated natural sentences, which are not suitable for accurately comprehending and describing the video contents. To address this issue, this paper proposes a video captioning method by semantic topicguided generation. First, a 3D convolutional neural network is utilized to extract the spatiotemporal features of videos during the encoding. Then, the semantic topics of video data are extracted using the visual labels retrieved from similar video data. In the decoding, a decoder is constructed by combining a novel EnhanceTopK sampling algorithm with a Generative Pre -trained Transformer -2 deep neural network, which decreases the influence of "deviation" in the semantic mapping process between videos and texts by jointly decoding a baseline and semantic topics of video contents. During this process, the designed Enhance-TopK sampling algorithm can alleviate a long -tail problem by dynamically adjusting the probability distribution of the predicted words. Finally, the experiments are conducted on two publicly used Microsoft Research Video Description and Microsoft Research -Video to Text datasets. The experimental results demonstrate that the proposed method outperforms several state -of -art approaches. Specifically, the performance indicators Bilingual Evaluation Understudy, Metric for Evaluation of Translation with Explicit Ordering, Recall Oriented Understudy for Gisting Evaluation -longest common subsequence, and Consensus -based Image Description Evaluation of the proposed method are improved by 1.2%, 0.1%, 0.3%, and 2.4% on the Microsoft Research Video Description dataset, and 0.1%, 1.0%, 0.1%, and 2.8% on the Microsoft Research -Video to Text dataset, respectively, compared with the existing video captioning methods. As a result, the proposed method can generate video captioning that is more closely aligned with human natural language expression habits.
MoreTranslated text
Key words
Video captioning,encoder-decoder,semantic topic,jointly decoding,Enhance-TopK sampling
AI Read Science
Must-Reading Tree
Example
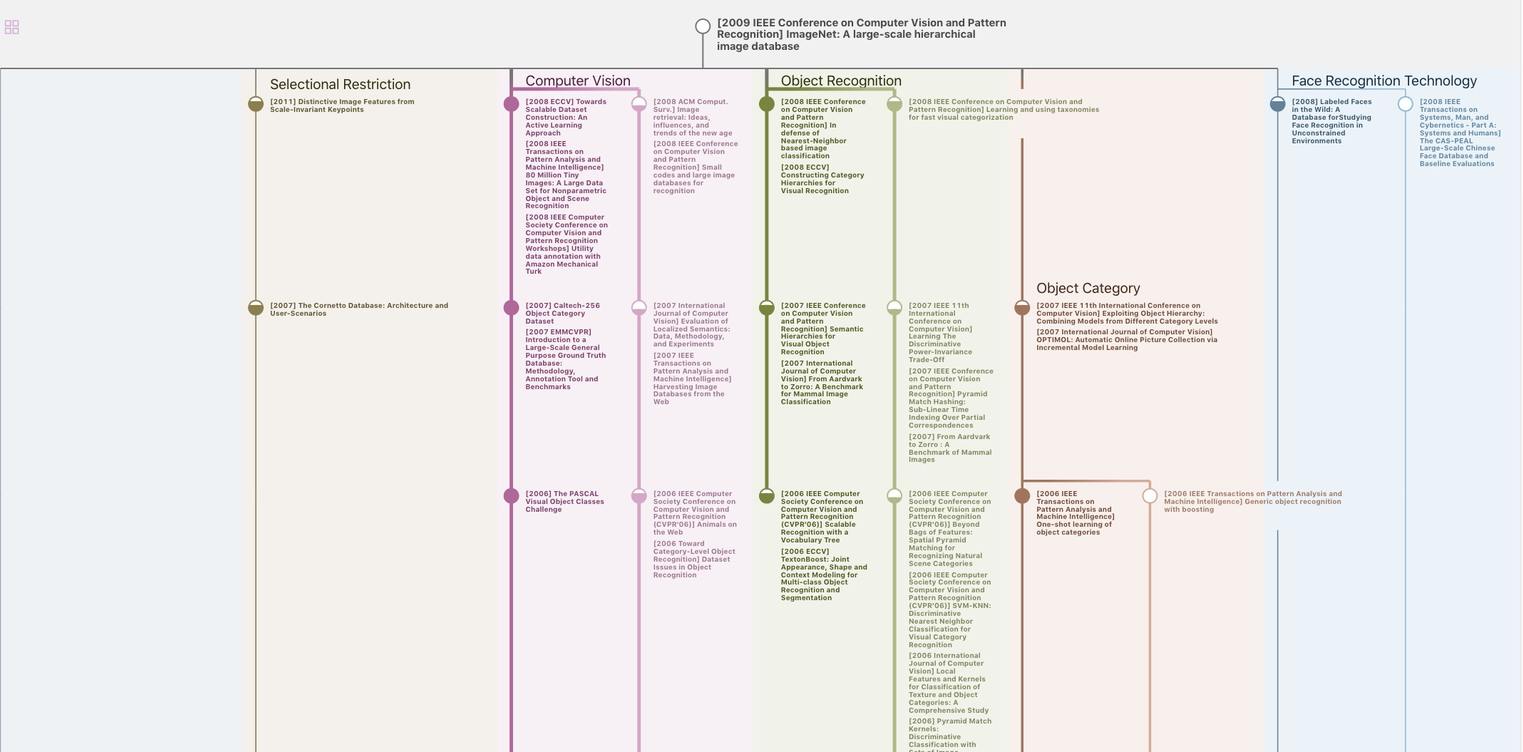
Generate MRT to find the research sequence of this paper
Chat Paper
Summary is being generated by the instructions you defined