Underground Railway Station Passenger Flow Prediction Based on Long Short-Term Memory Neural Network
COMPUTING IN CIVIL ENGINEERING 2023-DATA, SENSING, AND ANALYTICS(2024)
摘要
With the rapid expansion of underground railway systems and the increase in public transportation ridership, short-term metro passenger flow forecasting has become one of the prevalent topics in transportation planning, infrastructure construction and improvement, and underground railway monitoring and management. This paper proposes a short-term metro passenger prediction approach based on long short-term memory neural network (LSTM NN). The big data of historical metro passenger flow, the holiday information, and the characteristic of stations are taken into consideration. The prediction performance is evaluated by mean absolute error (MAE) and root mean squared error (RMSE). Experiments are conducted based on Hong Kong Mass Transit Railway (MTR) data, and the result indicates that the proposed model has a higher accuracy than baseline models. The novel model provides an option for stakeholders to achieve a high-performance prediction of metro passenger flow. By obtaining future passenger flow data in advance, the authorities can make appropriate decisions on real-time underground railway system management and deliver timely responses to unexpected events.
更多查看译文
AI 理解论文
溯源树
样例
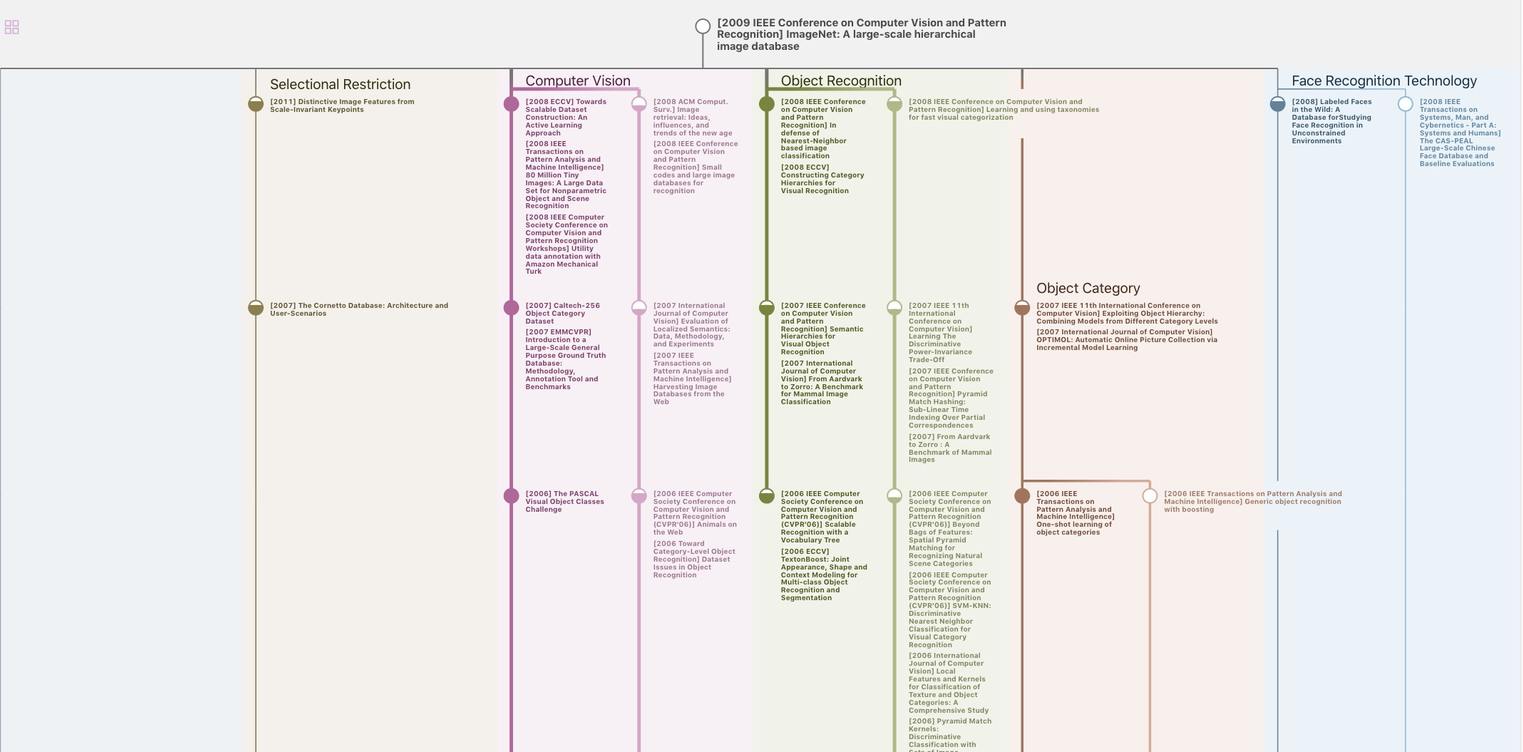
生成溯源树,研究论文发展脉络
Chat Paper
正在生成论文摘要