Graph Contrastive Learning with Knowledge Transfer for Recommendation
ENGINEERING LETTERS(2024)
Abstract
The graph collaborative filtering algorithm, known for its excellent data modeling capability, has found wide applications in recommendation systems. However, traditional graph collaborative filtering algorithms often face challenges related to sparse interaction data and inefficient utilization of side information between users and items. To address these issues, a graph Contrastive Learning with Knowledge Transfer (CLKT) has been proposed. The proposed method aims to integrate the side information of user and item attributes with interaction data using GNN. By leveraging contrastive learning, it achieves cross -view learning within the graph collaborative filtering algorithm. Recognizing that the impact of side information on user -item interaction data can vary, the proposed method introduces feature transformers and knowledge transfer networks into contrastive learning. This enables the adaptive embedding of node features for users and items in CLKT. To further enhance the embedding capability for different node relationships, the CLKT method incorporates cluster -based contrastive learning. This approach considers the diverse impacts that different types of node relationships can have on node feature learning. By simultaneously learning both the interaction relationships between nodes and the clustering relationships among nodes, the proposed method enables the exploration of multiple -node relationships for feature mining. Extensive experiments on three real datasets demonstrate the effectiveness and feasibility of the proposed CLKT, and the results demonstrated its promising performance.
MoreTranslated text
Key words
Recommendation Algorithm,Self-Supervised Learning,Graph Neural Network,Contrastive Learning
AI Read Science
Must-Reading Tree
Example
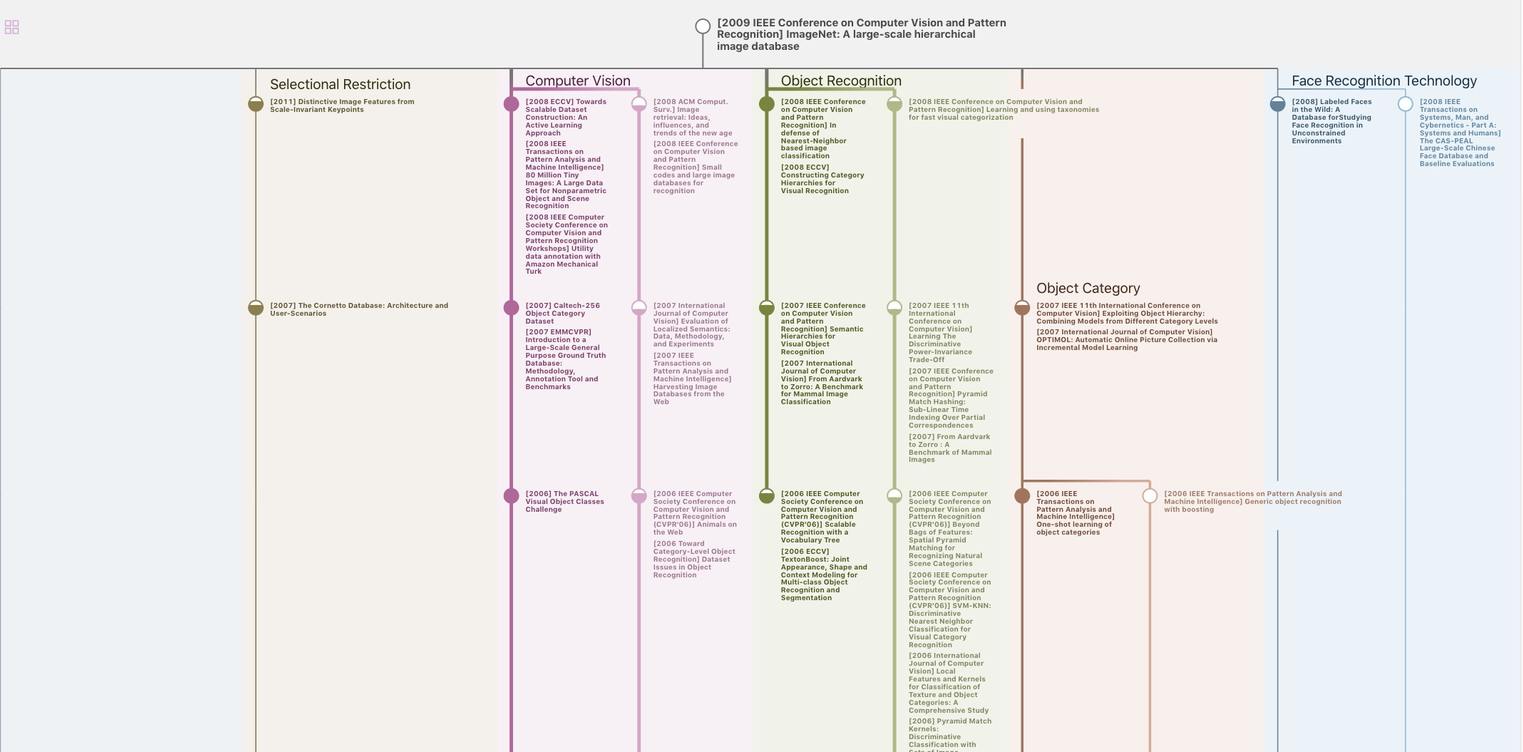
Generate MRT to find the research sequence of this paper
Chat Paper
Summary is being generated by the instructions you defined