Functional Directed Acyclic Graphs
JOURNAL OF MACHINE LEARNING RESEARCH(2024)
Abstract
In this article, we introduce a new method to estimate a directed acyclic graph (DAG) from multivariate functional data. We build on the notion of faithfulness that relates a DAG with a set of conditional independences among the random functions. We develop two linear operators, the conditional covariance operator and the partial correlation operator, to characterize and evaluate the conditional independence. Based on these operators, we adapt and extend the PC-algorithm to estimate the functional directed graph, so that the computation time depends on the sparsity rather than the full size of the graph. We study the asymptotic properties of the two operators, derive their uniform convergence rates, and establish the uniform consistency of the estimated graph, all of which are obtained while allowing the graph size to diverge to infinity with the sample size. We demonstrate the efficacy of our method through both simulations and an application to a time-course proteomic dataset.
MoreTranslated text
Key words
Graphical model,faithfulness,functional regression,linear operator,repro ducing kernel Hilbert space,uniform consistency
AI Read Science
Must-Reading Tree
Example
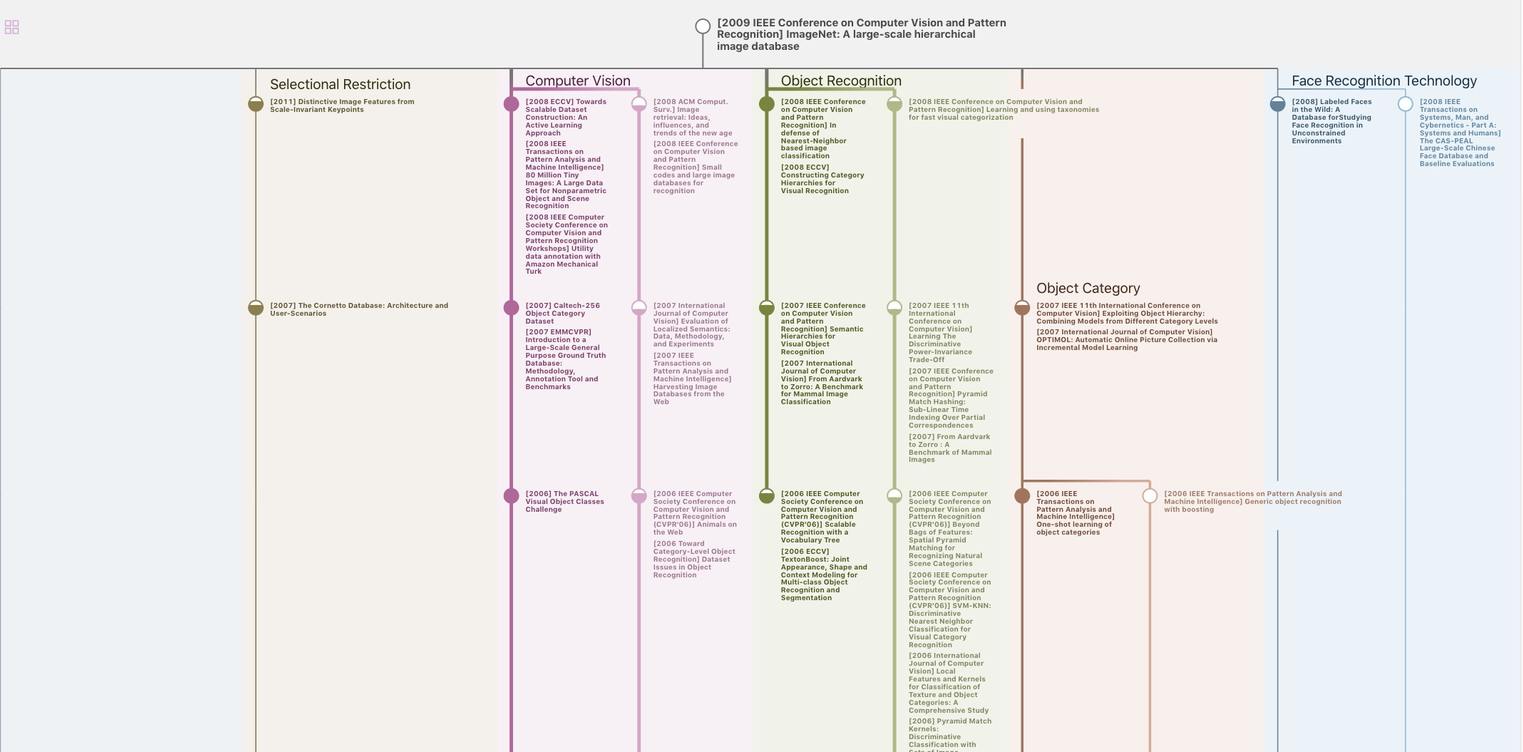
Generate MRT to find the research sequence of this paper
Chat Paper
Summary is being generated by the instructions you defined