Prediction of compressive strength of sustainable concrete using machine learning tools
COMPUTERS AND CONCRETE(2024)
摘要
The technique of experimentally determining concrete's compressive strength for a given mix design is timeconsuming and difficult. The goal of the current work is to propose a best working predictive model based on different machine learning algorithms such as Gradient Boosting Machine (GBM), Stacked Ensemble (SE), Distributed Random Forest (DRF), Extremely Randomized Trees (XRT), Generalized Linear Model (GLM), and Deep Learning (DL) that can forecast the compressive strength of ternary geopolymer concrete mix without carrying out any experimental procedure. A geopolymer mix uses supplementary cementitious materials obtained as industrial by-products instead of cement. The input variables used for assessing the best machine learning algorithm not only include individual ingredient quantities, but molarity of the alkali activator and age of testing as well. Myriad statistical parameters used to measure the effectiveness of the models in forecasting the compressive strength of ternary geopolymer concrete mix, it has been found that GBM performs better than all other algorithms. A sensitivity analysis carried out towards the end of the study suggests that GBM model predicts results close to the experimental conditions with an accuracy between 95.6 % to 98.2 % for testing and training datasets.
更多查看译文
关键词
compressive strength prediction,GBM,machine learning,sensitivity analysis,ternary geopolymer concrete
AI 理解论文
溯源树
样例
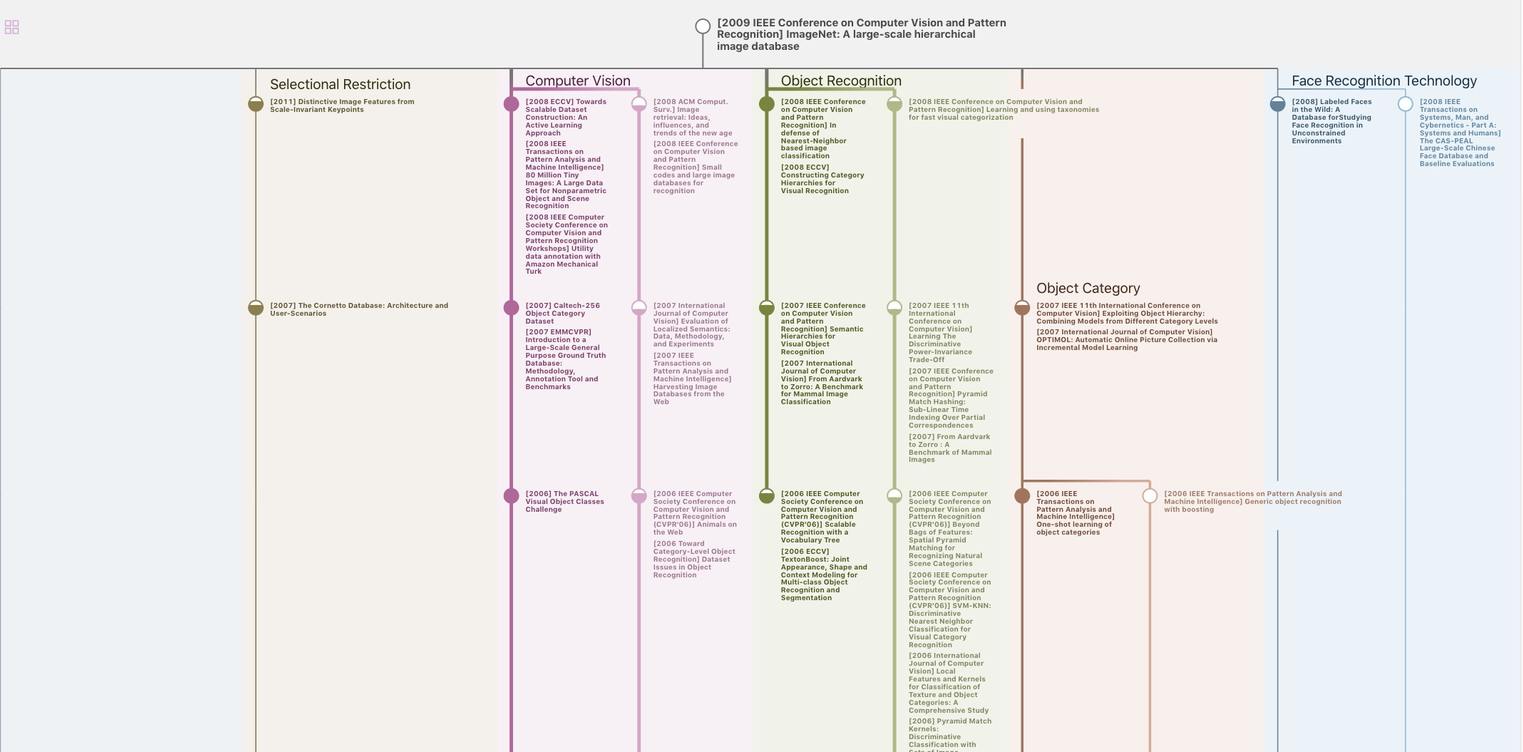
生成溯源树,研究论文发展脉络
Chat Paper
正在生成论文摘要