Automatic Detection of Collapsed Buildings after the 6 February 2023 Trkiye Earthquakes Using Post-Disaster Satellite Images with Deep Learning-Based Semantic Segmentation Models
BUILDINGS(2024)
摘要
This study focuses on the identification of collapsed buildings in satellite images after earthquakes through deep learning-based image segmentation models. The performance of four different architectures, namely U-Net, LinkNet, FPN, and PSPNet, was evaluated using various performance metrics, such as accuracy, precision, recall, F1 score, specificity, AUC, and IoU. The study used satellite images taken from the area located in the south and southeast of Turkiye covering the eleven provinces which are most affected by the Mw 7.7 Pazarcik (Kahramanmaras) and Mw 7.6 Elbistan (Kahramanmaras) earthquakes. The results indicated that FPN and U-Net were the best-performing models depending on the performance metric of interest. FPN achieved the highest accuracy and specificity scores, as well as the best precision score, while U-Net achieved the best recall and F1 score values, as well as the best AUC and IoU scores. The training and validation accuracy and loss curves were analyzed, and the results indicated that all four models achieved an accuracy value of over 96%. The FPN model outperformed the others in terms of accurately segmenting images while maintaining a low loss value. This study provides insights into the potential of deep learning-based image segmentation models in disaster management and can be useful for future research in this field.
更多查看译文
关键词
earthquake damage detection,collapsed building identification,deep learning,image segmentation,satellite images
AI 理解论文
溯源树
样例
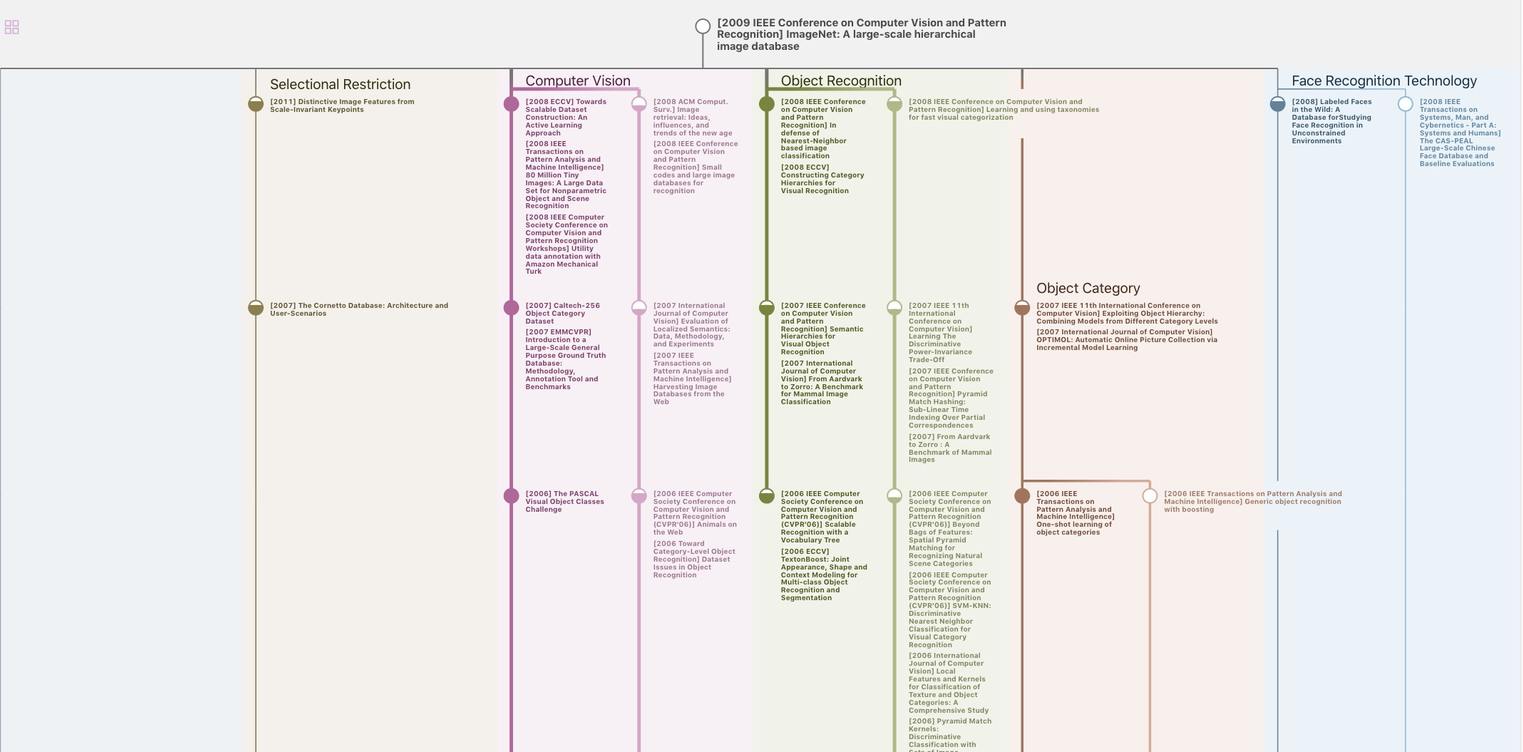
生成溯源树,研究论文发展脉络
Chat Paper
正在生成论文摘要