Towards a hygroscopic growth calibration for low-cost PM2.5 sensors
ATMOSPHERIC MEASUREMENT TECHNIQUES(2024)
摘要
Low-cost particulate matter (PM) sensors continue to grow in popularity, but issues such as aerosol-size-dependent sensitivity drive the need for effective calibration schemes. Here we devise a time-evolving calibration method for the Plantower PMS5003 PM2.5 mass concentration measurements. We use 2 years of measurements from the Berkeley Environmental Air-quality and CO2 Network sensors deployed in San Francisco and Los Angeles in our analysis. The calibration uses a hygroscopic growth correction factor derived from kappa-K & ouml;hler theory, where the calibration parameters are determined empirically using US Environmental Protection Agency Air Quality System (EPA AQS) reference data at co-location sites during the period from 2021-2022. The parameters are found to vary cyclically through the seasons, and the seasonal cycles match changes in sulfate and elemental carbon PM composition fractions throughout the year. In both regions, the seasonal RH dependence calibration performs better than the uncalibrated data and data calibrated with the EPA's national Plantower calibration algorithm. In the San Francisco Bay Area, the seasonal RH dependence calibration reduces the root mean square error (RMSE) by similar to 40 % from the uncalibrated data and maintains a mean bias much smaller than the EPA national calibration scheme (-0.90 vs -2.73 mu g m(-3)). We also find that calibration parameters forecasted beyond those fit with the EPA reference data continue to outperform the uncalibrated data and EPA calibration data, enabling real-time application of the calibration scheme even in the absence of reference data. While the correction greatly improves the data accuracy, non-Gaussian distribution of the residuals suggests that other processes besides hygroscopic growth can be parameterized for future improvement of this calibration.
更多查看译文
AI 理解论文
溯源树
样例
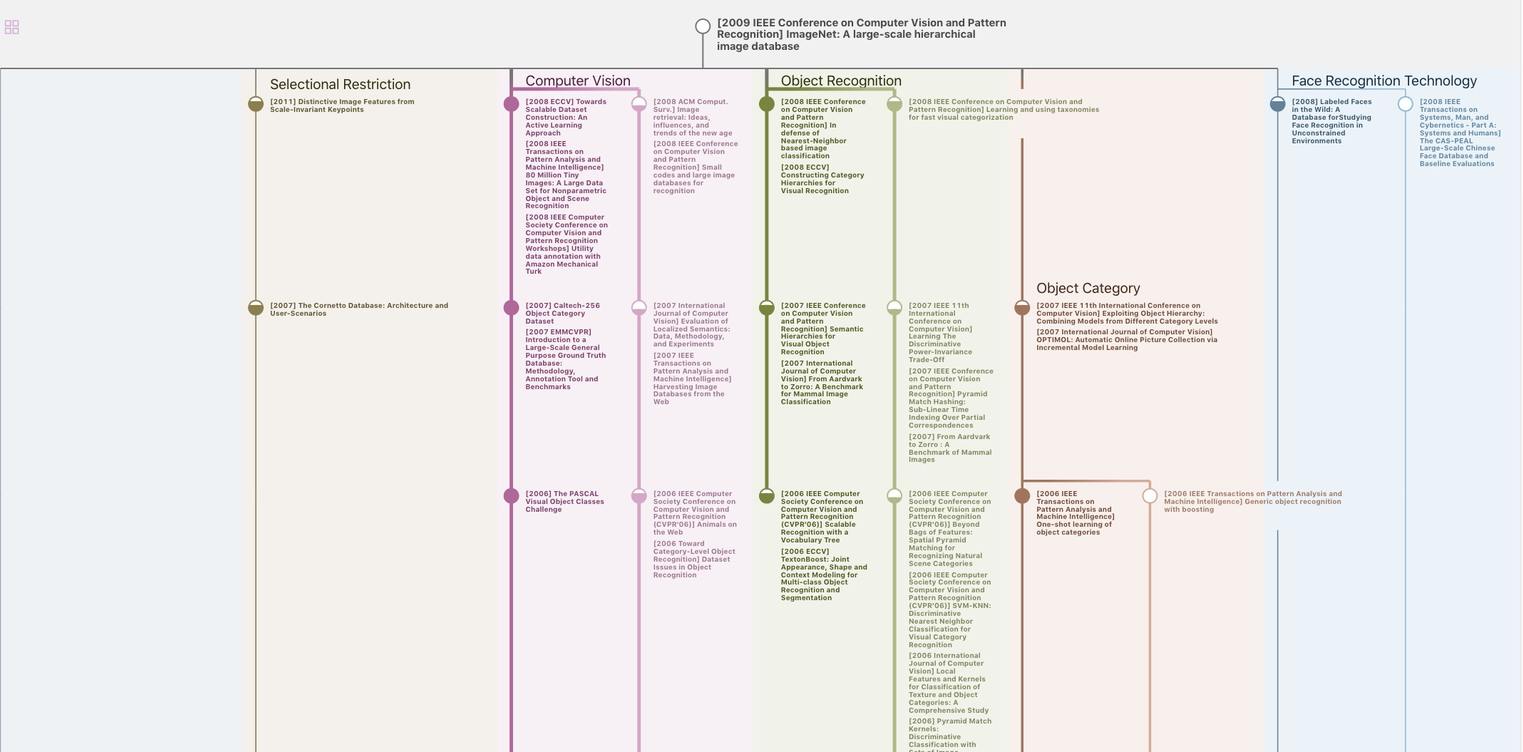
生成溯源树,研究论文发展脉络
Chat Paper
正在生成论文摘要